Combined strategy of knowledge-based rule selection and historical data percentile-based range determination to improve an autoverification system for clinical chemistry test results
JOURNAL OF CLINICAL LABORATORY ANALYSIS(2022)
摘要
Background Current autoverification, which is only knowledge-based, has low efficiency. Regular historical data analysis may improve autoverification range determination. We attempted to enhance autoverification by selecting autoverification rules by knowledge and ranges from historical data. This new system was compared with the original knowledge-based system. Methods New types of rules, extreme values, and consistency checks were added and the autoverification workflow was rearranged to construct a framework. Criteria for creating rules for extreme value ranges, limit checks, consistency checks, and delta checks were determined by analyzing historical Zhongshan laboratory data. The new system's effectiveness was evaluated using pooled data from 20 centers. Efficiency improvement was assessed by a multicenter process. Results Effectiveness was evaluated by the true positive rate, true negative rate, and overall consistency rate, as compared to manual verification, which were 77.55%, 78.53%, and 78.3%, respectively for the new system. The original overall consistency rate was 56.2%. The new pass rates, indicating efficiency, were increased by 19%-51% among hospitals. Further customization using individualized data increased this rate. Conclusions The improved system showed a comparable effectiveness and markedly increased efficiency. This transferable system could be further improved and popularized by utilizing historical data from each hospital.
更多查看译文
关键词
autoverification system, clinical chemistry test report, efficiency, historical data percentile-based, knowledge-based
AI 理解论文
溯源树
样例
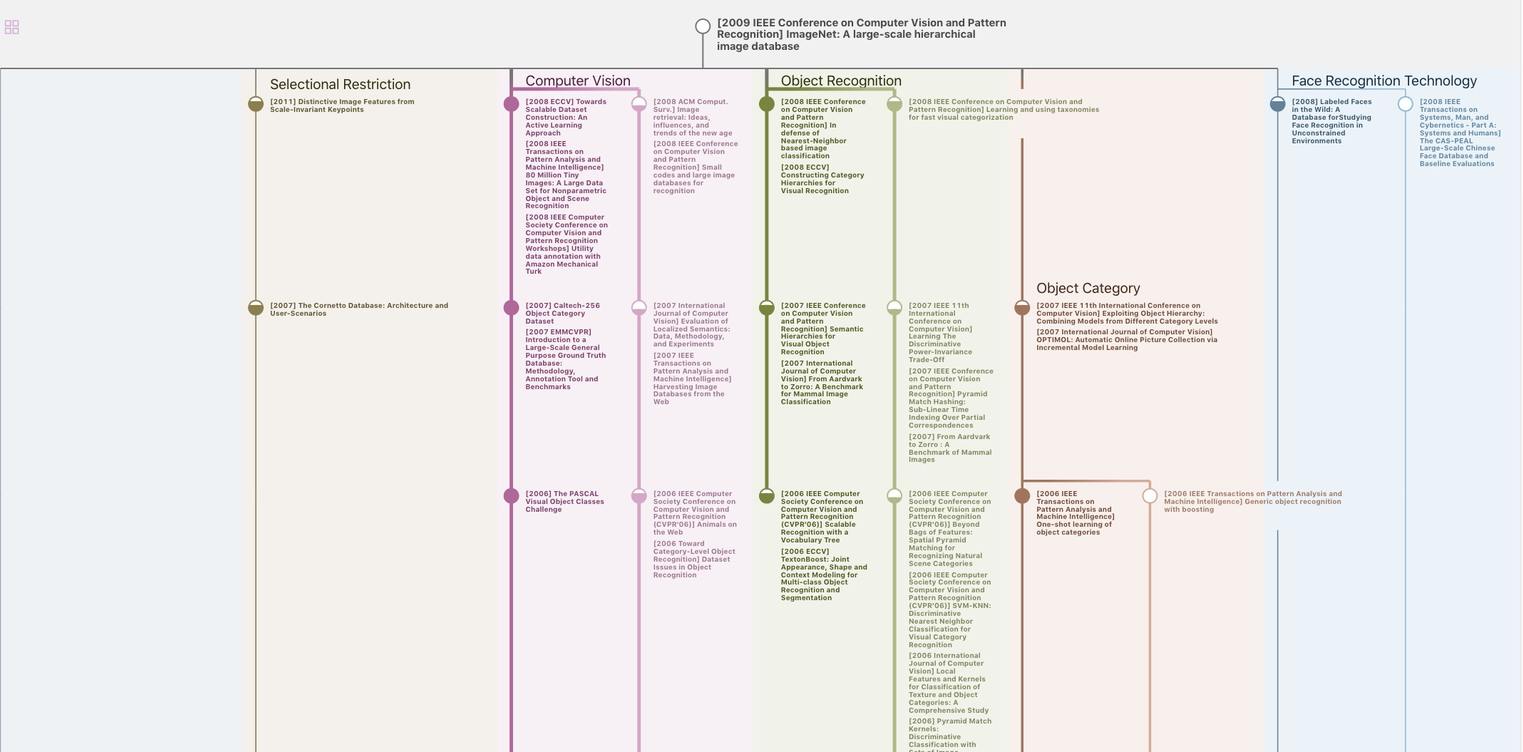
生成溯源树,研究论文发展脉络
Chat Paper
正在生成论文摘要