Machine-learning-based exploration to identify remodeling patterns associated with death or heart-transplant in pediatric-dilated cardiomyopathy
JOURNAL OF HEART AND LUNG TRANSPLANTATION(2022)
摘要
AIMS: We investigated left ventricular (LV) remodeling, mechanics, systolic and diastolic function, combined with clinical characteristics and heart-failure treatment in association to death or heart -transplant (DoT) in pediatric idiopathic, genetic or familial dilated cardiomyopathy (DCM), using interpretable machine-learning.& nbsp;METHODS AND RESULTS: Echocardiographic and clinical data from pediatric DCM and healthy controls were retrospectively analyzed. Machine-learning included whole cardiac-cycle regional longitudinal strain, aortic, mitral and pulmonary vein Doppler velocity traces, age and body surface area. We used unsupervised multiple kernel learning for data dimensionality reduction, positioning patients based on complex conglomerate information similarity. Subsequently, k-means identified groups with similar phenotypes. The proportion experiencing DoT was evaluated. Pheno-grouping identified 5 clinically distinct groups that were associated with differing proportions of DoT. All healthy controls clustered in groups 1 to 2, while all, but one, DCM subjects, clustered in groups 3 to 5; internally validating the algorithm. Cluster-5 comprised the oldest, most medicated patients, with combined systolic and diastolic heart-failure and highest proportion of DoT. Cluster 4 included the youngest patients characterized by severe LV remodeling and systolic dysfunction, but mild diastolic dysfunction and the second-highest proportion of DoT. Cluster-3 comprised young patients with moderate remodeling and systolic dysfunction, preserved apical strain, pronounced diastolic dysfunction and lowest proportion of DoT.& nbsp;CONCLUSIONS: Interpretable machine-learning, using full cardiac-cycle systolic and diastolic data, mechanics and clinical parameters, can potentially identify pediatric DCM patients at high-risk for DoT, and delineate mechanisms associated with risk. This may facilitate more precise prognostication and treatment of pediatric DCM.J Heart Lung Transplant 2022;41:516-526 (c) 2021 The Author(s). Published by Elsevier Inc. on behalf of International Society for Heart and Lung Transplantation. This is an open access article under the CC BY-NC-ND license (http://creativecommons.org/licenses/by-nc-nd/4.0/)
更多查看译文
关键词
dilated cardiomyopathy,pediatrics,heart failure,machine-learning,echocardiography,strain,death,heart transplantation
AI 理解论文
溯源树
样例
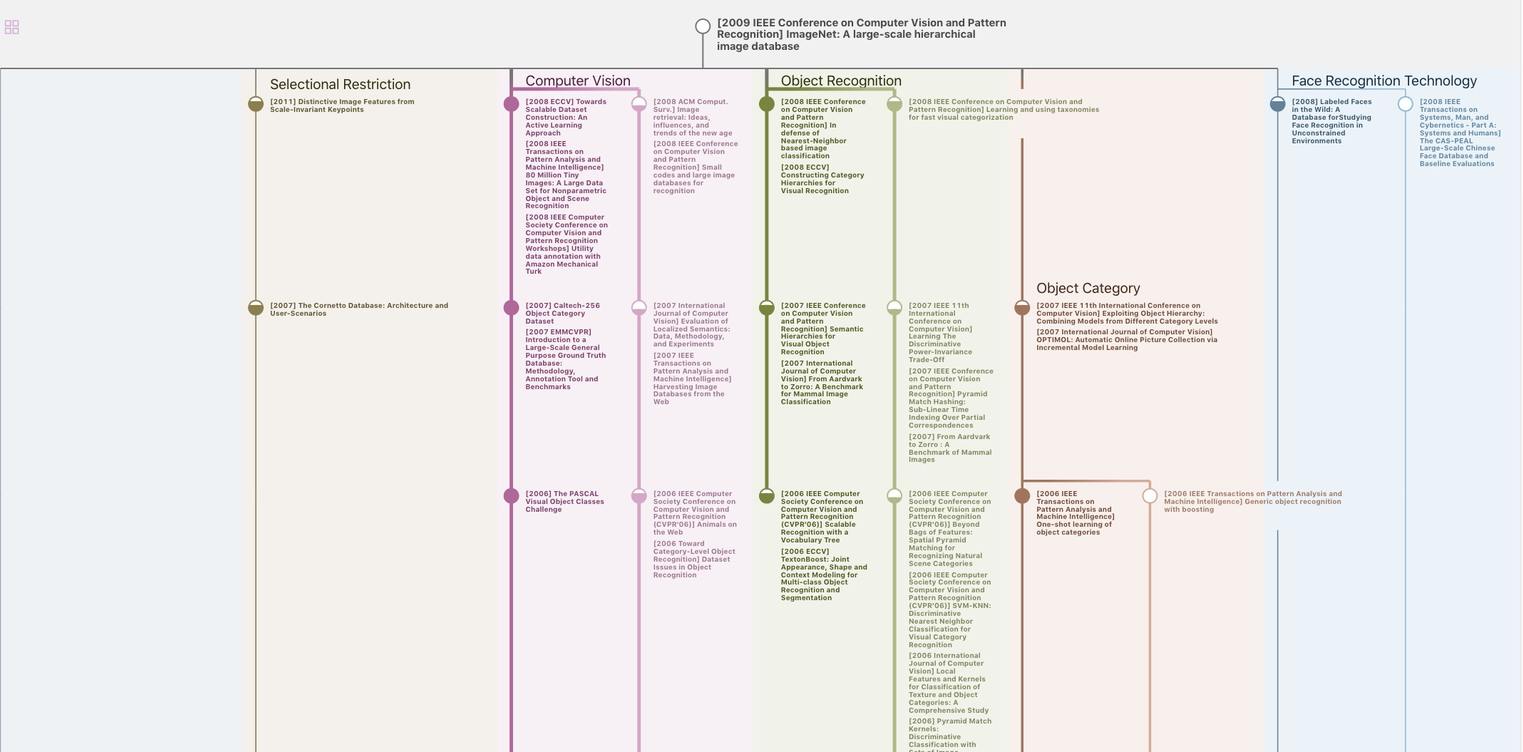
生成溯源树,研究论文发展脉络
Chat Paper
正在生成论文摘要