Physics-informed deep learning for solving phonon Boltzmann transport equation with large temperature non-equilibrium
NPJ COMPUTATIONAL MATERIALS(2022)
摘要
Phonon Boltzmann transport equation (BTE) is a key tool for modeling multiscale phonon transport, which is critical to the thermal management of miniaturized integrated circuits, but assumptions about the system temperatures (i.e., small temperature gradients) are usually made to ensure that it is computationally tractable. To include the effects of large temperature non-equilibrium, we demonstrate a data-free deep learning scheme, physics-informed neural network (PINN), for solving stationary, mode-resolved phonon BTE with arbitrary temperature gradients. This scheme uses the temperature-dependent phonon relaxation times and learns the solutions in parameterized spaces with both length scale and temperature gradient treated as input variables. Numerical experiments suggest that the proposed PINN can accurately predict phonon transport (from 1D to 3D) under arbitrary temperature gradients. Moreover, the proposed scheme shows great promise in simulating device-level phonon heat conduction efficiently and can be potentially used for thermal design.
更多查看译文
关键词
phonon boltzmann transport
AI 理解论文
溯源树
样例
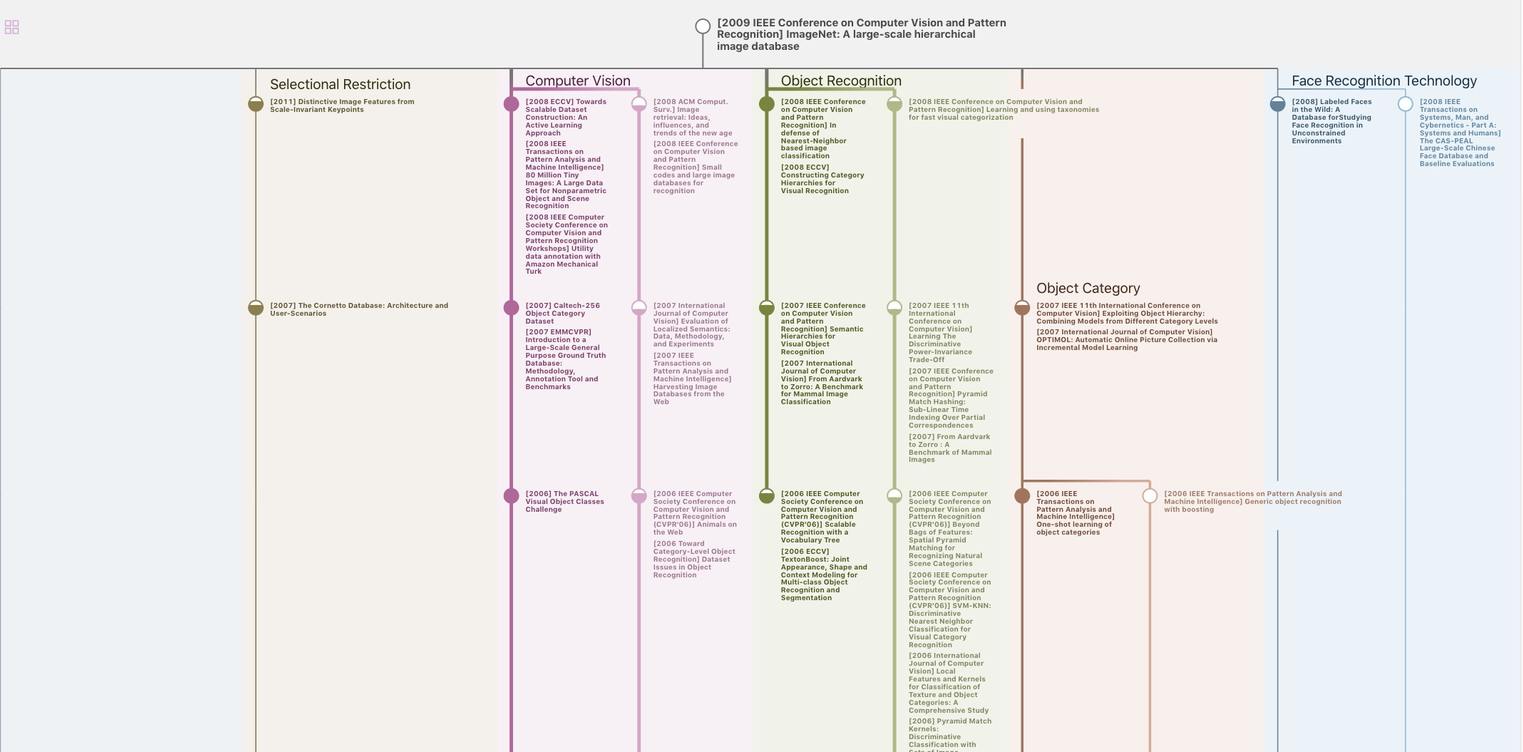
生成溯源树,研究论文发展脉络
Chat Paper
正在生成论文摘要