On the Optimization Landscape of Dynamic Output Feedback Linear Quadratic Control
IEEE TRANSACTIONS ON AUTOMATIC CONTROL(2024)
摘要
The convergence of policy gradient algorithms hinges on the optimization landscape of the underlying optimal control problem. Theoretical insights into these algorithms can often be acquired from analyzing those of linear quadratic control. However, most of the existing literature only considers the optimization landscape for static full-state or output feedback policies (controllers). In this article, we investigate the more challenging case of dynamic output-feedback policies for linear quadratic regulation (abbreviated as dLQR), which is prevalent in practice but has a rather complicated optimization landscape. We first show how the dLQR cost varies with the coordinate transformation of the dynamic controller, and then, derive the optimal transformation for a given observable stabilizing controller. One of our core results is the uniqueness of the stationary point of dLQR when it is observable, which provides an optimality certificate for solving dynamic controllers using policy gradient methods. Moreover, we establish conditions under which dLQR and linear quadratic Gaussian control are equivalent, thus providing a unified viewpoint of optimal control of both deterministic and stochastic linear systems. These results further shed light on designing policy gradient algorithms for more general decision-making problems with partially observed information.
更多查看译文
关键词
Dynamic output feedback,optimization landscape,policy gradient,reinforcement learning (RL)
AI 理解论文
溯源树
样例
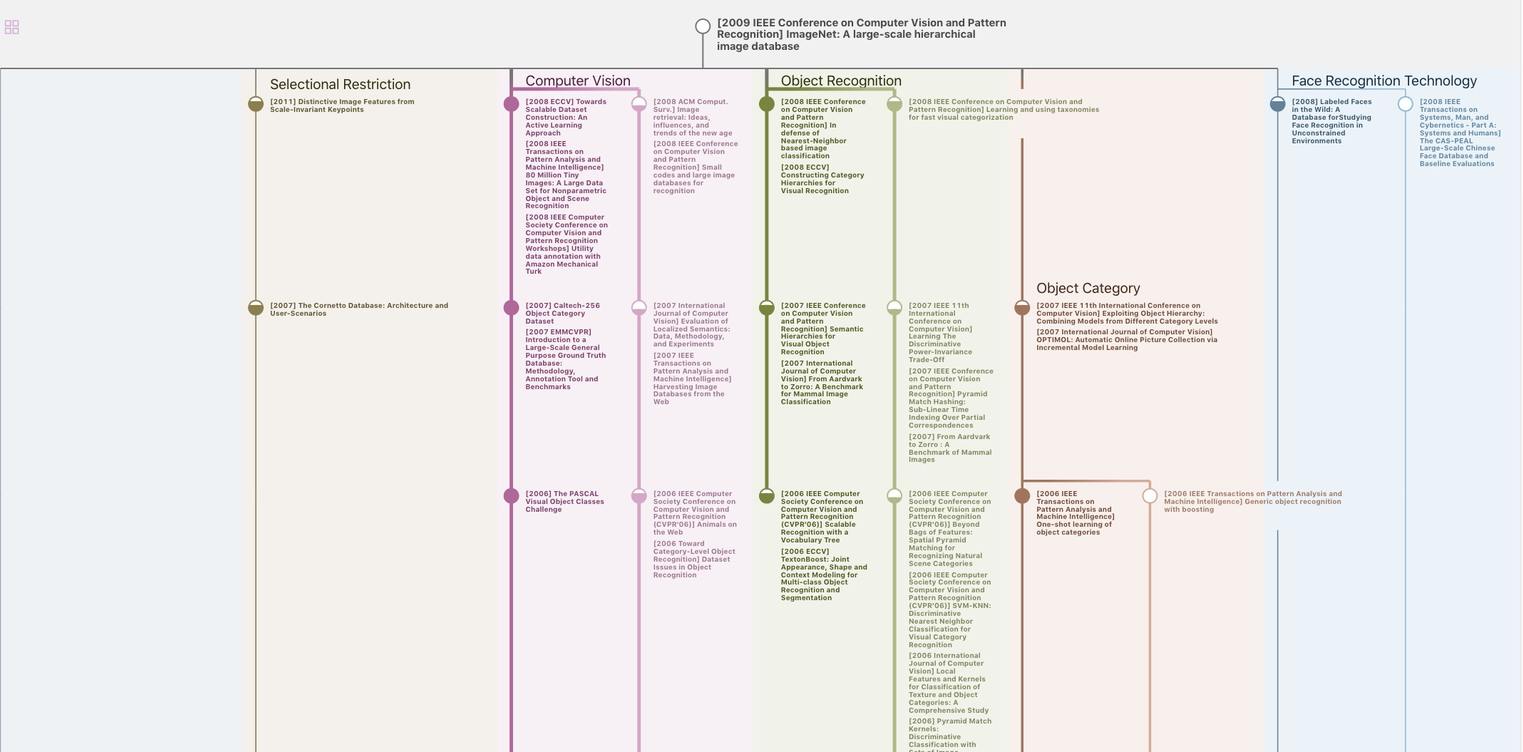
生成溯源树,研究论文发展脉络
Chat Paper
正在生成论文摘要