Symptom-Based Predictive Model of COVID-19 Disease in Children
VIRUSES-BASEL(2022)
摘要
Background: Testing for severe acute respiratory syndrome coronavirus 2 (SARS-CoV-2) infection is neither always accessible nor easy to perform in children. We aimed to propose a machine learning model to assess the need for a SARS-CoV-2 test in children (<16 years old), depending on their clinical symptoms. Methods: Epidemiological and clinical data were obtained from the REDCap(R) registry. Overall, 4434 SARS-CoV-2 tests were performed in symptomatic children between 1 November 2020 and 31 March 2021, 784 were positive (17.68%). We pre-processed the data to be suitable for a machine learning (ML) algorithm, balancing the positive-negative rate and preparing subsets of data by age. We trained several models and chose those with the best performance for each subset. Results: The use of ML demonstrated an AUROC of 0.65 to predict a COVID-19 diagnosis in children. The absence of high-grade fever was the major predictor of COVID-19 in younger children, whereas loss of taste or smell was the most determinant symptom in older children. Conclusions: Although the accuracy of the models was lower than expected, they can be used to provide a diagnosis when epidemiological data on the risk of exposure to COVID-19 is unknown.
更多查看译文
关键词
machine learning, deep learning, paediatrics, SARS-CoV-2, COVID-19, epidemiology, microbiology
AI 理解论文
溯源树
样例
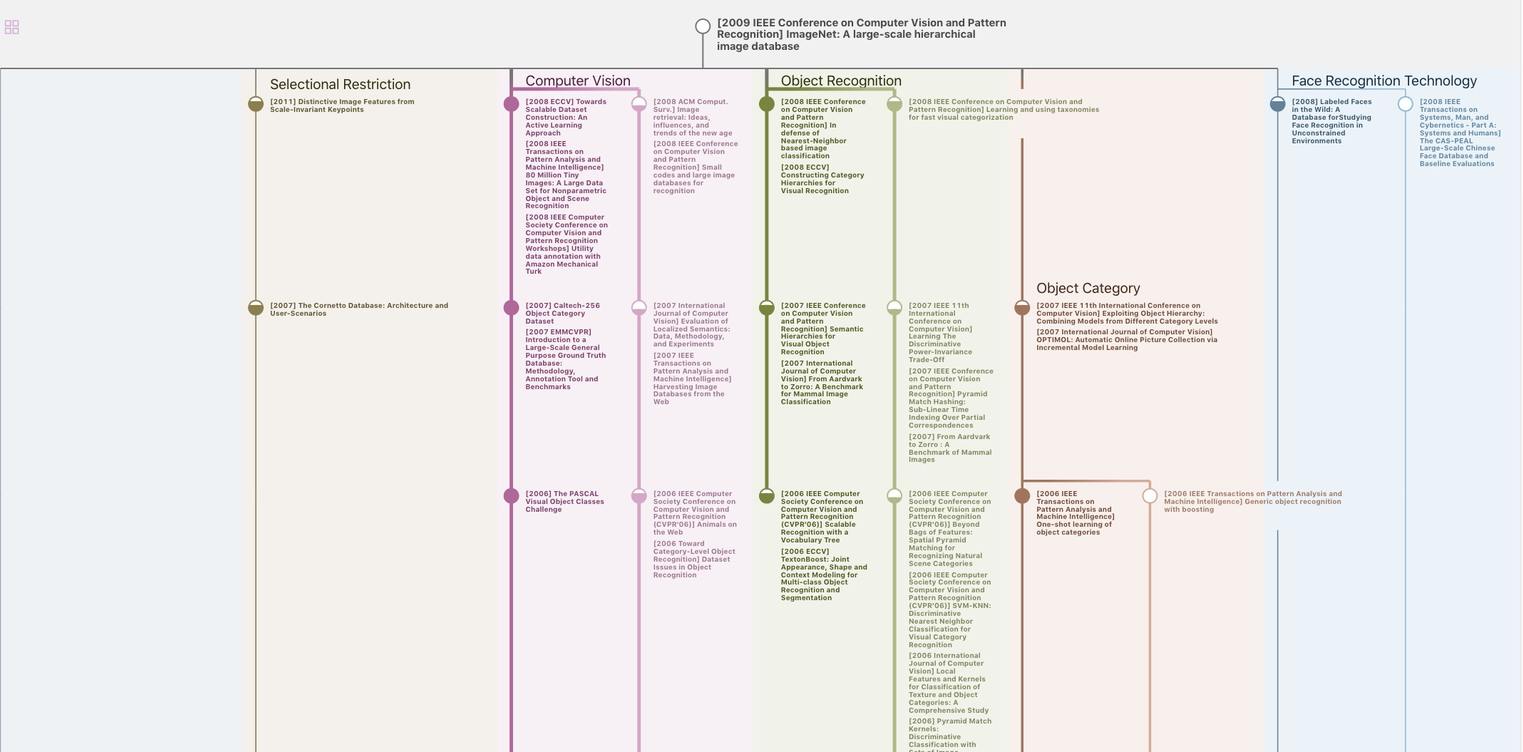
生成溯源树,研究论文发展脉络
Chat Paper
正在生成论文摘要