Deep Video Prior for Video Consistency and Propagation
IEEE Transactions on Pattern Analysis and Machine Intelligence(2023)
摘要
Applying an image processing algorithm independently to each video frame often leads to temporal inconsistency in the resulting video. To address this issue, we present a novel and general approach for blind video temporal consistency. Our method is only trained on a pair of original and processed videos directly instead of a large dataset. Unlike most previous methods that enforce temporal consistency with optical flow, we show that temporal consistency can be achieved by training a convolutional network on a video with Deep Video Prior (DVP). Moreover, a carefully designed iteratively reweighted training strategy is proposed to address the challenging multimodal inconsistency problem. We demonstrate the effectiveness of our approach on 7 computer vision tasks on videos. Extensive quantitative and perceptual experiments show that our approach obtains superior performance than state-of-the-art methods on blind video temporal consistency. We further extend DVP to video propagation and demonstrate its effectiveness in propagating three different types of information (color, artistic style, and object segmentation). A progressive propagation strategy with pseudo labels is also proposed to enhance DVP’s performance on video propagation. Our source codes are publicly available at
https://github.com/ChenyangLEI/deep-video-prior
.
更多查看译文
关键词
Temporal consistency,video processing,internal learning,video propagation,deep learning
AI 理解论文
溯源树
样例
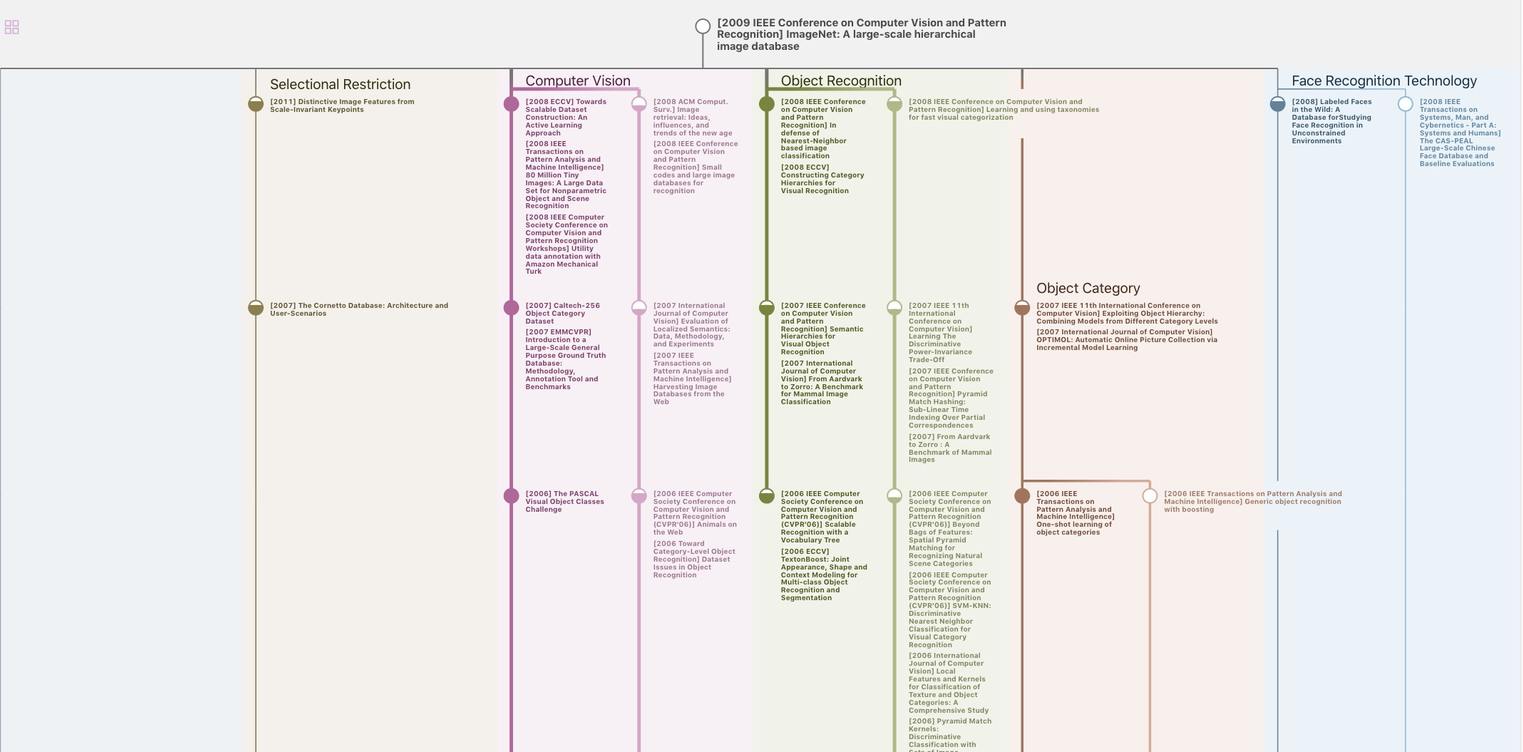
生成溯源树,研究论文发展脉络
Chat Paper
正在生成论文摘要