Change Detection for Heterogeneous Remote Sensing Images with Improved Training of Hierarchical Extreme Learning Machine (HELM)
REMOTE SENSING(2021)
Abstract
To solve the problems of susceptibility to image noise, subjectivity of training sample selection, and inefficiency of state-of-the-art change detection methods with heterogeneous images, this study proposes a post-classification change detection method for heterogeneous images with improved training of hierarchical extreme learning machine (HELM). After smoothing the images to suppress noise, a sample selection method is defined to train the HELM for each image, in which the feature extraction is respectively implemented for heterogeneous images and the parameters need not be fine-tuned. Then, the multi-temporal feature maps extracted from the trained HELM are segmented to obtain classification maps and then compared to generate a change map with changed types. The proposed method is validated experimentally by using one set of synthetic aperture radar (SAR) images obtained from Sentinel-1, one set of optical images acquired from Google Earth, and two sets of heterogeneous SAR and optical images. The results show that compared to state-of-the-art change detection methods, the proposed method can improve the accuracy of change detection by more than 8% in terms of the kappa coefficient and greatly reduce run time regardless of the type of images used. Such enhancement reflects the robustness and superiority of the proposed method.
MoreTranslated text
Key words
change detection, heterogeneous images, hierarchical extreme learning machine (HELM), training samples
AI Read Science
Must-Reading Tree
Example
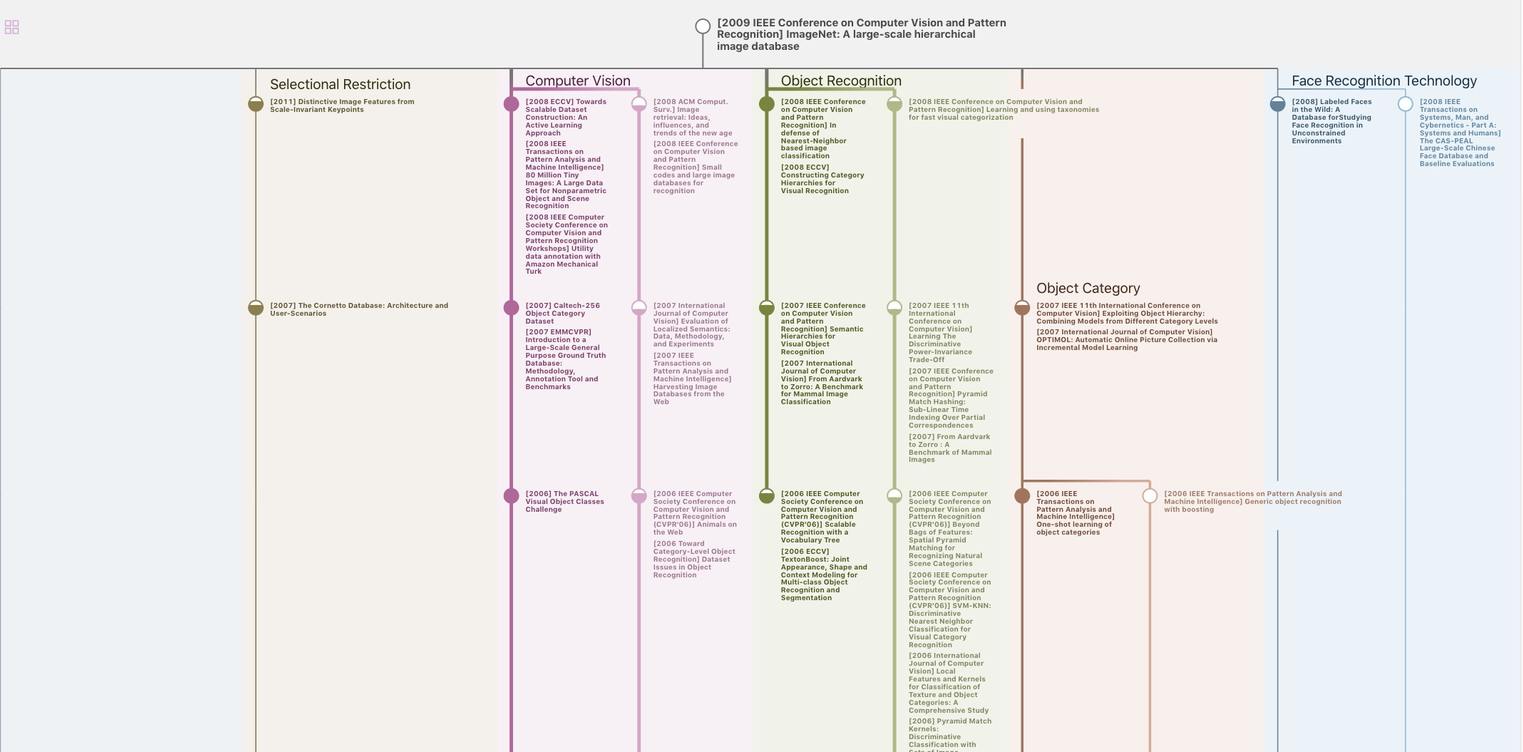
Generate MRT to find the research sequence of this paper
Chat Paper
Summary is being generated by the instructions you defined