A Lightweight Detection Model for SAR Aircraft in a Complex Environment
REMOTE SENSING(2021)
摘要
Recently, deep learning has been widely used in synthetic aperture radar (SAR) aircraft detection. However, the complex environment of the airport-consider the boarding bridges, for instance-greatly interferes with aircraft detection. Besides, the detection speed is also an important indicator in practical applications. To alleviate these problems, we propose a lightweight detection model (LDM), mainly including a reuse block (RB) and an information correction block (ICB) based on the Yolov3 framework. The RB module helps the neural network extract rich aircraft features by aggregating multi-layer information. While the RB module brings more effective information, there is also redundant and useless information aggregated by the reuse block, which is harmful to detection precision. Therefore, to accurately extract more aircraft features, we propose an ICB module combining scattering mechanism characteristics by extracting the gray features and enhancing spatial information, which helps suppress interference in a complex environment and redundant information. Finally, we conducted a series of experiments on the SAR aircraft detection dataset (SAR-ADD). The average precision was 0.6954, which is superior to the precision values achieved by other methods. In addition, the average detection time of LDM was only 6.38 ms, making it much faster than other methods.
更多查看译文
关键词
synthetic aperture radar, aircraft detection, scattering information, reuse information, lightweight detection model
AI 理解论文
溯源树
样例
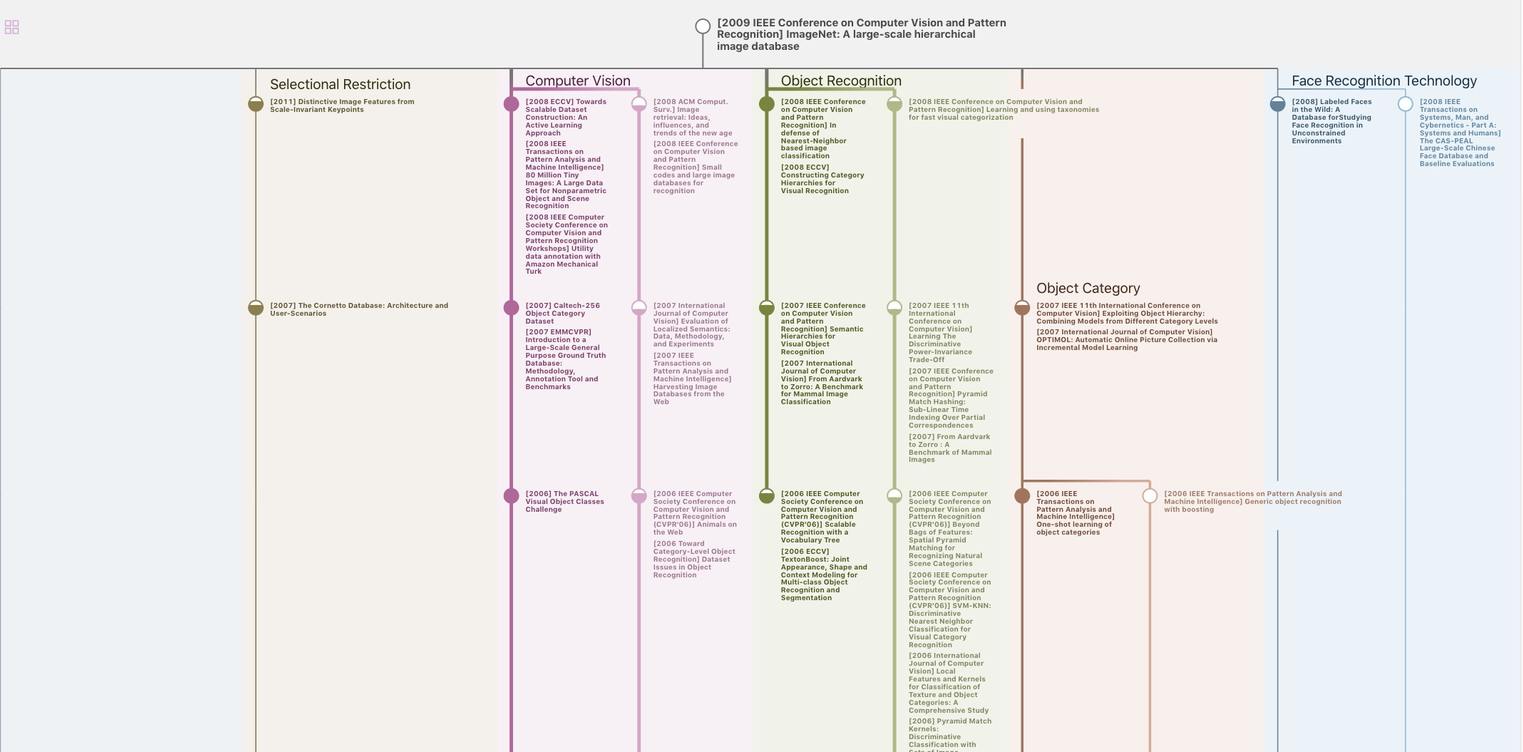
生成溯源树,研究论文发展脉络
Chat Paper
正在生成论文摘要