Table Pre-training: A Survey on Model Architectures, Pre-training Objectives, and Downstream Tasks.
European Conference on Artificial Intelligence(2022)
摘要
Since a vast number of tables can be easily collected from web pages, spreadsheets, PDFs, and various other document types, a flurry of table pretraining frameworks have been proposed following the success of text and images, and they have achieved new state-of-the-arts on various tasks such as table question answering, table type recognition, column relation classification, table search, formula prediction, etc. To fully use the supervision signals in unlabeled tables, a variety of pretraining objectives have been designed and evaluated, for example, denoising cell values, predicting numerical relationships, and implicitly executing SQLs. And to best leverage the characteristics of (semi-)structured tables, various tabular language models, particularly with specially-designed attention mechanisms, have been explored. Since tables usually appear and interact with free-form text, table pretraining usually takes the form of table-text joint pretraining, which attracts significant research interests from multiple domains. This survey aims to provide a comprehensive review of different model designs, pretraining objectives, and downstream tasks for table pretraining, and we share our thoughts and vision on existing challenges and future opportunities.
更多查看译文
关键词
Survey Track: Knowledge Representation and Reasoning,Survey Track: Natural Language Processing,Survey Track: Data Mining
AI 理解论文
溯源树
样例
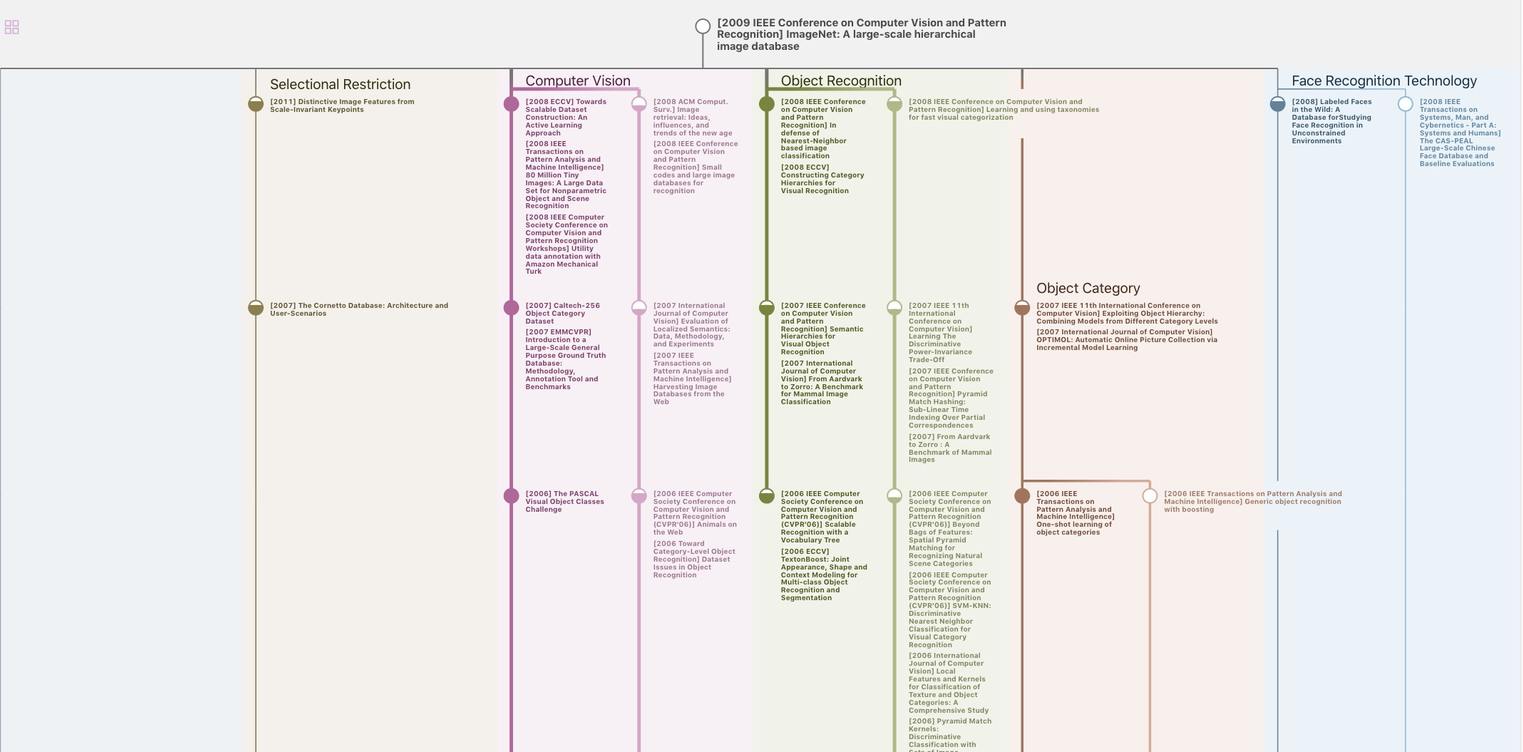
生成溯源树,研究论文发展脉络
Chat Paper
正在生成论文摘要