Sequence-to-Sequence Models for Extracting Information from Registration and Legal Documents
DOCUMENT ANALYSIS SYSTEMS, DAS 2022(2022)
Abstract
A typical information extraction pipeline consists of token- or span-level classification models coupled with a series of pre- and post-processing scripts. In a production pipeline, requirements often change, with classes being added and removed, which leads to nontrivial modifications to the source code and the possible introduction of bugs. In this work, we evaluate sequence-to-sequence models as an alternative to token-level classification methods for information extraction of legal and registration documents. We finetune models that jointly extract the information and generate the output already in a structured format. Post-processing steps are learned during training, thus eliminating the need for rule-based methods and simplifying the pipeline. Furthermore, we propose a novel method to align the output with the input text, thus facilitating system inspection and auditing. Our experiments on four real-world datasets show that the proposed method is an alternative to classical pipelines. The source code is available at https://github.com/neuralmind-ai/information-extraction-t5.
MoreTranslated text
Key words
Information extraction, Sequence-to-sequence, Legal texts
AI Read Science
Must-Reading Tree
Example
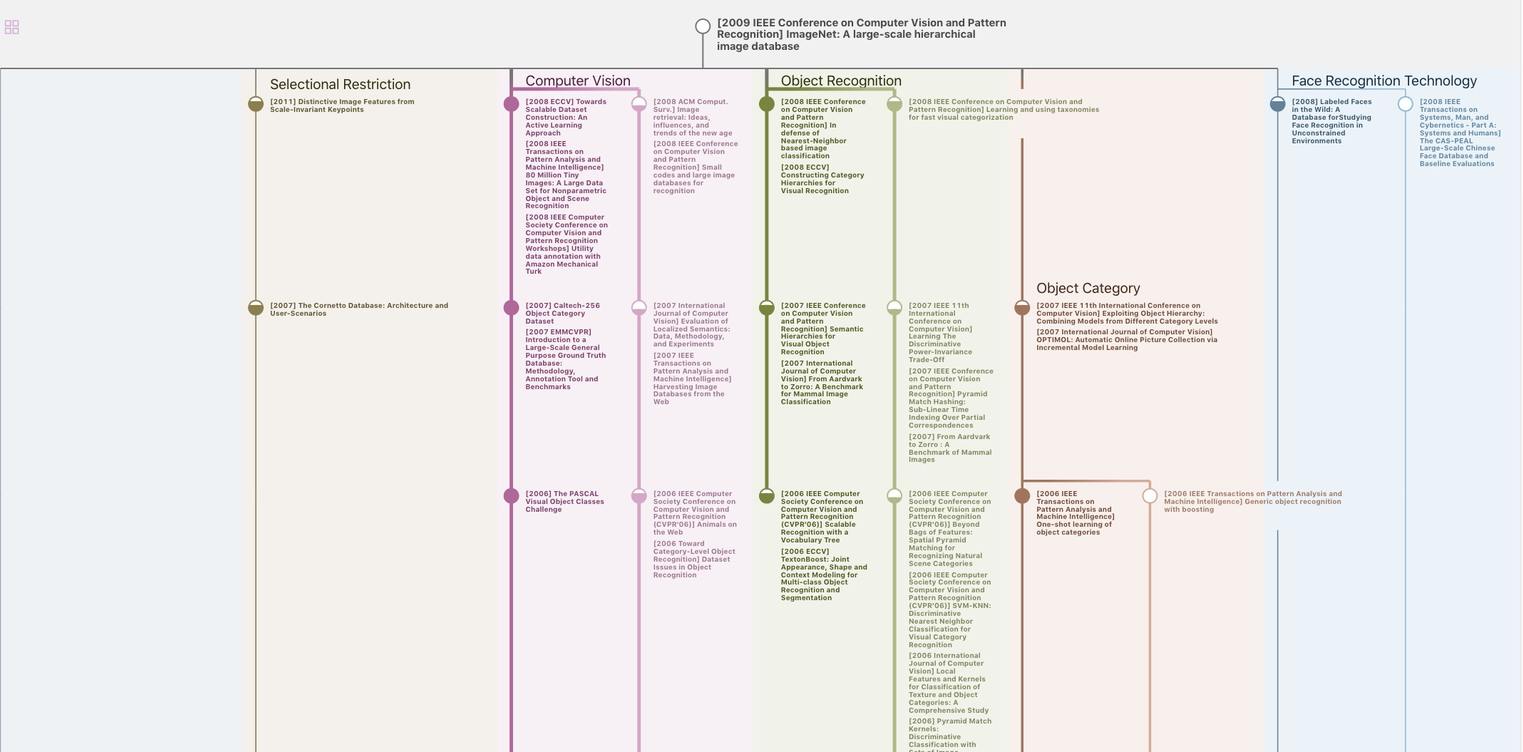
Generate MRT to find the research sequence of this paper
Chat Paper
Summary is being generated by the instructions you defined