Spatiotemporal Clustering with Neyman-Scott Processes via Connections to Bayesian Nonparametric Mixture Models
JOURNAL OF THE AMERICAN STATISTICAL ASSOCIATION(2023)
摘要
Neyman-Scott processes (NSPs) are point process models that generate clusters of points in time or space. They are natural models for a wide range of phenomena, ranging from neural spike trains to document streams. The clustering property is achieved via a doubly stochastic formulation: first, a set of latent events is drawn from a Poisson process; then, each latent event generates a set of observed data points according to another Poisson process. This construction is similar to Bayesian nonparametric mixture models like the Dirichlet process mixture model (DPMM) in that the number of latent events (i.e., clusters) is a random variable, but the point process formulation makes the NSP especially well suited to modeling spatiotemporal data. While many specialized algorithms have been developed for DPMMs, comparatively fewer works have focused on inference in NSPs. Here, we present novel connections between NSPs and DPMMs, with the key link being a third class of Bayesian mixture models called mixture of finite mixture models (MFMMs). Leveraging this connection, we adapt the standard collapsed Gibbs sampling algorithm for DPMMs to enable scalable Bayesian inference on NSP models. We demonstrate the potential of Neyman-Scott processes on a variety of applications including sequence detection in neural spike trains and event detection in document streams. Supplementary materials for this article are available online.
更多查看译文
关键词
Collapsed Gibbs,Dirichlet process,Mixture of finite mixture model,Neyman-Scott process
AI 理解论文
溯源树
样例
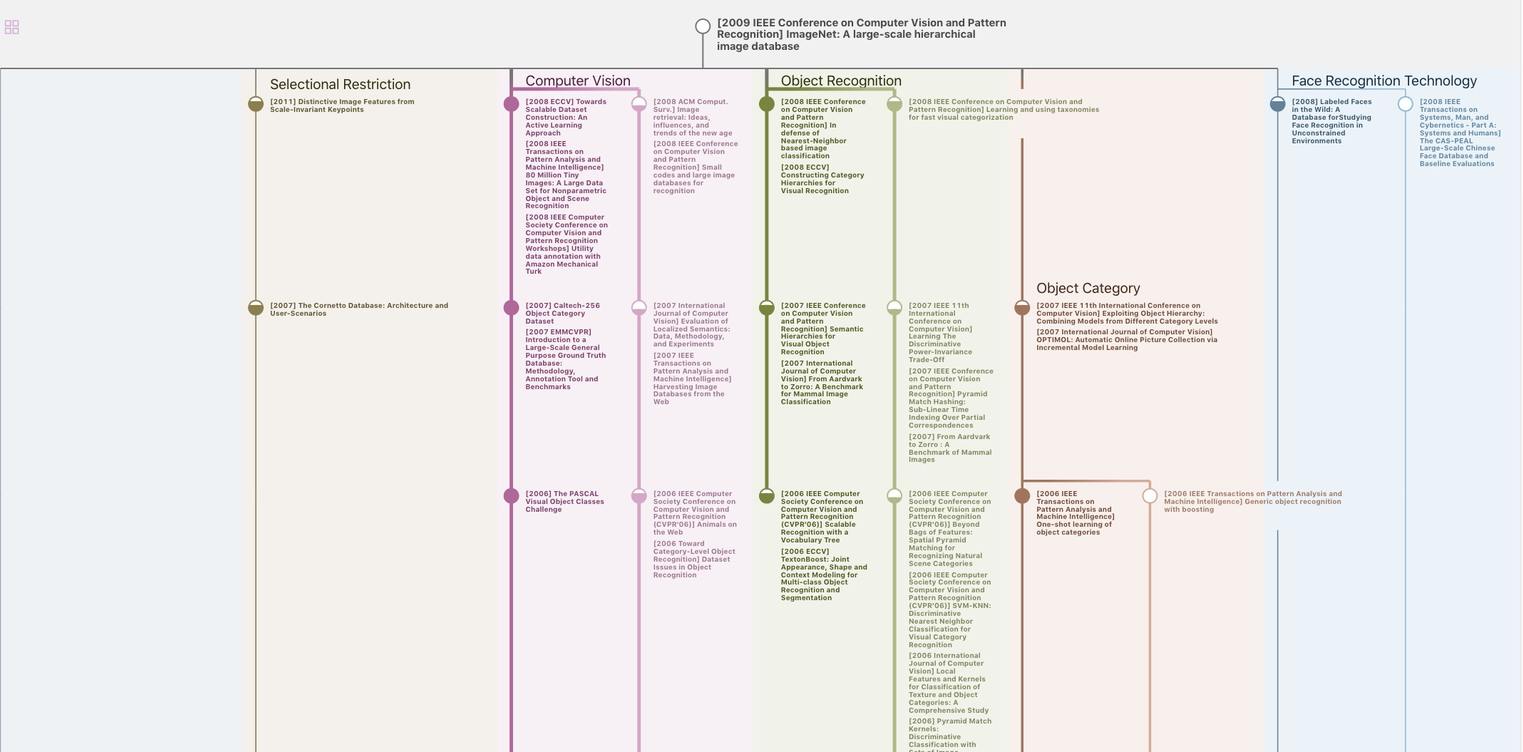
生成溯源树,研究论文发展脉络
Chat Paper
正在生成论文摘要