Superpixel Pre-segmentation of HER2 Slides for Efficient Annotation.
Bildverarbeitung für die Medizin(2022)
摘要
Supervised deep learning has shown state-of-the-art performance for medical image segmentation across different applications, including histopathology and cancer research; however, the manual annotation of such data is extremely laborious. In this work, we explore the use of superpixel approaches to compute a pre-segmentation of HER2 stained images for breast cancer diagnosis that facilitates faster manual annotation and correction in a second step. Four methods are compared: Standard Simple Linear Iterative Clustering (SLIC) as a baseline, a domain adapted SLIC, and superpixels based on feature embeddings of a pretrained ResNet-50 and a denoising autoencoder. To tackle oversegmentation, we propose to hierarchically merge superpixels, based on their content in the respective feature space. When evaluating the approaches on fully manually annotated images, we observe that the autoencoder-based superpixels achieve a 23% increase in boundary F1 score compared to the baseline SLIC superpixels. Furthermore, the boundary F1 score increases by 73% when hierarchical clustering is applied on the adapted SLIC and the autoencoder-based superpixels. These evaluations show encouraging first results for a pre-segmentation for efficient manual refinement without the need for an initial set of annotated training data.
更多查看译文
关键词
her2 slides,annotation,pre-segmentation
AI 理解论文
溯源树
样例
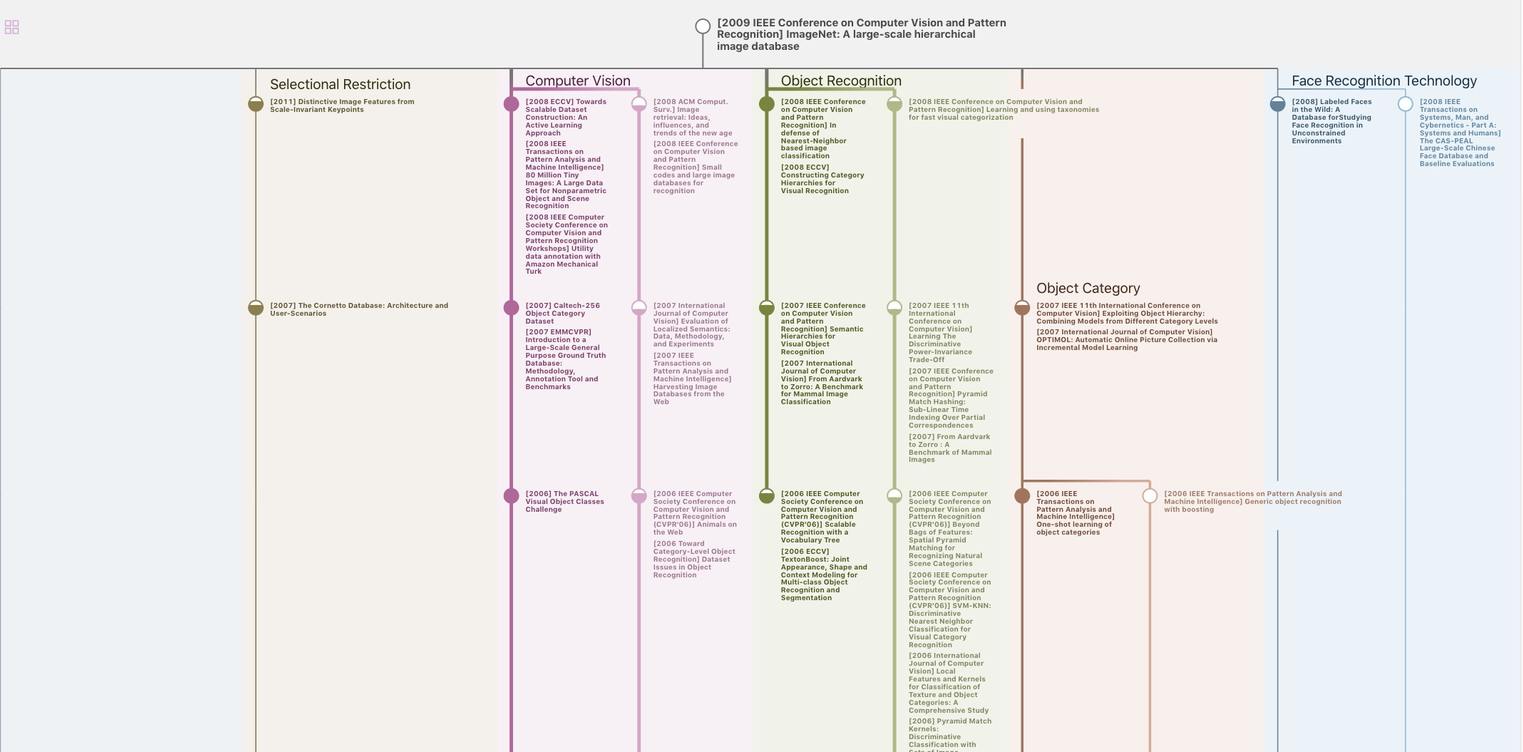
生成溯源树,研究论文发展脉络
Chat Paper
正在生成论文摘要