Online Auction-Based Incentive Mechanism Design for Horizontal Federated Learning with Budget Constraint
arxiv(2022)
摘要
Federated learning makes it possible for all parties with data isolation to train the model collaboratively and efficiently while satisfying privacy protection. To obtain a high-quality model, an incentive mechanism is necessary to motivate more high-quality workers with data and computing power. The existing incentive mechanisms are applied in offline scenarios, where the task publisher collects all bids and selects workers before the task. However, it is practical that different workers arrive online in different orders before or during the task. Therefore, we propose a reverse auction-based online incentive mechanism for horizontal federated learning with budget constraint. Workers submit bids when they arrive online. The task publisher with a limited budget leverages the information of the arrived workers to decide on whether to select the new worker. Theoretical analysis proves that our mechanism satisfies budget feasibility, computational efficiency, individual rationality, consumer sovereignty, time truthfulness, and cost truthfulness with a sufficient budget. The experimental results show that our online mechanism is efficient and can obtain high-quality models.
更多查看译文
关键词
incentive mechanism design,horizontal federated
AI 理解论文
溯源树
样例
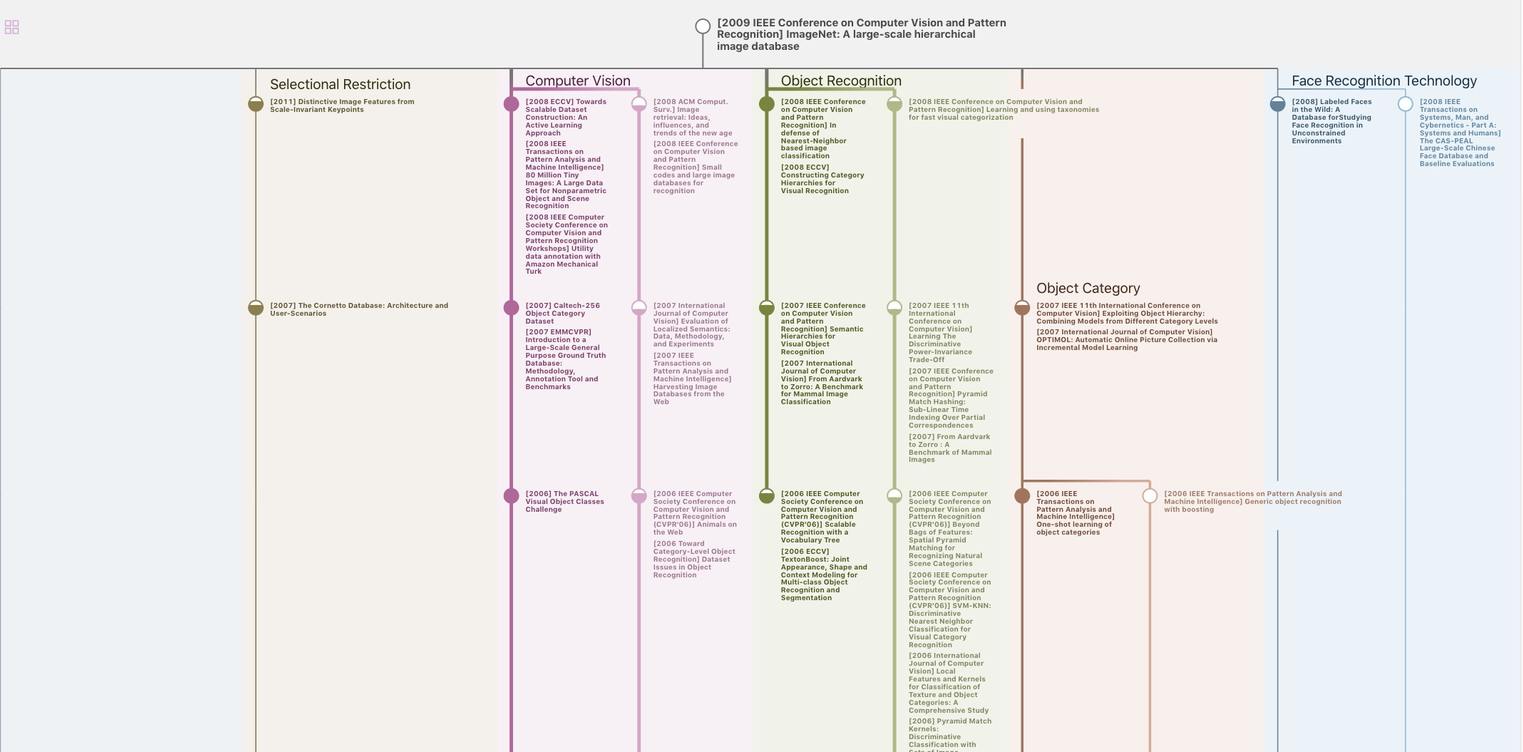
生成溯源树,研究论文发展脉络
Chat Paper
正在生成论文摘要