Local Causal Discovery in Multiple Manipulated Datasets
IEEE transactions on neural networks and learning systems(2023)
摘要
We consider the problem of distinguishing direct causes from direct effects of a target variable of interest from multiple manipulated datasets with unknown manipulated variables and nonidentical data distributions. Recent studies have shown that datasets attained from manipulated experiments (i.e., manipulated data) contain richer causal information than observational data for causal structure learning. Thus, in this article, we propose a new algorithm, which makes full use of the interventional properties of a causal model to discover the direct causes and direct effects of a target variable from multiple datasets with different manipulations. It is more suited to real-world cases and is also a challenge to be addressed in this article. First, we apply the backward framework to learn parents and children (PC) of a given target from multiple manipulated datasets. Second, we orient some edges connected to the target in advance through the assumption that the target variable is not manipulated and then orient the remaining undirected edges by finding invariant V-structures from multiple datasets. Third, we analyze the correctness of the proposed algorithm. To the best of our knowledge, the proposed algorithm is the first that can identify the local causal structure of a given target from multiple manipulated datasets with unknown manipulated variables. Experimental results on standard Bayesian networks validate the effectiveness of our algorithm.
更多查看译文
关键词
Markov processes,Inference algorithms,Skeleton,Learning systems,Bayes methods,Standards,Research and development,Bayesian network,local causal structure learning,Markov equivalence class,multiple manipulated datasets
AI 理解论文
溯源树
样例
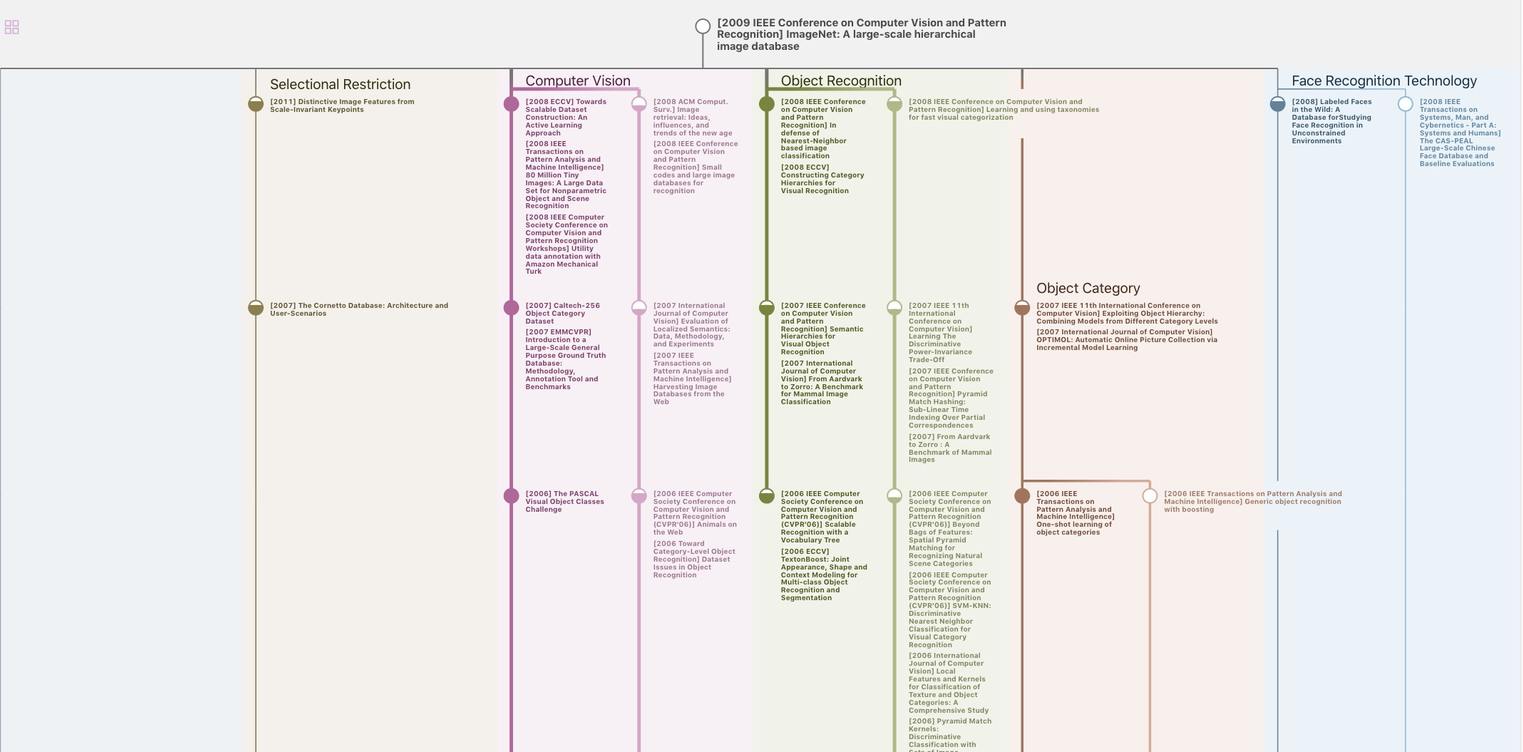
生成溯源树,研究论文发展脉络
Chat Paper
正在生成论文摘要