URLLC resource slicing and scheduling for trustworthy 6G vehicular services: A federated reinforcement learning approach
PHYSICAL COMMUNICATION(2021)
摘要
In the upcoming 6G era, vehicles will massively connect to the wireless network through edge access points such as roadside units (RSUs). The increasing number of connected vehicles and vehicular services will take 6G vehicular network to a new challenging security boundary, i.e., the so-called zero trust network. The traditional resource slicing and scheduling solution has to evolve to deal with the zero trust security problems. In this paper, we consider the trustworthy 6G vehicular services and focus on the typical scenario of vehicular task offloading with resource slicing and scheduling. In order to prevent vehicles from malicious attacks by untrusted edge access points, we exploit a subjective logic model to score the reputation of edge nodes. The vehicles will select the edge nodes with high reputation for task offloading. After that, we develop an federated asynchronous reinforcement learning algorithm to optimize the offloading problem. The simulation results show that our approach can efficiently schedule the slice resources and effectively protect the information security of vehicles. (C) 2021 Elsevier B.V. All rights reserved.
更多查看译文
关键词
6G, Vehicular edge computing, Resource slicing, Federated learning, Zero trust architecture
AI 理解论文
溯源树
样例
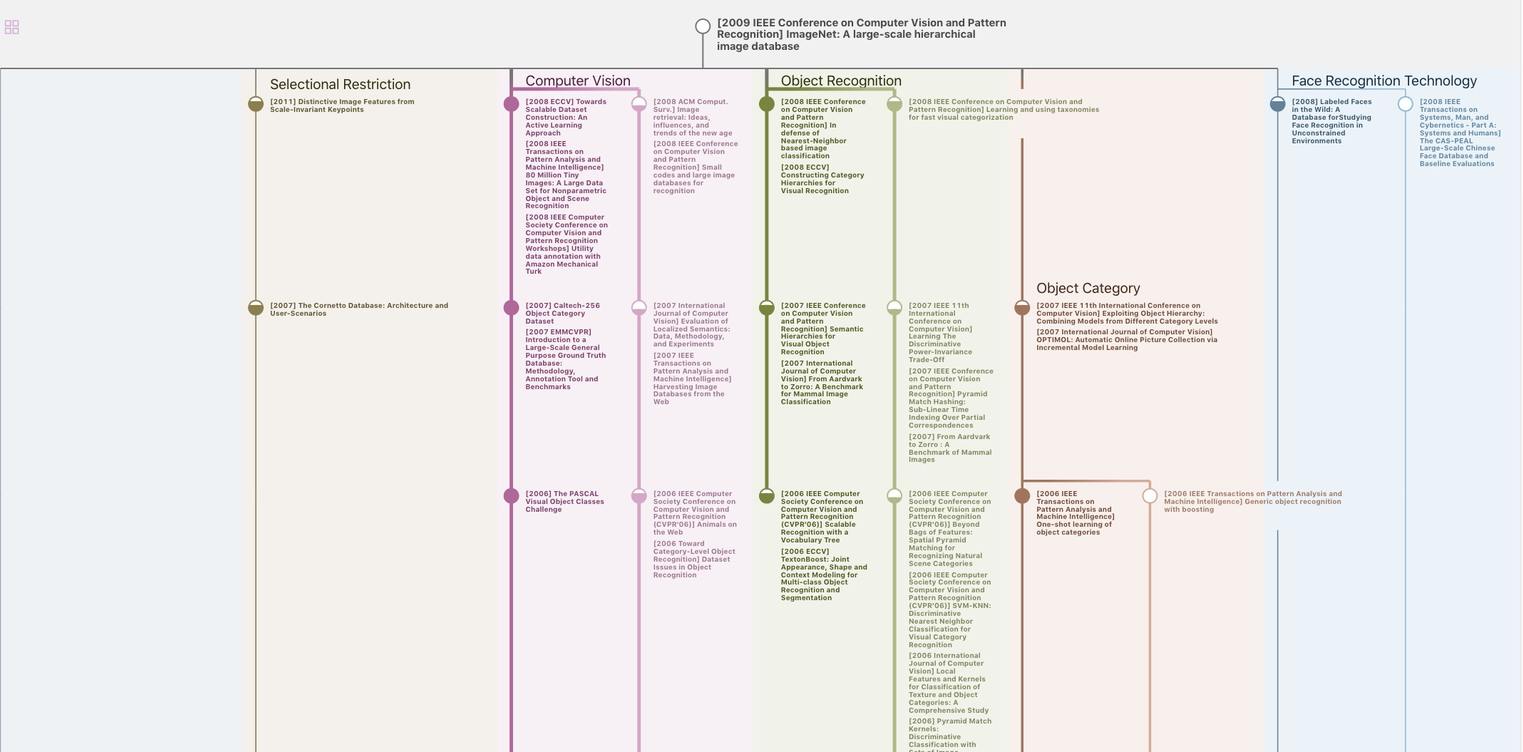
生成溯源树,研究论文发展脉络
Chat Paper
正在生成论文摘要