Hyperspectral Image Denoising via Low-Rank Representation and CNN Denoiser
IEEE Journal of Selected Topics in Applied Earth Observations and Remote Sensing(2022)
Abstract
Hyperspectral images (HSIs) are widely used in various tasks such as earth observation and target detection. However, during the imaging process, HSIs are often corrupted by various noises. In this article, we firstly investigate the advantages of traditional physical restoration models and the denoising convolutional neural networks (CNN) for HSIs denoising tasks. The sparse based low-rank representation can explore the global correlations in both the spatial and spectral domains, and the CNN-based denoiser can represent the deep prior which cannot be designed by traditional restoration models. Then, we propose a HSI denoising model with low-rank representation and CNN denoiser prior in the flexible and extensible plug-and-play framework by combining the advantages of the two methods. The proposed model is user-friendly, requiring no retraining. Simulated data experiments show that, compared with competitive methods, the proposed one achieves better denoising results for both additive Gaussian noise and Poissonian noise in various quantitative evaluation indicators. Real data experiments show that the proposed model yields the best performance.
MoreTranslated text
Key words
Convolutional neural network (CNN),hyperspectral image (HSI) denoising,low-rank representation
AI Read Science
Must-Reading Tree
Example
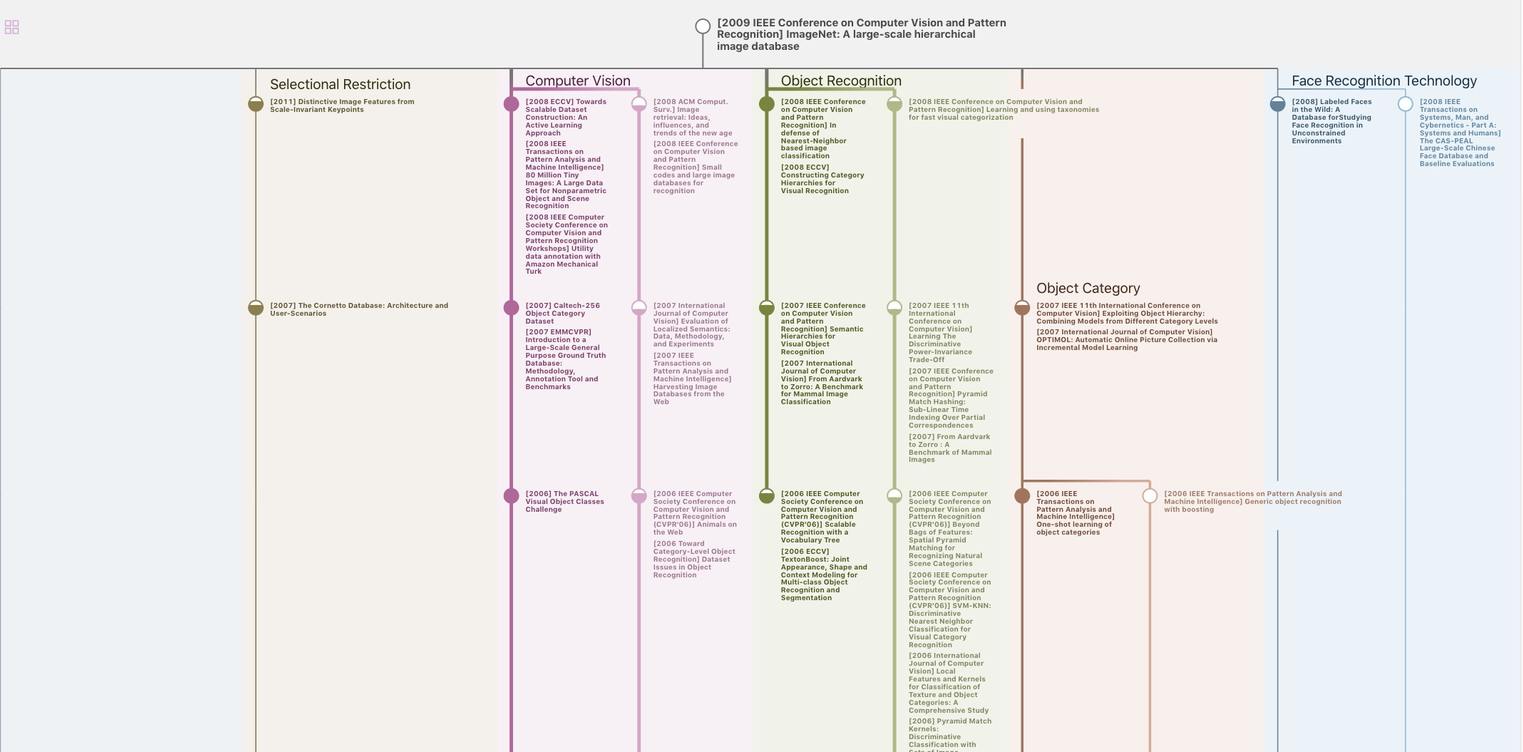
Generate MRT to find the research sequence of this paper
Chat Paper
Summary is being generated by the instructions you defined