Transformers in Action:Weakly Supervised Action Segmentation
arxiv(2022)
摘要
The video action segmentation task is regularly explored under weaker forms of supervision, such as transcript supervision, where a list of actions is easier to obtain than dense frame-wise labels. In this formulation, the task presents various challenges for sequence modeling approaches due to the emphasis on action transition points, long sequence lengths, and frame contextualization, making the task well-posed for transformers. Given developments enabling transformers to scale linearly, we demonstrate through our architecture how they can be applied to improve action alignment accuracy over the equivalent RNN-based models with the attention mechanism focusing around salient action transition regions. Additionally, given the recent focus on inference-time transcript selection, we propose a supplemental transcript embedding approach to select transcripts more quickly at inference-time. Furthermore, we subsequently demonstrate how this approach can also improve the overall segmentation performance. Finally, we evaluate our proposed methods across the benchmark datasets to better understand the applicability of transformers and the importance of transcript selection on this video-driven weakly-supervised task.
更多查看译文
关键词
action,weakly
AI 理解论文
溯源树
样例
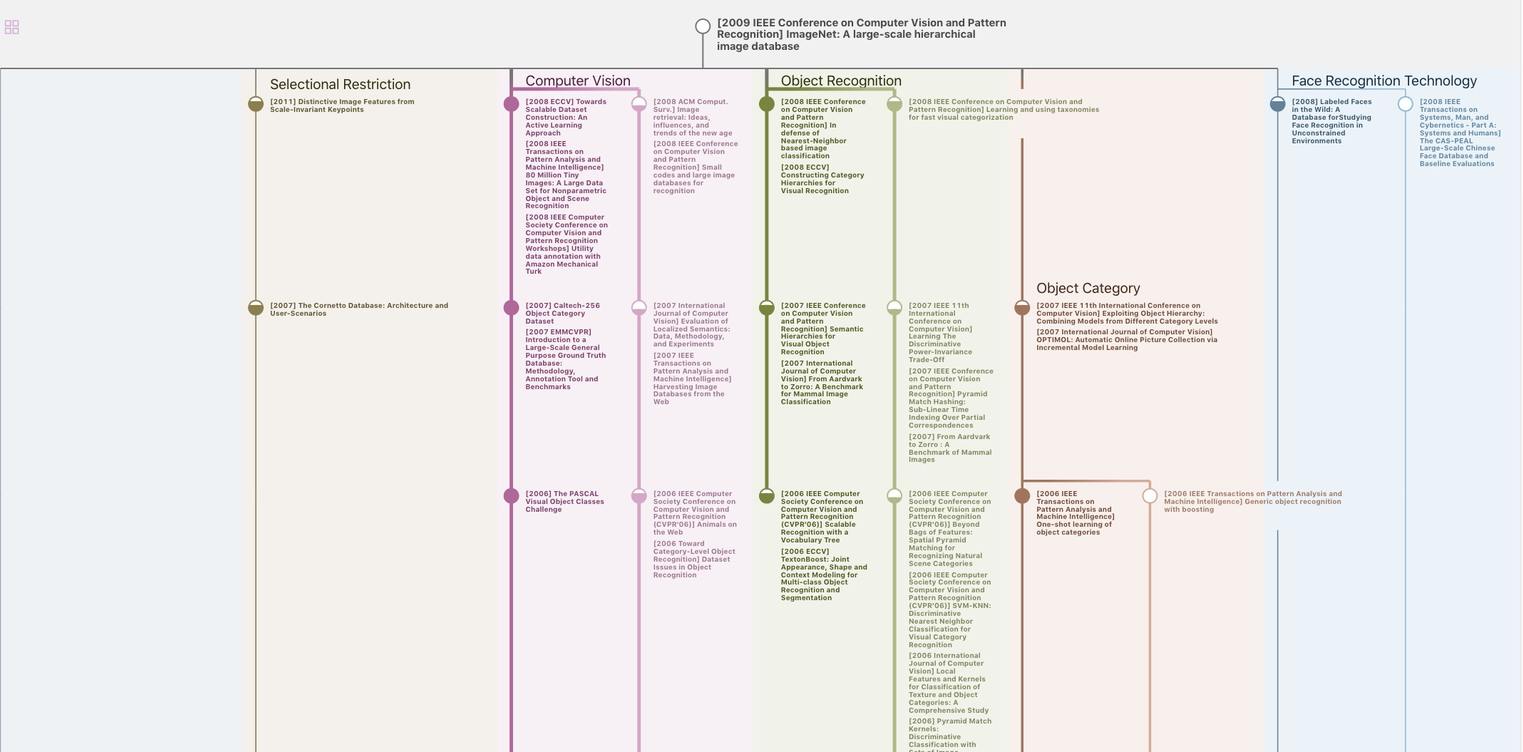
生成溯源树,研究论文发展脉络
Chat Paper
正在生成论文摘要