Statistical Learning for Individualized Asset Allocation
arxiv(2022)
摘要
We establish a high-dimensional statistical learning framework for individualized asset allocation. Our proposed methodology addresses continuous-action decision-making with a large number of characteristics. We develop a discretization approach to model the effect of continuous actions and allow the discretization frequency to be large and diverge with the number of observations. The value function of continuous-action is estimated using penalized regression with our proposed generalized penalties that are imposed on linear transformations of the model coefficients. We show that our proposed Discretization and Regression with generalized fOlded concaVe penalty on Effect discontinuity (DROVE) approach enjoys desirable theoretical properties and allows for statistical inference of the optimal value associated with optimal decision-making. Empirically, the proposed framework is exercised with the Health and Retirement Study data in finding individualized optimal asset allocation. The results show that our individualized optimal strategy improves the population financial well-being.
更多查看译文
关键词
Continuous-action decision-making,High-dimensional statistical learning,Individualization,Penalized regression
AI 理解论文
溯源树
样例
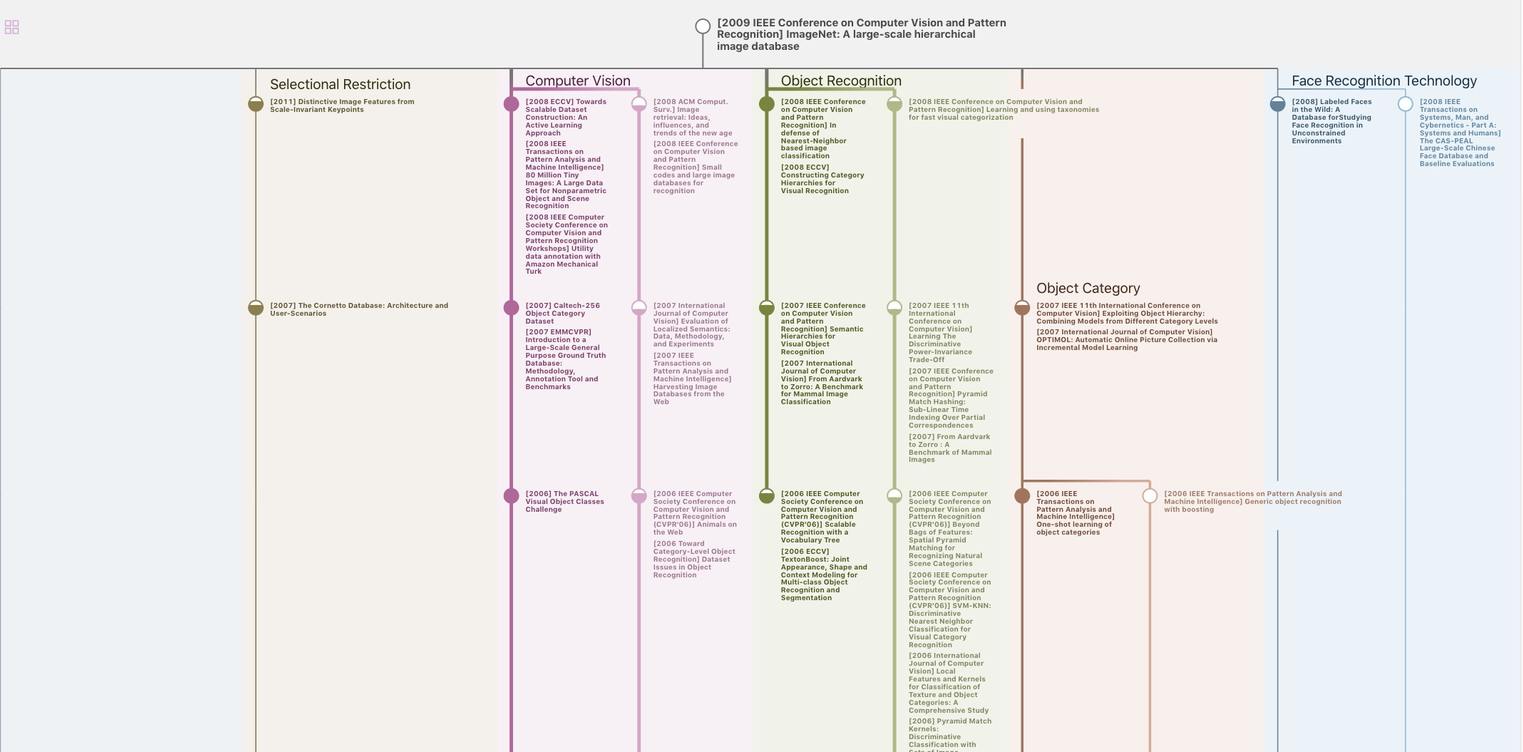
生成溯源树,研究论文发展脉络
Chat Paper
正在生成论文摘要