Extrapolative Bayesian Optimization with Gaussian Process and Neural Network Ensemble Surrogate Models
ADVANCED INTELLIGENT SYSTEMS(2021)
摘要
Bayesian optimization (BO) has emerged as the algorithm of choice for guiding the selection of experimental parameters in automated active learning driven high throughput experiments in materials science and chemistry. Previous studies suggest that optimization performance of the typical surrogate model in the BO algorithm, Gaussian processes (GPs), may be limited due to its inability to handle complex datasets. Herein, various surrogate models for BO, including GPs and neural network ensembles (NNEs), are investigated. Two materials datasets of different complexity with different properties are used, to compare the performance of GP and NNE-the first is the compressive strength of concrete (8 inputs and 1 target), and the second is a simulated high-dimensional dataset of thermoelectric properties of inorganic materials (22 inputs and 1 target). While NNEs can converge faster toward optimum values, GPs with optimized kernels are able to ultimately achieve the best evaluated values after 100 iterations, even for the most complex dataset. This surprising result is contrary to expectations. It is believed that these findings shed new light on the understanding of surrogate models for BO, and can help accelerate the inverse design of new materials with better structural and functional performance.
更多查看译文
关键词
automated experiments, Bayesian optimization, extrapolative algorithms, machine learning, neural network ensembles
AI 理解论文
溯源树
样例
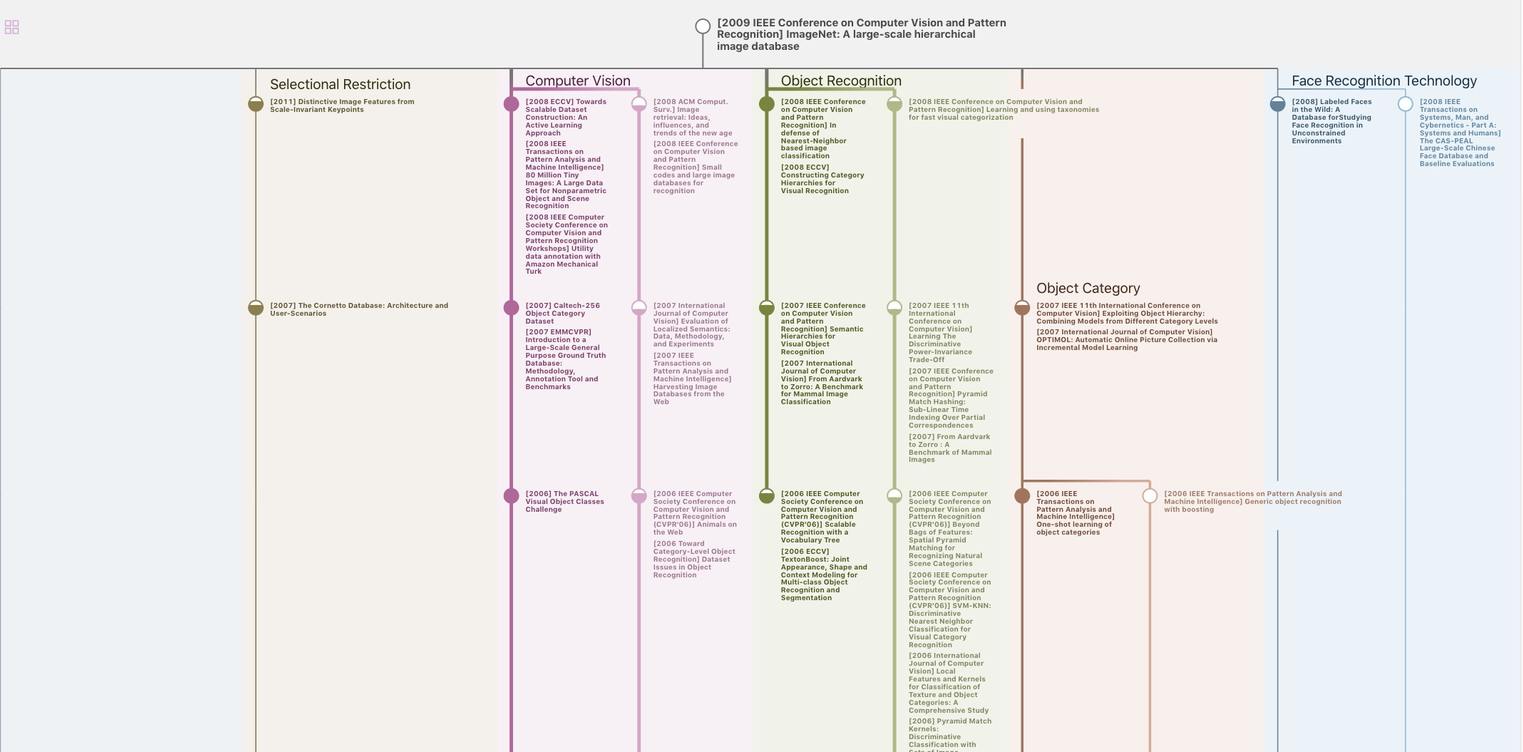
生成溯源树,研究论文发展脉络
Chat Paper
正在生成论文摘要