Tool wear prediction using convolutional bidirectional LSTM networks
The Journal of Supercomputing(2021)
摘要
Machine health monitoring systems are vital components of modern manufacturing industries. As advanced sensors collecting machine health-related data become commonplace, such systems have started adopting data-driven approaches to harness the collected data. However, dealing with noisy data and gleaning the spatial and temporal correlation within the data is a challenge. Extant literature focuses on the use of feature extraction to judge the state of normal and worn tools. However, the process of detecting the features of worn tools can often damage the workpiece material and result in machine downtime, which increases costs. Using recent developments in artificial intelligence, data features can be used to gauge the condition of tools in real-time. This paper proposes Holistic–Local Long Short-Term Memory (HLLSTM), a deep learning approach that adopts Long Short-Term Memory to predict tool wear based on holistic and local features. The data is divided into segments to learn short-term data features and further divided into holistic training and local training data to extract more implicit feature information to improve the accuracy of tool wear prediction. Experimental results indicate that HLLSTM can reduce the mean absolute error of real tool wear value two-fold and accurately predict tool wear.
更多查看译文
关键词
Machine health monitoring, Tool wear prediction, Recurrent neural network, Long short-term memory
AI 理解论文
溯源树
样例
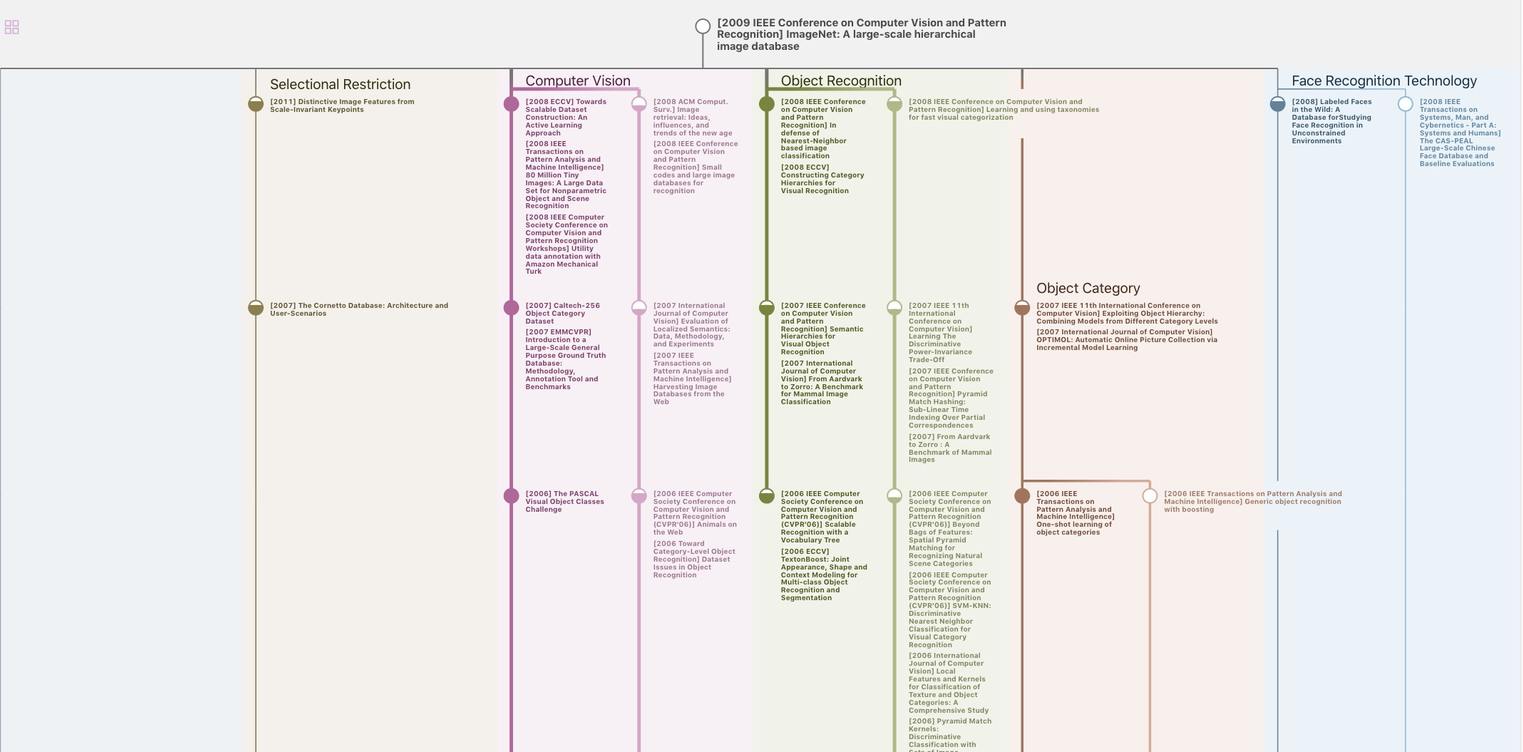
生成溯源树,研究论文发展脉络
Chat Paper
正在生成论文摘要