Learning-driven lossy image compression: A comprehensive survey
arxiv(2023)
摘要
In the field of image processing and computer vision (CV), machine learning (ML) architectures are widely used. Image compression problems can be solved using convolutional neural networks (CNNs). As a result of bandwidth and memory constraints, compression of images is a necessity. There are three types of information found in images: useful, redundant, and irrelevant. In this survey, we will discuss how ML is used to compress lossy images. Firstly, we describe the background of lossy image compression. Next, we classify ML-based image compression frameworks into subgroups based on their architectures. Auto-encoders (AEs), variational auto-encoders (VAEs), CNNs, recurrent neural networks (RNNs), long short-term memories (LSTMs), gated recurrent units (GRUs), generative adversarial networks (GANs), transformers, principal component analysis (PCA) and fuzzy means clustering are among these subgroups. By analyzing learning-driven image compression frameworks, we present pros and cons of each subgroup. Lastly, we outline several research gaps and future research directions in the field of ML-based image compression.
更多查看译文
关键词
Learned image compression,Deep learning,JPEG,End-to-end image compression,Machine learning,JPEG AI,Vision transformers
AI 理解论文
溯源树
样例
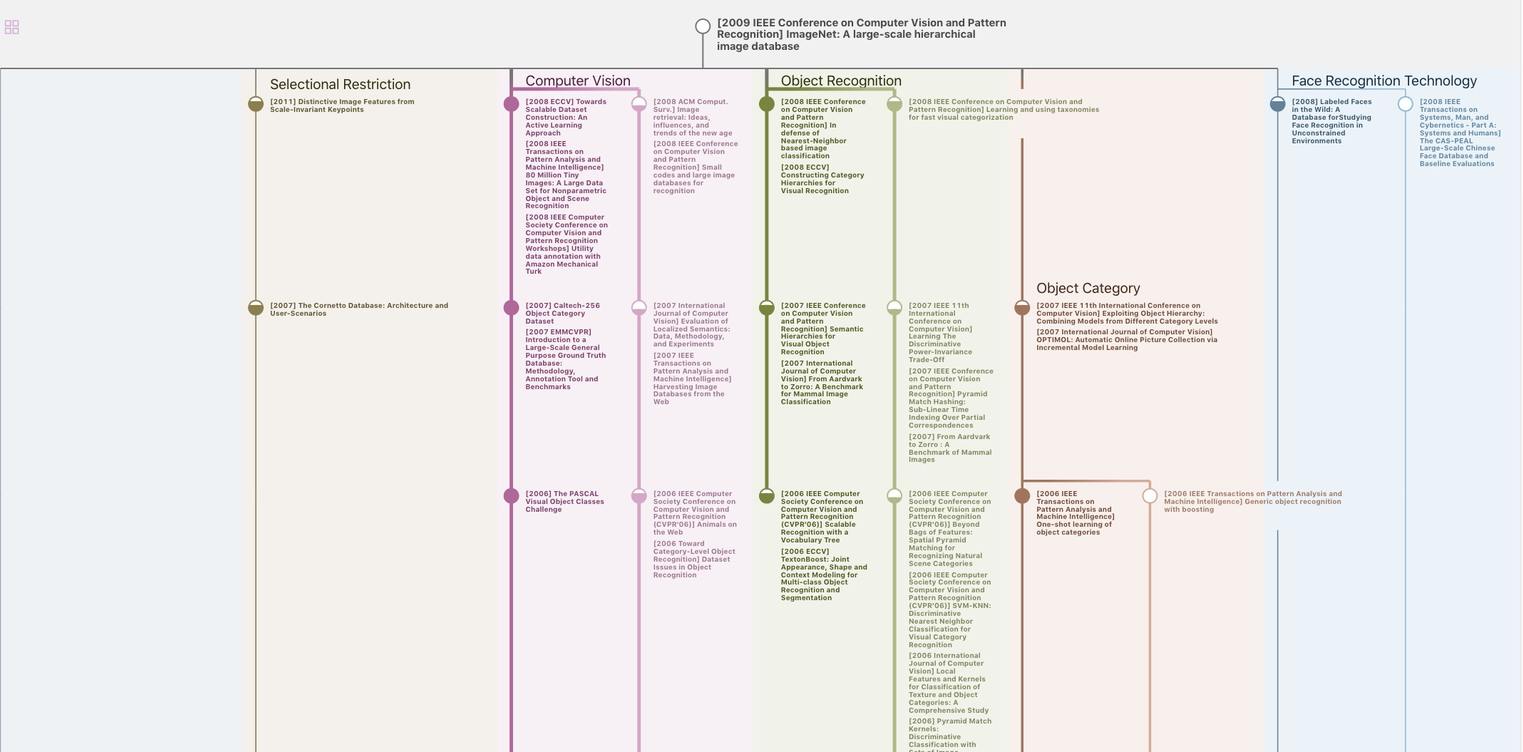
生成溯源树,研究论文发展脉络
Chat Paper
正在生成论文摘要