Development of a resource-efficient FPGA-based neural network regression model for the ATLAS muon trigger upgrades
The European Physical Journal C(2022)
摘要
This paper reports on the development of a resource-efficient FPGA-based neural network regression model for potential applications in the future hardware muon trigger system of the ATLAS experiment at the Large Hadron Collider (LHC). Effective real-time selection of muon candidates is the cornerstone of the ATLAS physics programme. With the planned ATLAS upgrades for the High Luminosity LHC, an entirely new FPGA-based hardware muon trigger system will be installed that will process full muon detector data within a 10 s latency window. The large FPGA devices planned for this upgrade should have sufficient spare resources to allow deployment of machine learning methods for improving identification of muon candidates and searching for new exotic particles. Our neural network regression model promises to improve rejection of the dominant source of background trigger events in the central detector region, which are due to muon candidates with low transverse momenta. This model was implemented in FPGA using 157 digital signal processors and about 5000 lookup tables. The simulated network latency and deadtime are 122 and 25 ns, respectively, when implemented in the FPGA device using a 320 MHz clock frequency. Two other FPGA implementations were also developed to study the impact of design choices on resource utilisation and latency. The performance parameters of our FPGA implementation are well within the requirements of the future muon trigger system, therefore opening a possibility for deploying machine learning methods for future data taking by the ATLAS experiment.
更多查看译文
关键词
atlas muon trigger upgrades,neural network regression model,neural network,resource-efficient,fpga-based
AI 理解论文
溯源树
样例
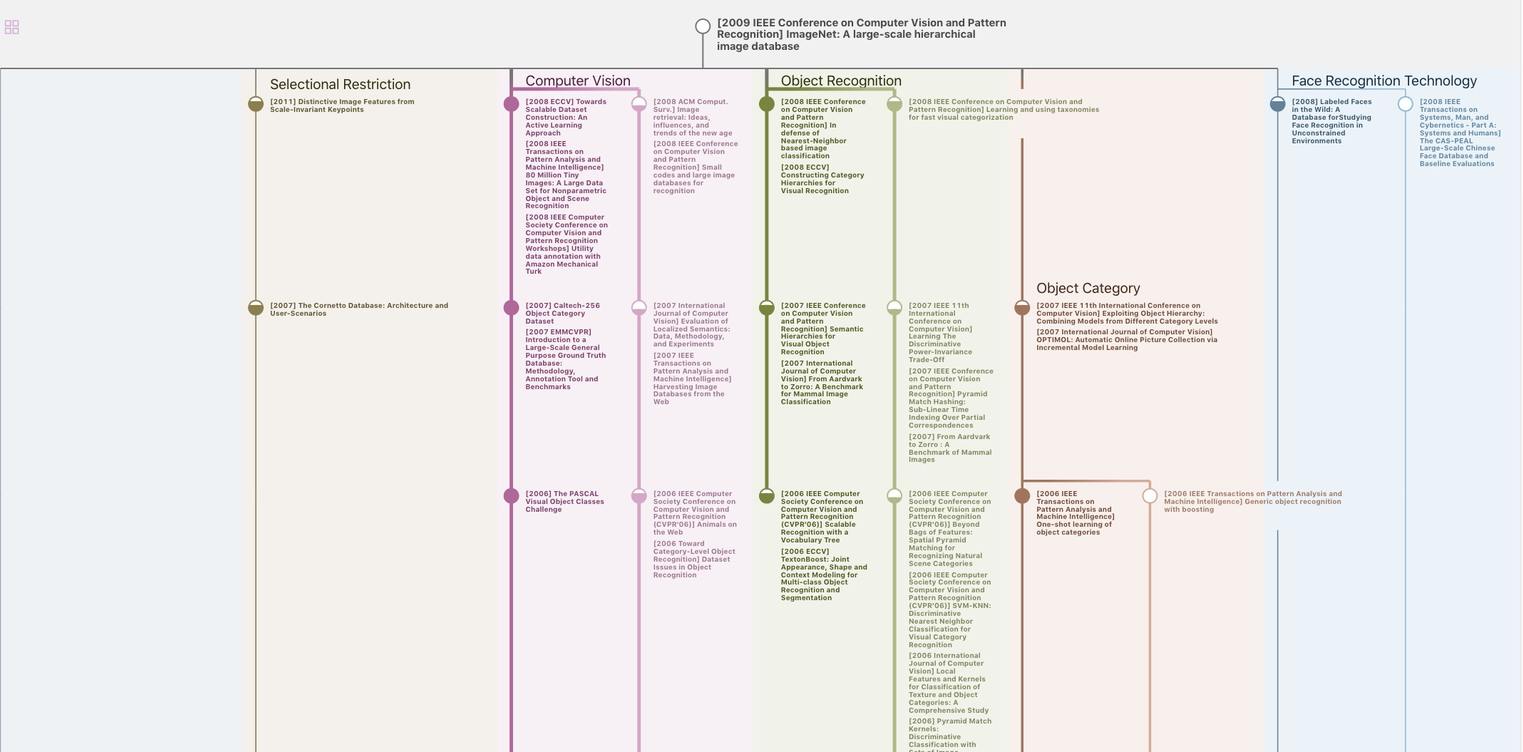
生成溯源树,研究论文发展脉络
Chat Paper
正在生成论文摘要