AirPose: Multi-View Fusion Network for Aeria 3D Human Pose and Shape Estimation
IEEE ROBOTICS AND AUTOMATION LETTERS(2022)
摘要
In this letter, we present a novel markerless 3D human motion capture (MoCap) system for unstructured, outdoor environments that uses a team of autonomous unmanned aerial vehicles (UAVs) with on-hoard RGB cameras and computation. Existing methods are limited by calibrated cameras and off-line processing. Thus, we present the first method (AirPose) to estimate human pose and shape using images captured by multiple extrinsically uncalibrated flying cameras. AirPose itself calibrates the cameras relative to the person instead of relying on any pre-calibration. It uses distributed neural networks running on each UAV that communicate viewpoint-independent information with each other about the person (i.e., their 3D shape and articulated pose). The person's shape and pose are parameterized using the SMPL-X body model, resulting in a compact representation, that minimizes communication between the UAVs. The network is trained using synthetic images of realistic virtual environments, and fine-tuned on a small set of real images. We also introduce an optimization-based post-processing method (AirPose+) for offline applications that require higher MoCap quality. We make our method's code and data available for research at https://github.com/robot-perception-group/AirPose. A video describing the approach and results is available at https://youtu.be/xLYelTNHsfs.
更多查看译文
关键词
Aerial systems, deep learning for visual perception, datasets for human motion, human detection and tracking, perception and autonomy, sensor fusion
AI 理解论文
溯源树
样例
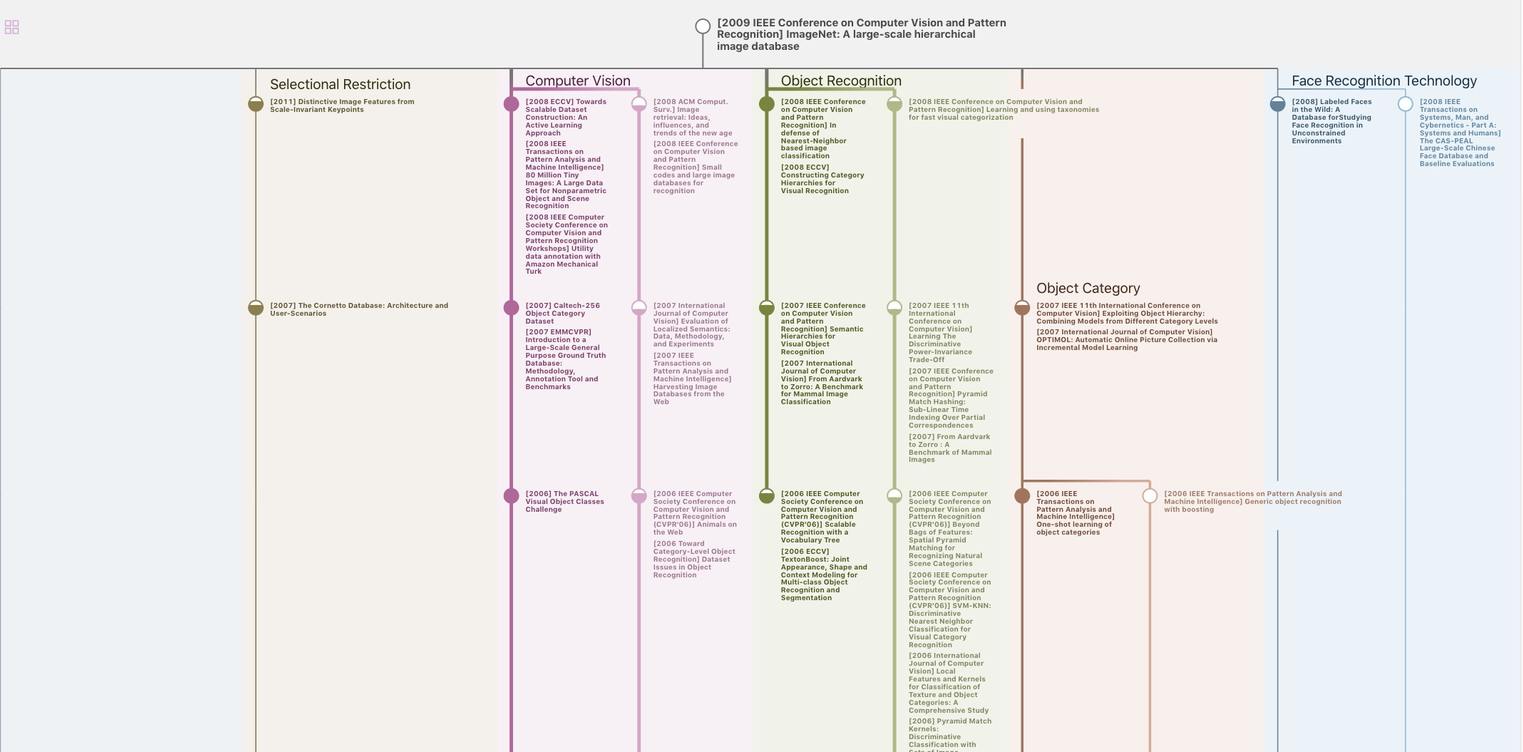
生成溯源树,研究论文发展脉络
Chat Paper
正在生成论文摘要