Framework for Denoising Monte Carlo Photon Transport Simulations Using Deep Learning
Journal of Biomedical Optics(2022)
摘要
Significance The Monte Carlo (MC) method is widely used as the gold-standard for modeling light propagation inside turbid media like human tissues, but combating its inherent stochastic noise requires one to simulate large number photons, resulting in high computational burdens.
Aim We aim to develop an effective image denoising technique using deep learning (DL) to dramatically improve low-photon MC simulation result quality, equivalently bringing further acceleration to the MC method.
Approach We have developed a cascade-network combining DnCNN with UNet, in the meantime, extended a range of established image denoising neural-network architectures, including DnCNN, UNet, DRUNet, and ResMCNet, in handling three-dimensional (3-D) MC data and compared their performances against model-based denoising algorithms. We have also developed a simple yet effective approach to create synthetic datasets that can be used to train DL based MC denoisers.
Results Overall, DL based image denoising algorithms exhibit significantly higher image quality improvements over traditional model-based denoising algorithms. Among the tested DL denoisiers, our Cascade network yields a 14 - 19 dB improvement in signal-noise ratio (SNR), which is equivalent to simulating 25 × to 78 × more photons. Other DL-based methods yielded similar results, with our method performing noticeably better with low-photon inputs, and ResMCNet along with DRUNet performing better with high-photon inputs. Our Cascade network achieved the highest quality when denoising complex domains, including brain and mouse atlases.
Conclusion Incorporating state-of-the-art DL denoising techniques can equivalently reduce the computation time of MC simulations by one to two orders of magnitude. Our open-source MC denoising codes and data can be freely accessed at .
### Competing Interest Statement
The authors have declared no competing interest.
更多查看译文
AI 理解论文
溯源树
样例
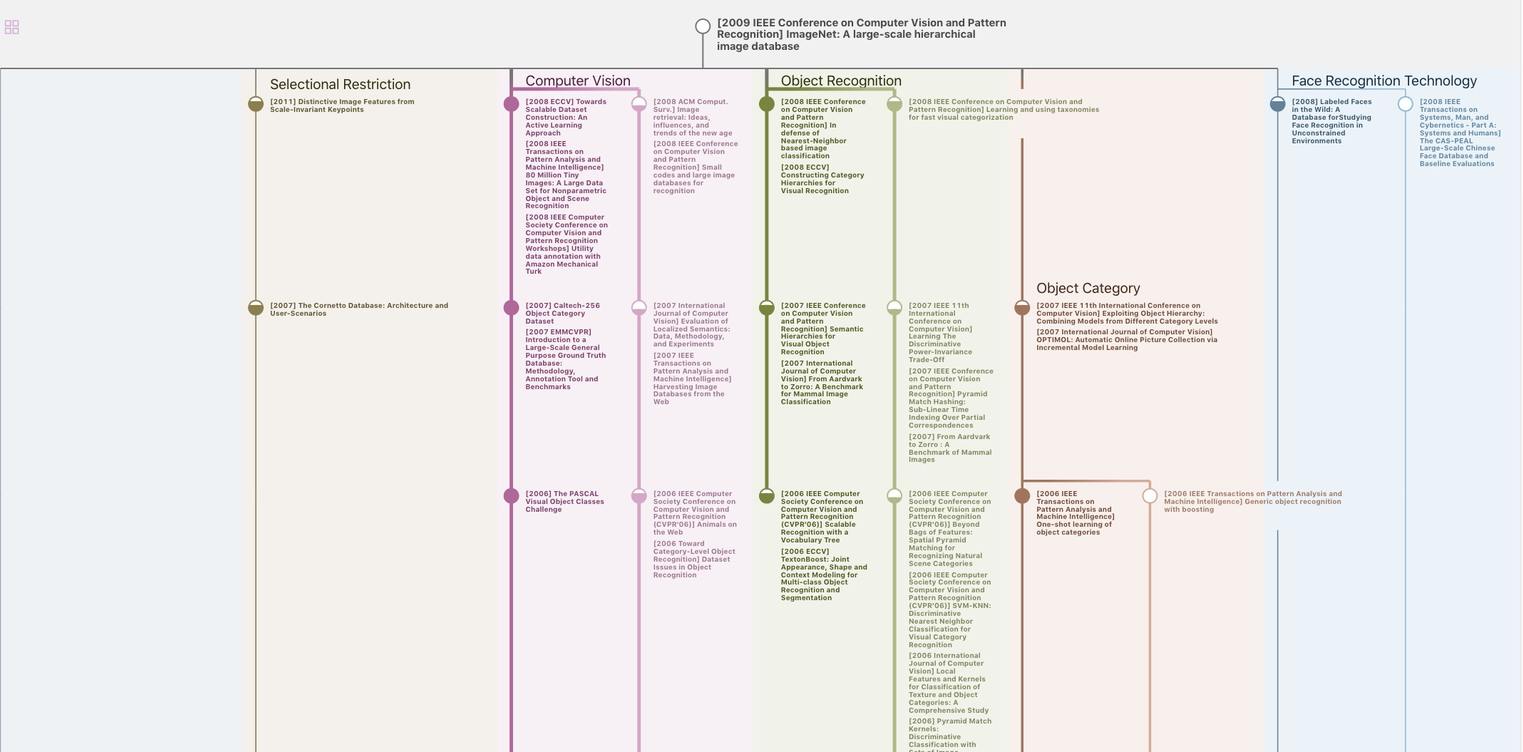
生成溯源树,研究论文发展脉络
Chat Paper
正在生成论文摘要