Mining High Utility Subgraphs.
2021 International Conference on Data Mining Workshops (ICDMW)(2021)
摘要
High utility pattern mining discovers more realistic and important knowledge than the traditional frequent pattern mining by considering non-binary occurrences of items inside transactions/sequences and different weight values for different items. Research has been done for high utility itemset and sequence mining. However, graphs have ubiquitous applications, including social networks, road networks, webpage access networks, citation networks, and biological protein-protein interaction networks. Moreover, it is more challenging to devise a graph mining approach than the traditional itemset/sequence mining due to the structural complexity of graphs. In this work, we propose a generic framework for utility-based graph pattern mining. A complete algorithm is presented for high utility subgraph mining and to the best of our knowledge, we are the first to develop a complete framework for high utility subgraph mining. The number of candidates generated in the search space grows exponentially for weighted or utility-based pattern mining. To address this problem, we introduce a pruning technique for effective pruning of the search space. We conducted experiments on various datasets to analyze the performance of the algorithm. Our experimental results show the effectiveness of our proposed algorithm to extract high utility subgraph patterns.
更多查看译文
关键词
Graph Mining,High Utility Pattern Mining
AI 理解论文
溯源树
样例
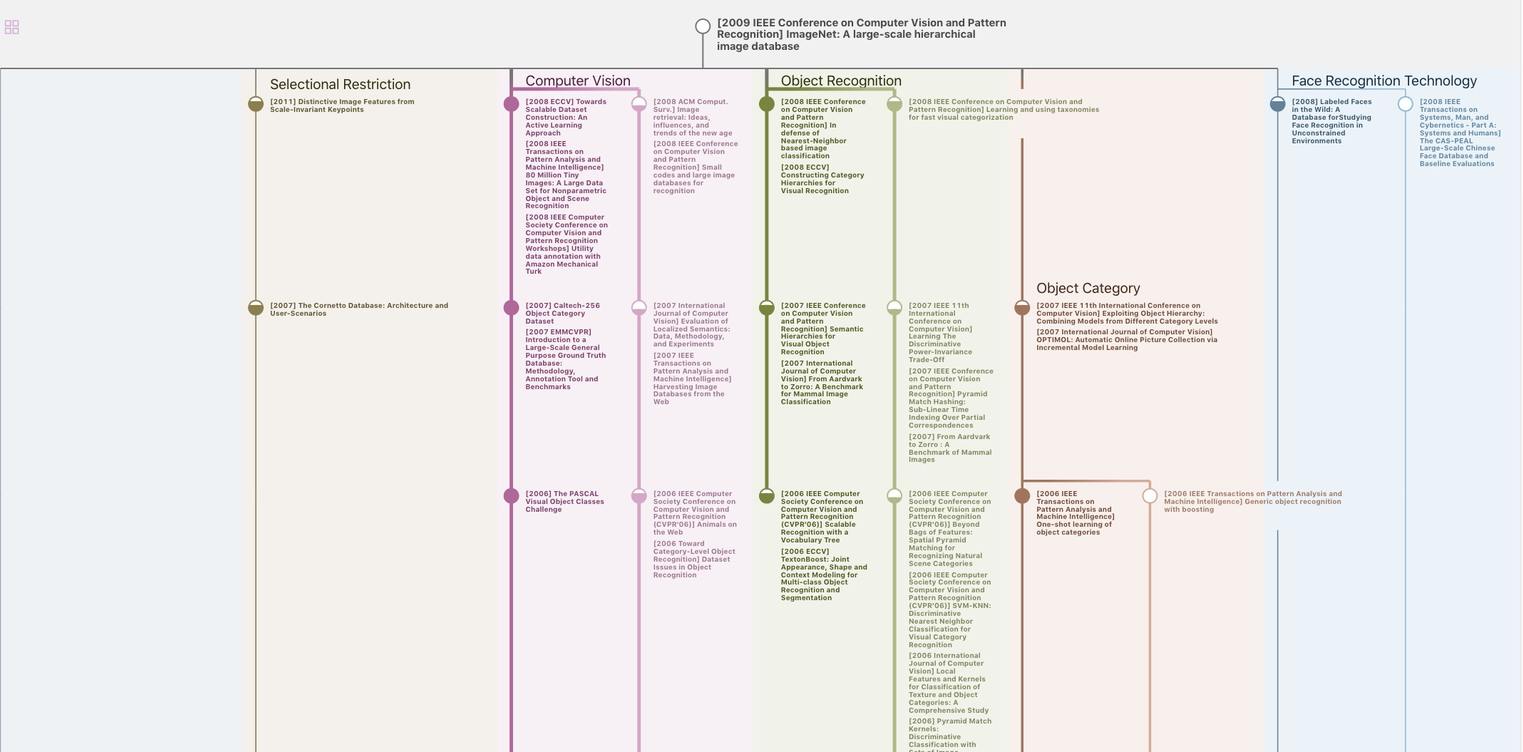
生成溯源树,研究论文发展脉络
Chat Paper
正在生成论文摘要