A novel fidelity selection strategy-guided multifidelity kriging algorithm for structural reliability analysis
Reliability Engineering & System Safety(2022)
摘要
Multifidelity (MF) surrogate models have recently attracted intensive attention due to their advantage of reducing computational demand by fusing multiple sources of data. However, their applications in reliability analyses with active learning processes are scarce because of lack of effective strategies for determining locations and fidelities of updated samples. To circumvent this bottleneck, a new active learning-based MF kriging method for reliability analysis via RU learning function and “BSC-Believer” fidelity selection strategy is proposed. The proposed approach employs a two-phase operation to determine location and fidelity of the updated sample in each iteration. In the first stage, the location of the updated sample is ascertained via learning function RU, which has excellent performance regarding the tradeoff between exploration and exploitation. Then, the “BSC-Believer” strategy is introduced by combining the estimated relative error of the failure probability, the kriging believer concept, and a cost function to select the optimal fidelity for updating. Through the “BSC-Believer” strategy, the appropriate fidelity for reducing failure probability prediction uncertainty within a unit of computational demand is identified. It is demonstrated through five examples that the proposed algorithm is superior to the recently reported approaches in reducing the computational burden of maintaining the desired estimation accuracy.
更多查看译文
关键词
Reliability analysis,Multifidelity kriging model,Fidelity selection,Active learning process,Uncertainty quantification
AI 理解论文
溯源树
样例
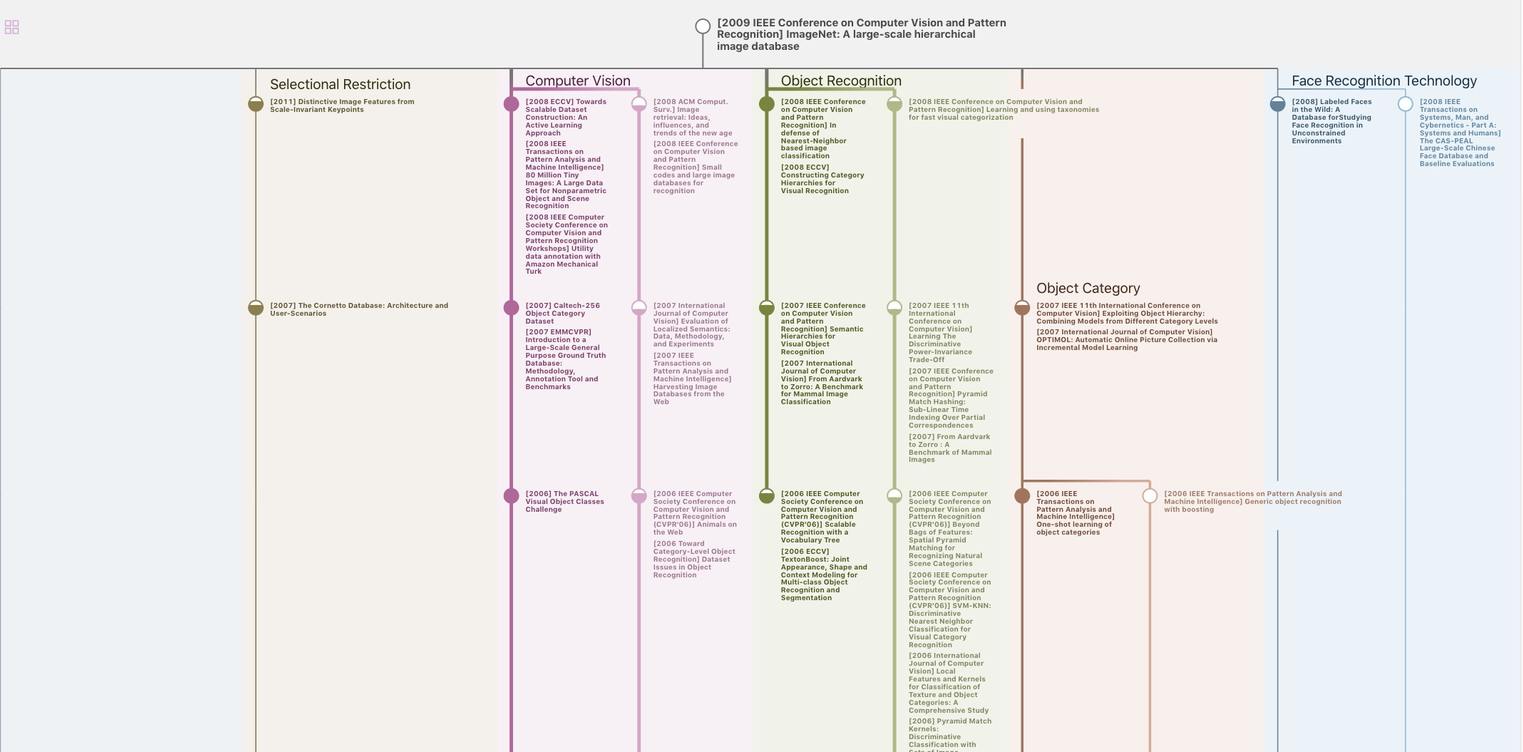
生成溯源树,研究论文发展脉络
Chat Paper
正在生成论文摘要