STEdge: Self-Training Edge Detection With Multilayer Teaching and Regularization.
arXiv (Cornell University)(2023)
摘要
Learning-based edge detection has hereunto been strongly supervised with pixel-wise annotations which are tedious to obtain manually. We study the problem of self-training edge detection, leveraging the untapped wealth of large-scale unlabeled image datasets. We design a self-supervised framework with multilayer regularization and self-teaching. In particular, we impose a consistency regularization which enforces the outputs from each of the multiple layers to be consistent for the input image and its perturbed counterpart. We adopt L0-smoothing as the "perturbation" to encourage edge prediction lying on salient boundaries following the cluster assumption in self-supervised learning. Meanwhile, the network is trained with multilayer supervision by pseudo labels which are initialized with Canny edges and then iteratively refined by the network as the training proceeds. The regularization and self-teaching together attain a good balance of precision and recall, leading to a significant performance boost over supervised methods, with lightweight refinement on the target dataset. Through extensive experiments, our method demonstrates strong cross-dataset generality and can improve the original performance of edge detectors after self-training and fine-tuning.
更多查看译文
关键词
Consistency regularization,edge detection,pseudo labels,self-training
AI 理解论文
溯源树
样例
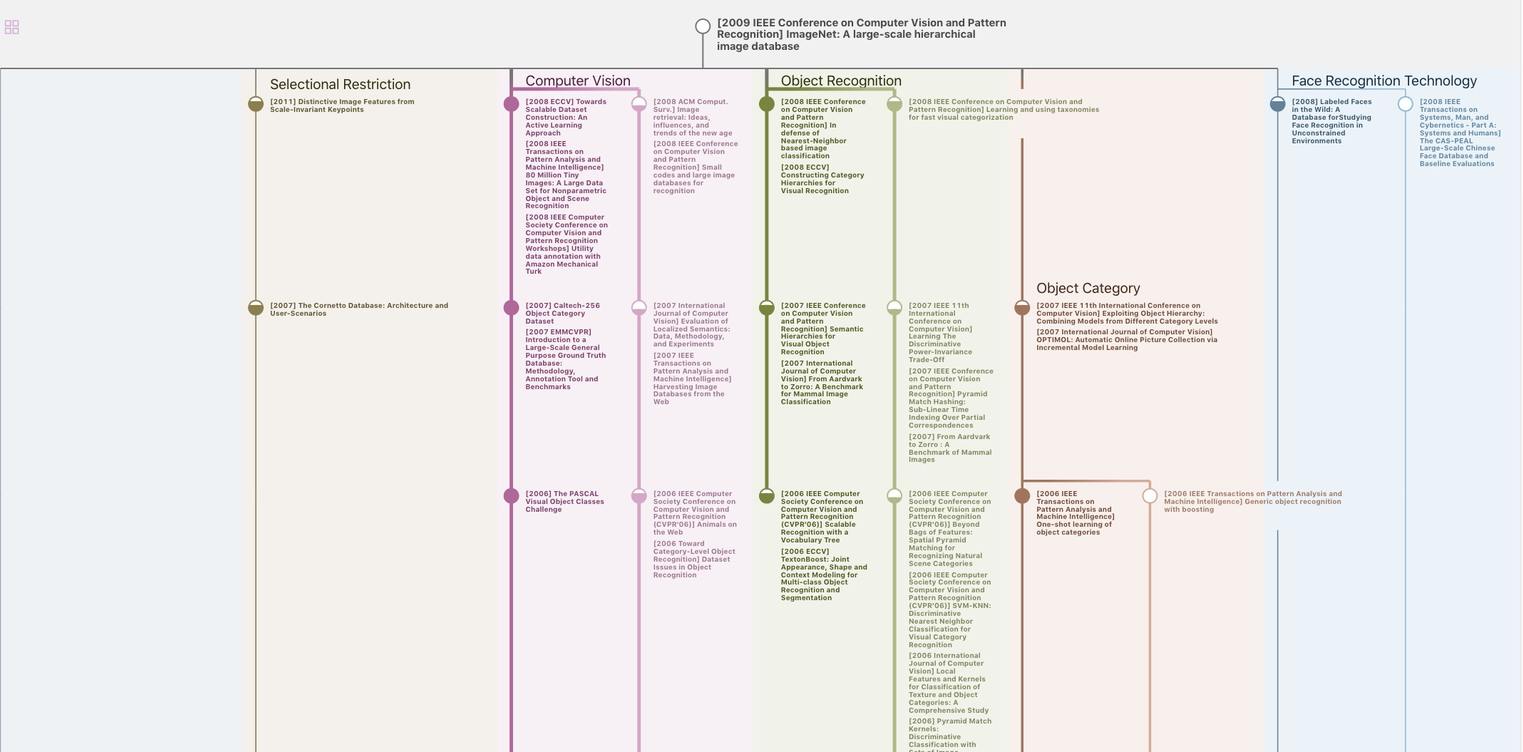
生成溯源树,研究论文发展脉络
Chat Paper
正在生成论文摘要