Data-Driven Divide-and-Conquer for Estimating Build Times of 3D Objects.
2021 International Conference on Data Mining Workshops (ICDMW)(2021)
摘要
Accurate estimation of build times of 3D objects is of great importance in the different phases of an additive manufacturing process. The existing physics-based models can very precisely tackle this task but at the cost of spending a considerable amount of time. An alternative solution is to use a data-driven machine learning method for build time estimation (BTE). However, estimating build times of a dataset of objects with diverse and heterogeneous characteristics is a challenging task for a single learning algorithm. The aim of this study is therefore to investigate the value of the divide-and-conquer strategy in partitioning the dataset into subsets of homogeneous objects to facilitate the BTE task for the examined learning models. The comprehensive experiments performed in this study prove that this strategy is indeed capable of providing accurate BTEs and can outperform the performance of a single learning method trained with all objects in the dataset.
更多查看译文
关键词
additive manufacturing,machine learning,feature engineering and selection,divide-and-conquer
AI 理解论文
溯源树
样例
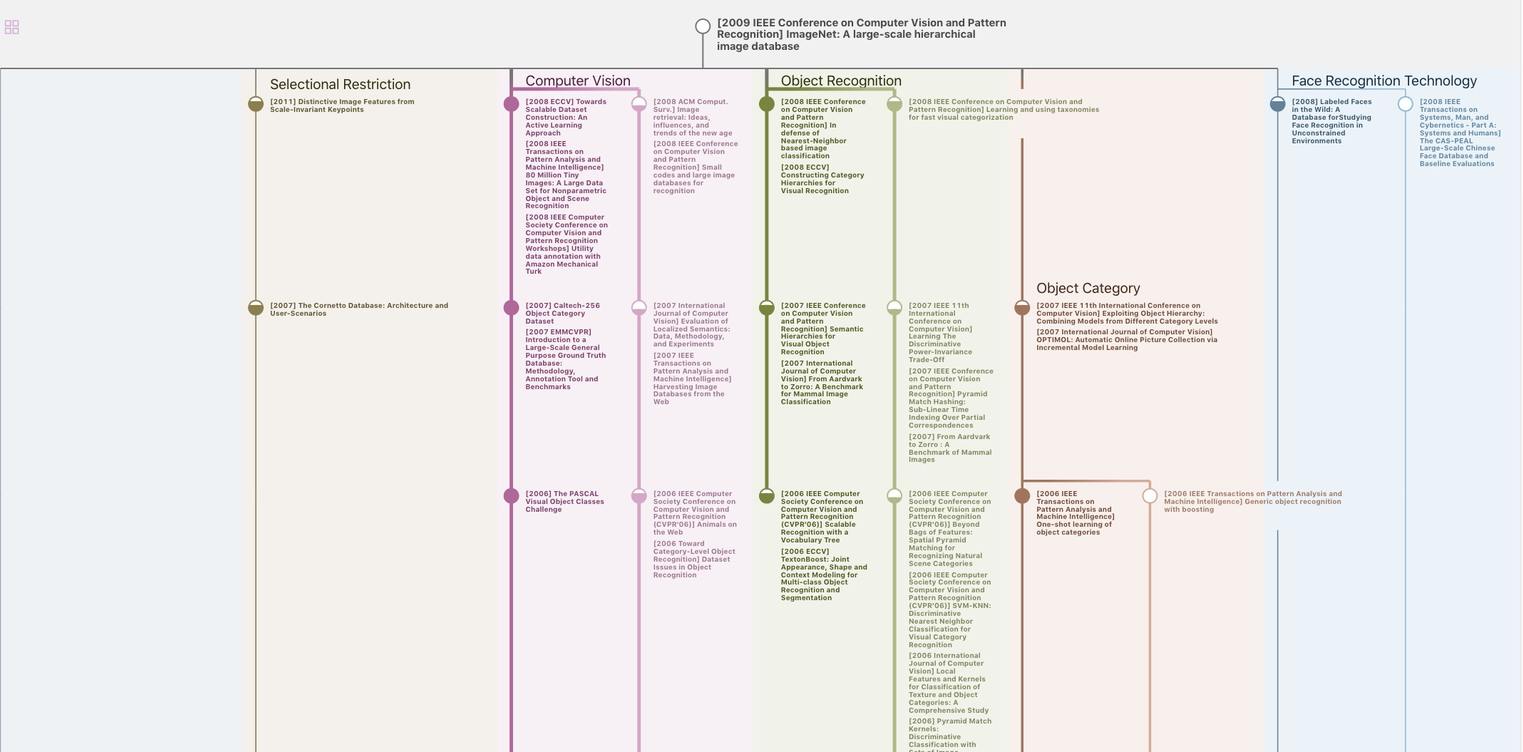
生成溯源树,研究论文发展脉络
Chat Paper
正在生成论文摘要