Deep self-consistent learning of local volatility
arxiv(2021)
摘要
We present an algorithm for the calibration of local volatility from market option prices through deep self-consistent learning, by approximating market option prices and local volatility using deep neural networks. Our method uses the initial-boundary value problem of the underlying Dupire's partial differential equation solved by the parameterized option prices to bring corrections to the parameterization in a self-consistent way. By exploiting the differentiability of the neural networks, we can evaluate Dupire's equation locally at each maturity-strike pair; while by exploiting their continuity, we sample maturity-strike pairs uniformly from a given domain, going beyond the discrete points where the options are quoted. For comparison with existing approaches, the proposed method is tested on both synthetic and market option prices, which shows an improved performance in terms of repricing error, no violation of the no-arbitrage constraints, and smoothness of the calibrated local volatility.
更多查看译文
关键词
local volatility,learning,self-consistent
AI 理解论文
溯源树
样例
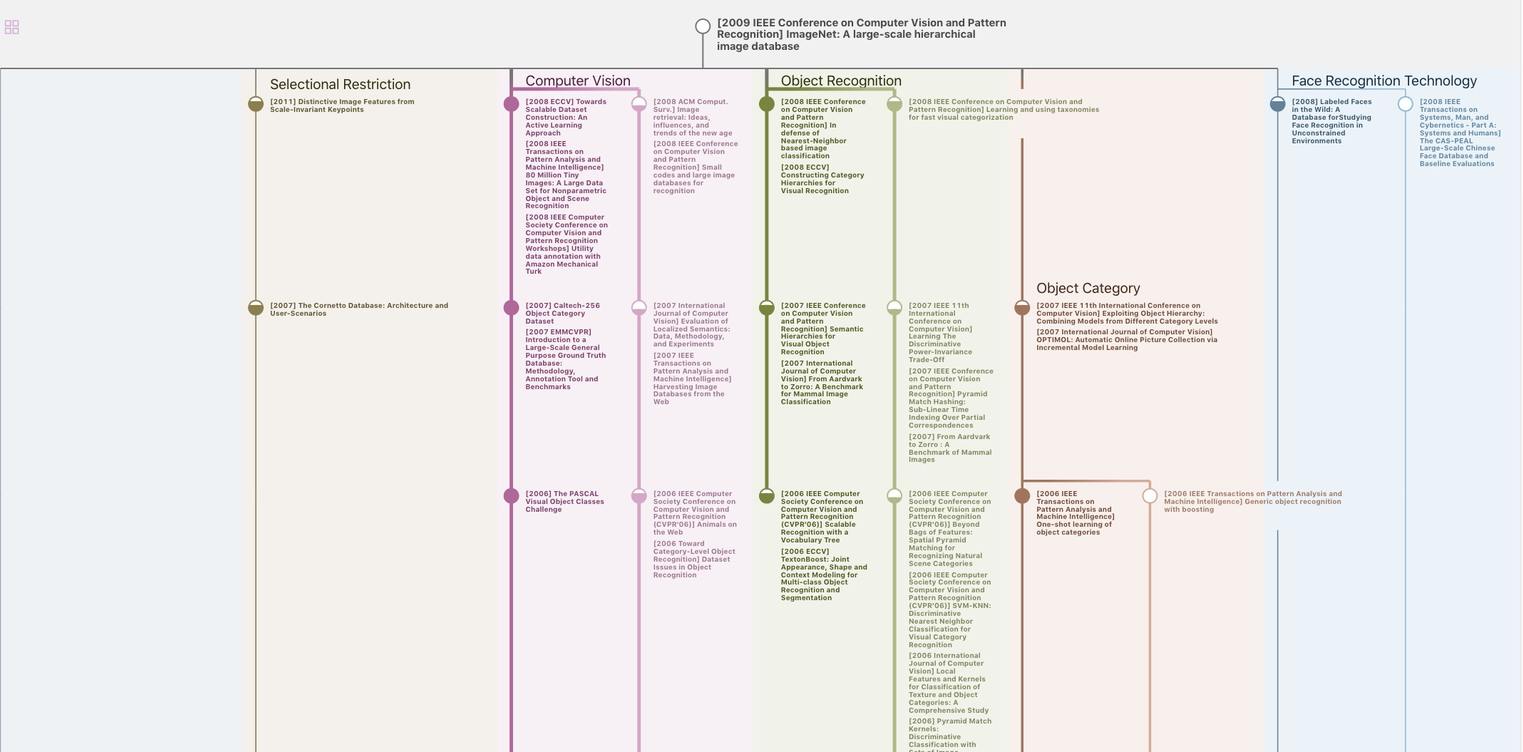
生成溯源树,研究论文发展脉络
Chat Paper
正在生成论文摘要