Fine-grained Graph Learning for Multi-view Subspace Clustering
arxiv(2022)
摘要
Multi-view subspace clustering (MSC) is a popular unsupervised method by integrating heterogeneous information to reveal the intrinsic clustering structure hidden across views. Usually, MSC methods use graphs (or affinity matrices) fusion to learn a common structure, and further apply graph-based approaches to clustering. Despite progress, most of the methods do not establish the connection between graph learning and clustering. Meanwhile, conventional graph fusion strategies assign coarse-grained weights to combine multi-graph, ignoring the importance of local structure. In this paper, we propose a fine-grained graph learning framework for multi-view subspace clustering (FGL-MSC) to address these issues. To utilize the multi-view information sufficiently, we design a specific graph learning method by introducing graph regularization and local structure fusion pattern. The main challenge is how to optimize the fine-grained fusion weights while generating the learned graph that fits the clustering task, thus making the clustering representation meaningful and competitive. Accordingly, an iterative algorithm is proposed to solve the above joint optimization problem, which obtains the learned graph, the clustering representation, and the fusion weights simultaneously. Extensive experiments on eight real-world datasets show that the proposed framework has comparable performance to the state-of-the-art methods.
更多查看译文
关键词
Multi-view learning,subspace clustering,graph learning,joint optimization
AI 理解论文
溯源树
样例
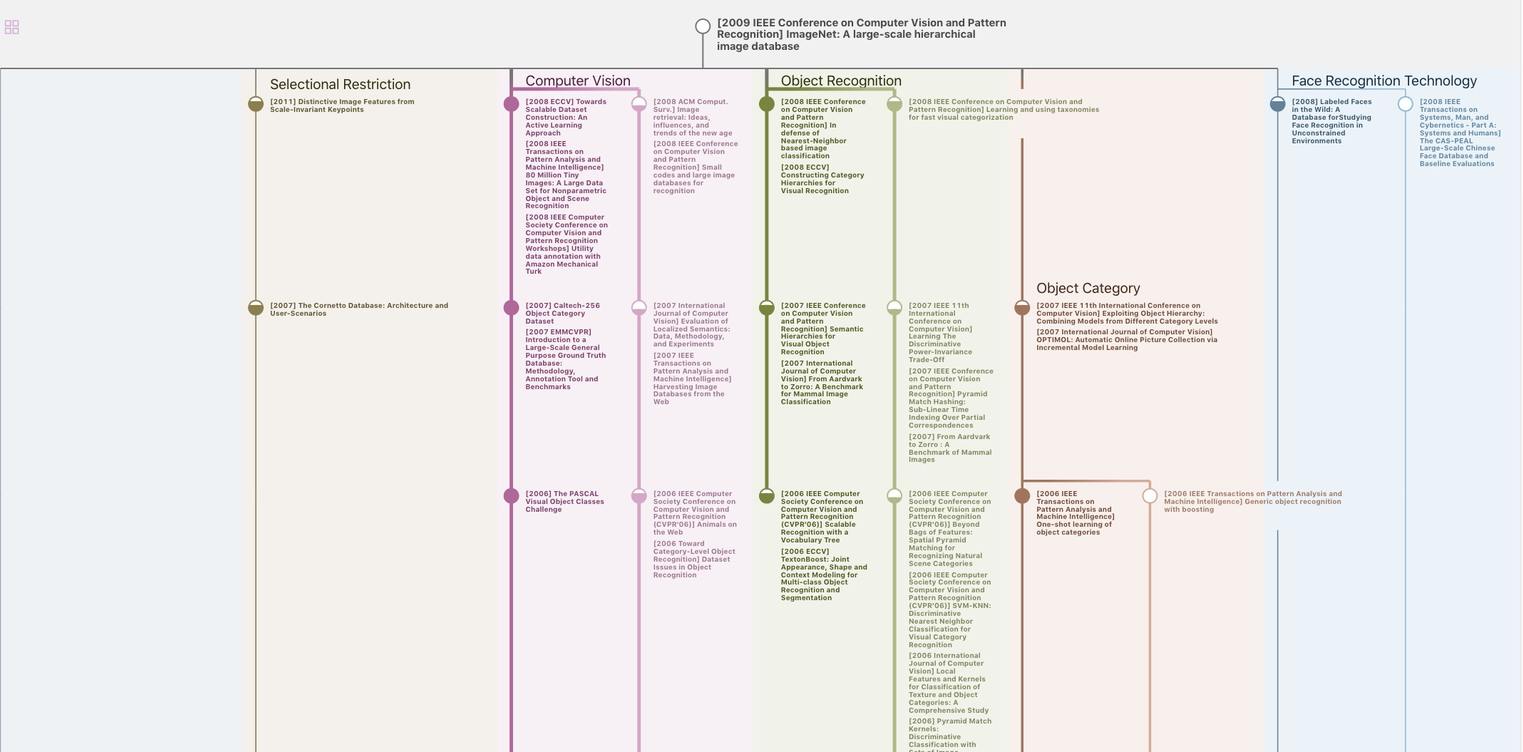
生成溯源树,研究论文发展脉络
Chat Paper
正在生成论文摘要