SEN12MS-CR-TS: A Remote-Sensing Data Set for Multimodal Multitemporal Cloud Removal
IEEE TRANSACTIONS ON GEOSCIENCE AND REMOTE SENSING(2022)
摘要
About half of all optical observations collected via spaceborne satellites are affected by haze or clouds. Consequently, cloud coverage affects the remote-sensing practitioner's capabilities of a continuous and seamless monitoring of our planet. This work addresses the challenge of optical satellite image reconstruction and cloud removal by proposing a novel multimodal and multitemporal data set called SEN12MS-CR-TS. We propose two models highlighting the benefits and use cases of SEN12MS-CR-TS: First, a multimodal multitemporal 3-D convolution neural network that predicts a cloud-free image from a sequence of cloudy optical and radar images. Second, a sequence-to-sequence translation model that predicts a cloud-free time series from a cloud-covered time series. Both approaches are evaluated experimentally, with their respective models trained and tested on SEN12MS-CR-TS. The conducted experiments highlight the contribution of our data set to the remote-sensing community as well as the benefits of multimodal and multitemporal information to reconstruct noisy information. Our data set is available at https://patrickTUM.github.io/cloud_removal.
更多查看译文
关键词
Clouds, Optical imaging, Optical sensors, Satellites, Remote sensing, Image reconstruction, Earth, Cloud removal, data fusion, image reconstruction, sequence-to-sequence, synthetic aperture radar (SAR)-optical, time series
AI 理解论文
溯源树
样例
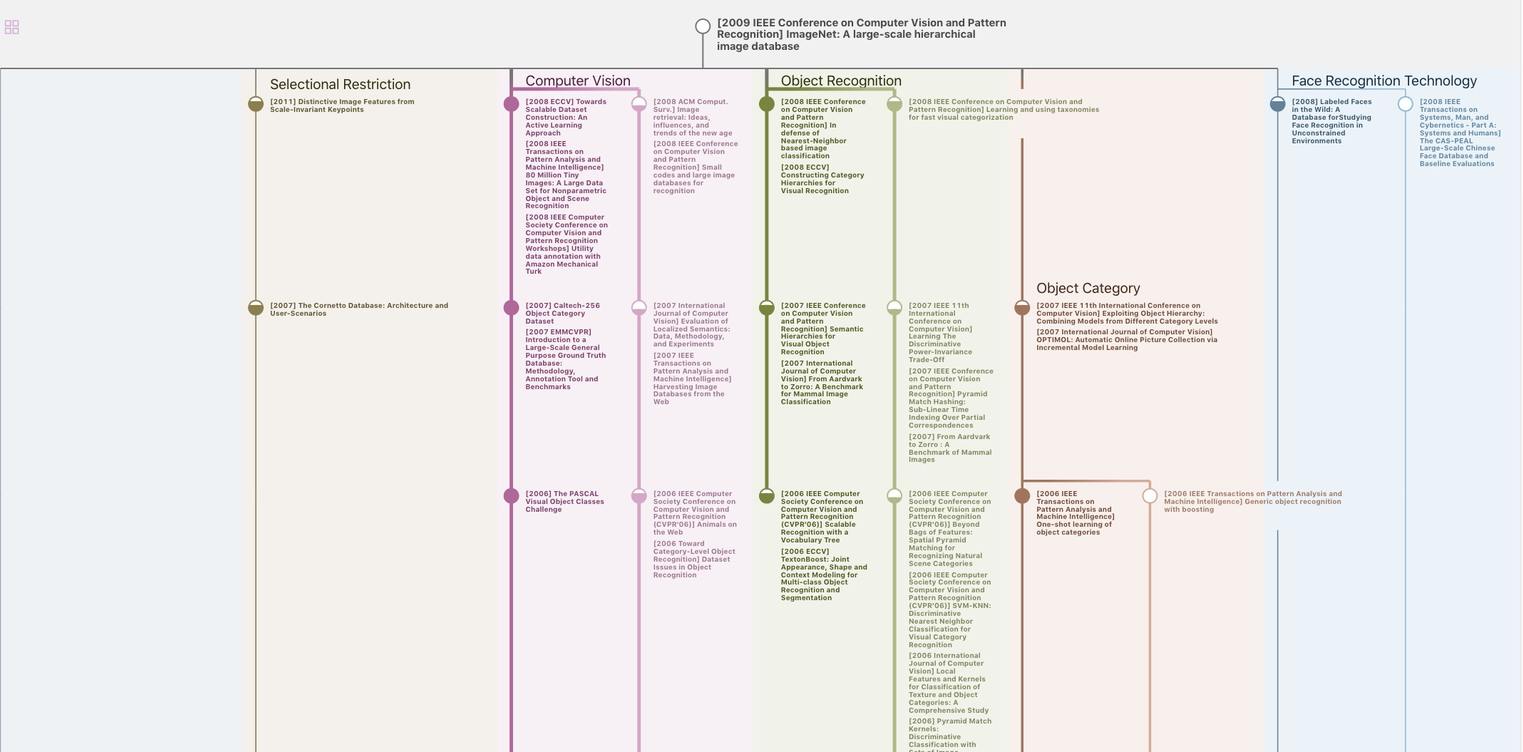
生成溯源树,研究论文发展脉络
Chat Paper
正在生成论文摘要