Cluster formation in particle-laden flows is a continuous phase transition
arxiv(2022)
摘要
Studying particle-laden flows is essential to understand diverse physical processes such as rain formation in clouds, pathogen transmission, and pollutant dispersal. Distinct clustering patterns are formed in such flows with particles of different inertia (characterized by Stokes number St). For the first time, we use complex networks to study the spatiotemporal dynamics in such flows. We simulate particles in a 2D Taylor-Green flow and show that the network measures characterize both the local and global clustering properties. As particles cluster into specific patterns from a randomly distributed initial condition, we observe an emergence of a giant component in the derived network through a continuous phase transition. Further, the phase transition time is identified to be related to the Stokes number through a power law for St < 0.25 and an exponential function for St in the range 0.25 to 1. Our findings provide novel insights into the clustering phenomena in particle-laden flows.
更多查看译文
关键词
phase
AI 理解论文
溯源树
样例
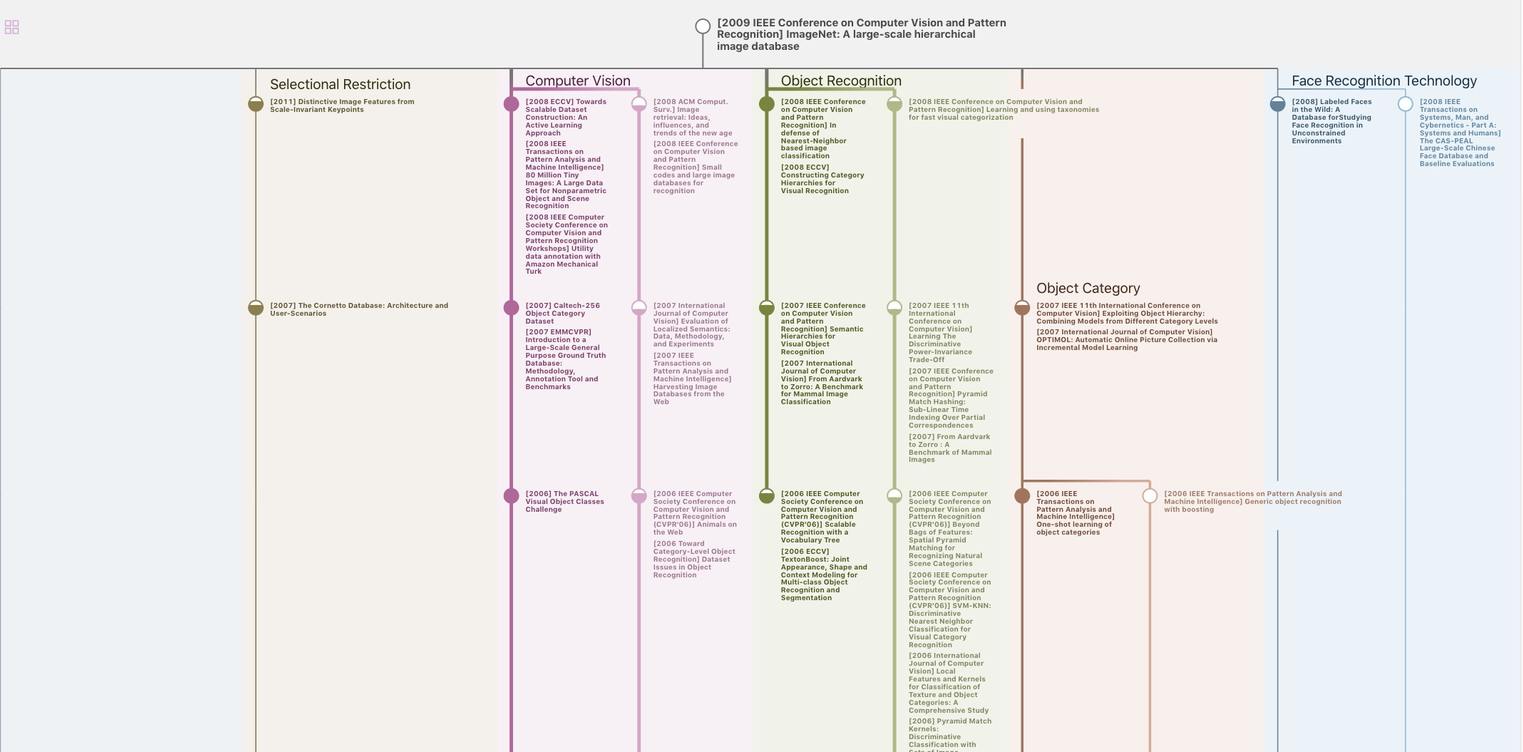
生成溯源树,研究论文发展脉络
Chat Paper
正在生成论文摘要