Full wCDM analysis of KiDS-1000 weak lensing maps using deep learning
PHYSICAL REVIEW D(2022)
摘要
We present a full forward-modeled wCDM analysis of the KiDS-1000 weak lensing maps using graphconvolutional neural networks (GCNN). Utilizing the CosmoGrid, a novel massive simulation suite spanning six different cosmological parameters, we generate almost one million tomographic mock surveys on the sphere. Due to the large dataset size and survey area, we perform a spherical analysis while limiting our map resolution to HEALPix nside = 512. We marginalize over systematics such as photometric redshift errors, multiplicative calibration and additive shear bias. Furthermore, we use a map-level implementation of the nonlinear intrinsic alignment model along with a novel treatment of baryonic feedback to incorporate additional astrophysical nuisance parameters. We also perform a spherical power spectrum analysis for comparison. The constraints of the cosmological parameters are generated using a likelihood-free inference method called Gaussian process approximate Bayesian computation (GPABC). Finally, we check that our pipeline is robust against choices of the simulation parameters. We find constraints on the degeneracy p ffiffiffiffiffiffiffiffiffiffiffiffiffiffiffi parameter of S8 ??? ??8 ??M/0.3 = 0.78??0.06 GCNN analysis, improving the former by 16%. This is consistent with earlier analyses of the 2-point function, albeit slightly higher. Baryonic corrections generally broaden the constraints on the degeneracy parameter by about 10%. These results offer great prospects for full machine learning based analyses of ongoing and future weak lensing surveys.
更多查看译文
关键词
maps,deep learning,<mmlmath xmlnsmml=http//wwww3org/1998/math/mathml
AI 理解论文
溯源树
样例
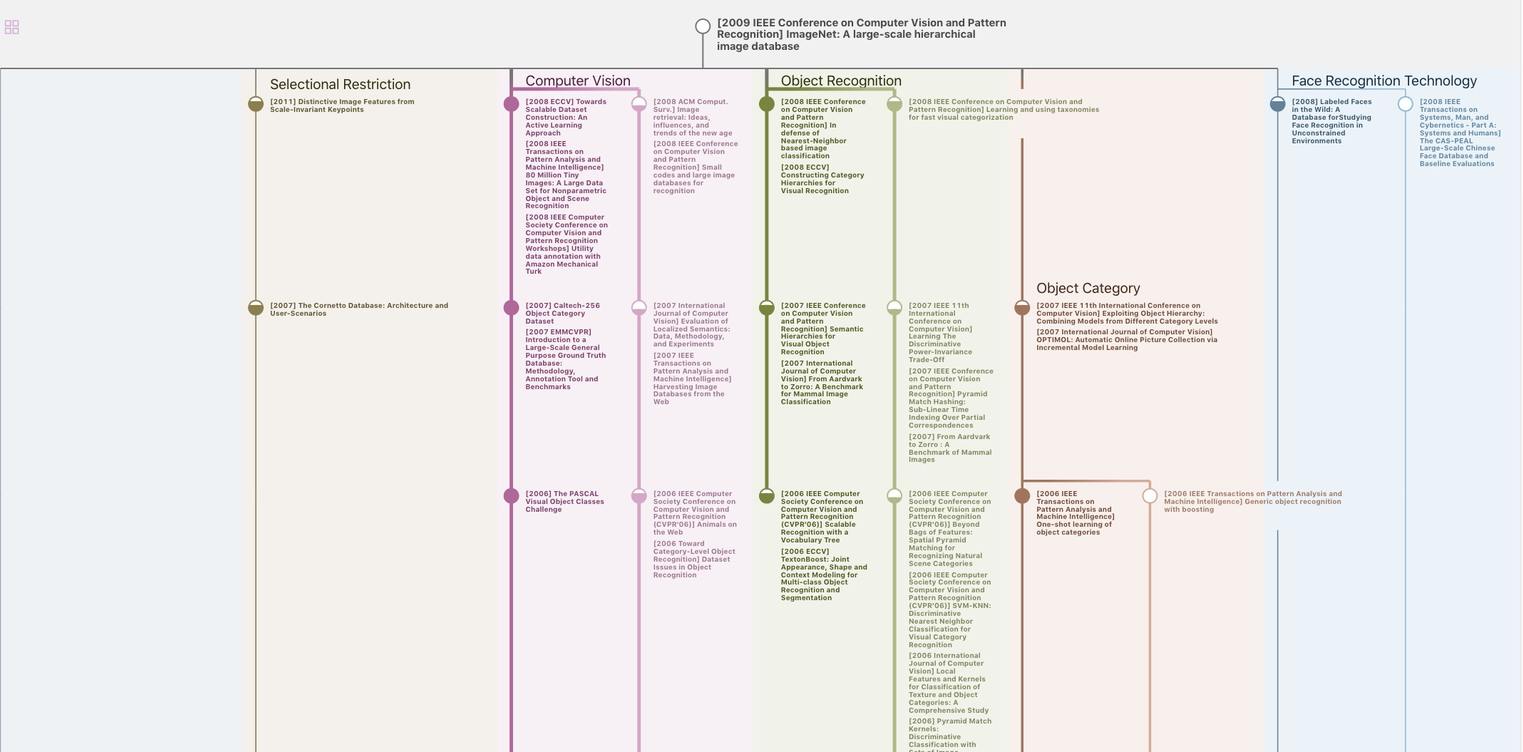
生成溯源树,研究论文发展脉络
Chat Paper
正在生成论文摘要