Emulation of the cosmic dawn 21-cm power spectrum and classification of excess radio models using an artificial neural network
MONTHLY NOTICES OF THE ROYAL ASTRONOMICAL SOCIETY(2024)
摘要
The cosmic 21-cm line of hydrogen is expected to be measured in detail by the next generation of radio telescopes. The enormous data set from future 21-cm surveys will revolutionize our understanding of early cosmic times. We present a machine learning approach based on an artificial neural network that uses emulation in order to uncover the astrophysics in the epoch of reionization and cosmic dawn. Using a seven-parameter astrophysical model that covers a very wide range of possible 21-cm signals, over the redshift range 6 to 30 and wavenumber range 0.05 to 1 Mpc(-1) we emulate the 21-cm power spectrum with a typical accuracy of 10 - 20 per. As a realistic example, we train an emulator using the power spectrum with an optimistic noise model of the square kilometre array (SKA). Fitting to mock SKA data results in a typical measurement accuracy of 2.8 per cent in the optical depth to the cosmic microwave background, 34 per cent in the star-formation efficiency of galactic haloes, and a factor of 9.6 in the X-ray efficiency of galactic haloes. Also, with our modelling we reconstruct the true 21-cm power spectrum from the mock SKA data with a typical accuracy of 15 - 30 per cent. In addition to standard astrophysical models, we consider two exotic possibilities of strong excess radio backgrounds at high redshifts. We use a neural network to identify the type of radio background present in the 21-cm power spectrum, with an accuracy of 87 per cent for mock SKA data.
更多查看译文
关键词
methods: numerical,methods: statistical,dark ages, reionization, first stars,cosmology: theory
AI 理解论文
溯源树
样例
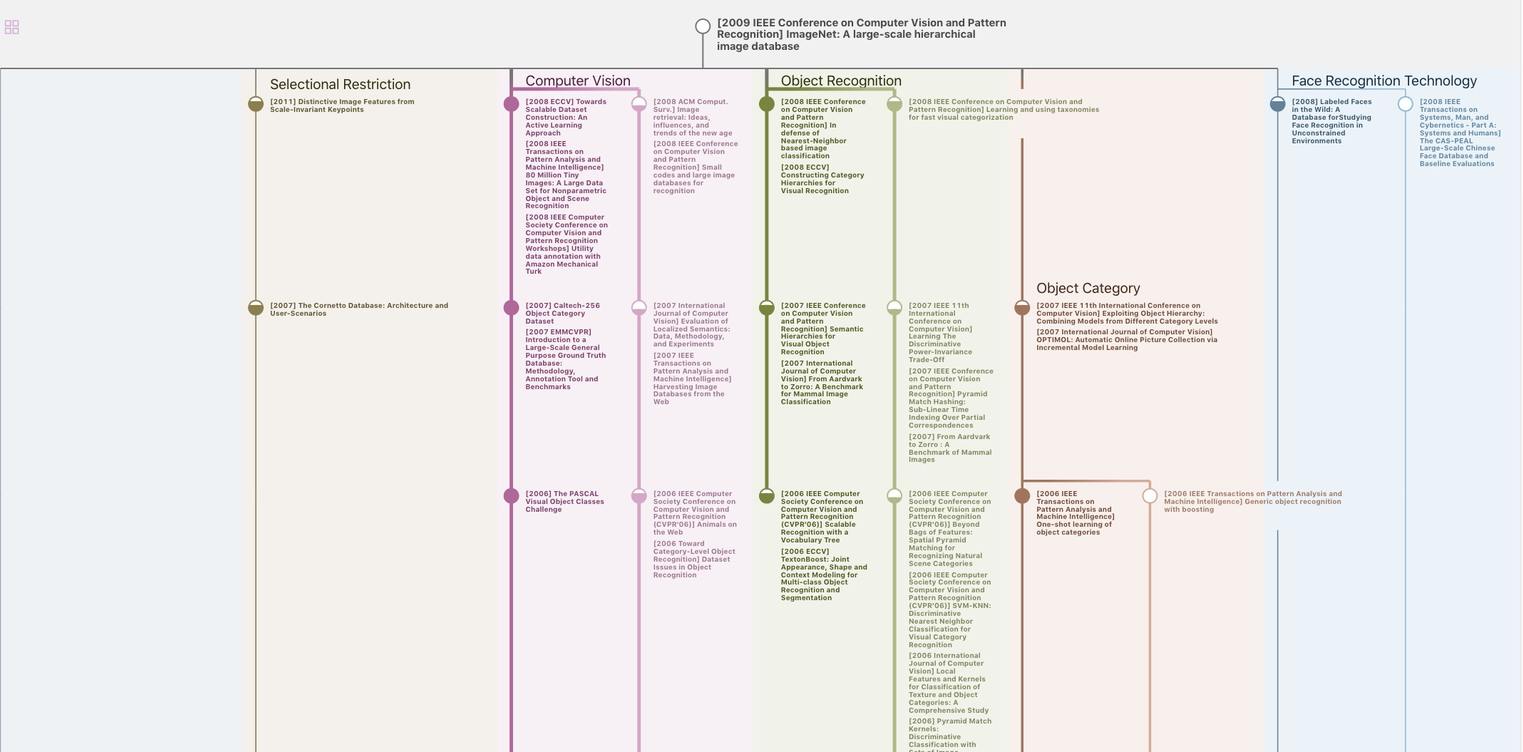
生成溯源树,研究论文发展脉络
Chat Paper
正在生成论文摘要