Gaining or losing perspective for convex multivariate functions on a simplex
Journal of Global Optimization(2024)
摘要
MINLO (mixed-integer nonlinear optimization) formulations of the disjunction between the origin and a polytope via a binary indicator variable have broad applicability in nonlinear combinatorial optimization, for modeling a fixed cost c associated with carrying out a set of d activities and a convex variable cost function f associated with the levels of the activities. The perspective relaxation is often used to solve such models to optimality in a branch-and-bound context, especially in the context in which f is univariate (e.g., in Markowitz-style portfolio optimization). But such a relaxation typically requires conic solvers and are typically not compatible with general-purpose NLP software which can accommodate additional classes of constraints. This motivates the study of weaker relaxations to investigate when simpler relaxations may be adequate. Comparing the volume (i.e., Lebesgue measure) of the relaxations as means of comparing them, we lift some of the results related to univariate functions f to the multivariate case. Along the way, we survey, connect and extend relevant results on integration over a simplex, some of which we concretely employ, and others of which can be used for further exploration on our main subject.
更多查看译文
关键词
Mixed-integer nonlinear optimization,Global optimization,Convex relaxation,Perspective,Simplex,Polytope,Volume,Integration
AI 理解论文
溯源树
样例
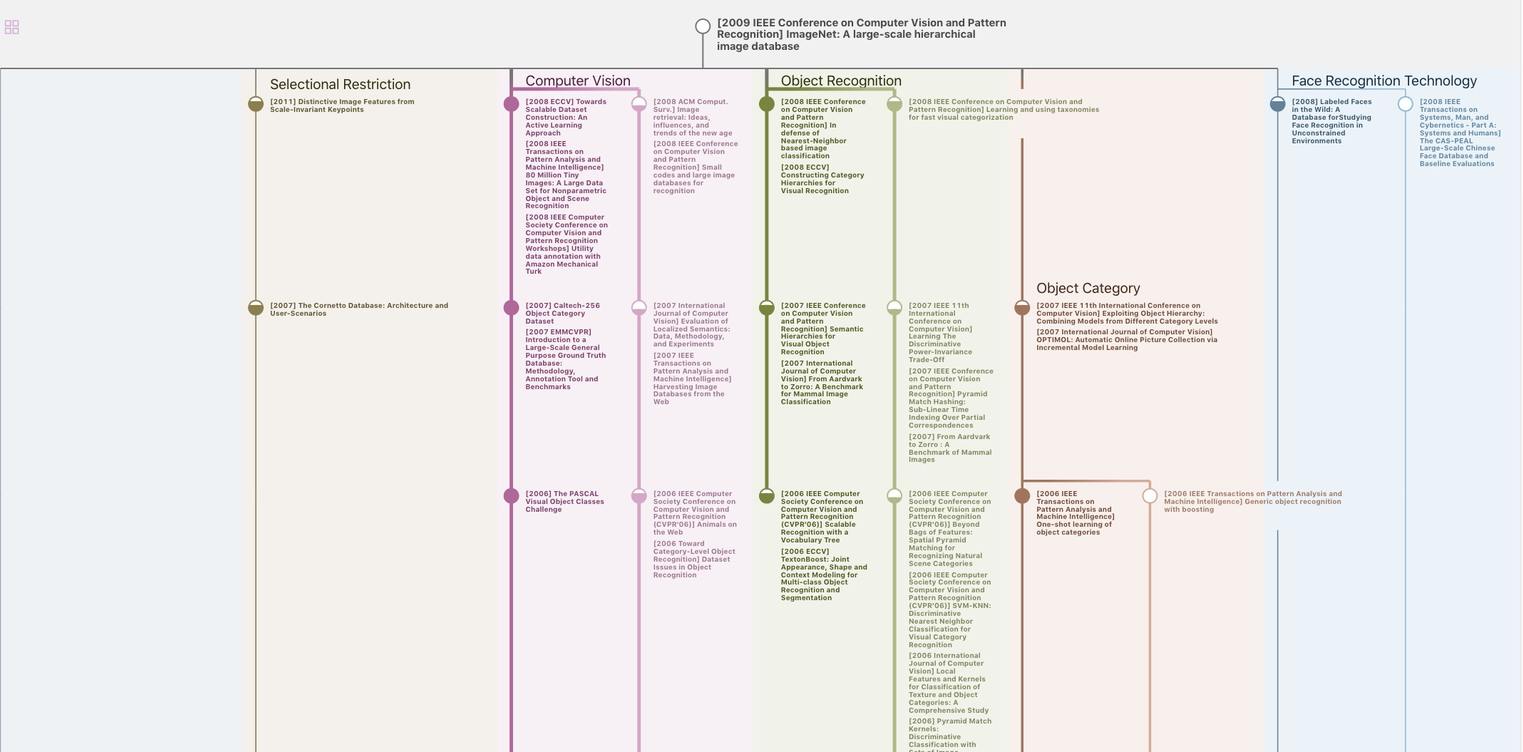
生成溯源树,研究论文发展脉络
Chat Paper
正在生成论文摘要