Multidimensional nonadditivity in one-facet g-theory designs: A profile analytic approach.
Psychological methods(2022)
摘要
We introduce a new method for estimating the degree of nonadditivity in a one-facet generalizability theory design. One-facet G-theory designs have only one observation per cell, such as persons answering items in a test, and assume that there is no interaction between facets. When there is interaction, the model becomes nonadditive, and G-theory variance estimates and reliability coefficients are likely biased. We introduce a multidimensional method for detecting interaction and nonadditivity in G-theory that has less bias and smaller error variance than methods that use the one-degree of freedom method based on Tukey's test for nonadditivity. The method we propose is more flexible and detects a greater variety of interactions than the formulation based on Tukey's test. Further, the proposed method is descriptive and illustrates the nature of the facet interaction using profile analysis, giving insight into potential interaction like rater biases, DIF, threats to test security, and other possible sources of systematic construct-irrelevant variance. We demonstrate the accuracy of our method using a simulation study and illustrate its descriptive profile features with a real data analysis of neurocognitive test scores. (PsycInfo Database Record (c) 2022 APA, all rights reserved).
更多查看译文
关键词
generalizability theory,nonadditivity,ANOVA,reliability,profile analysis
AI 理解论文
溯源树
样例
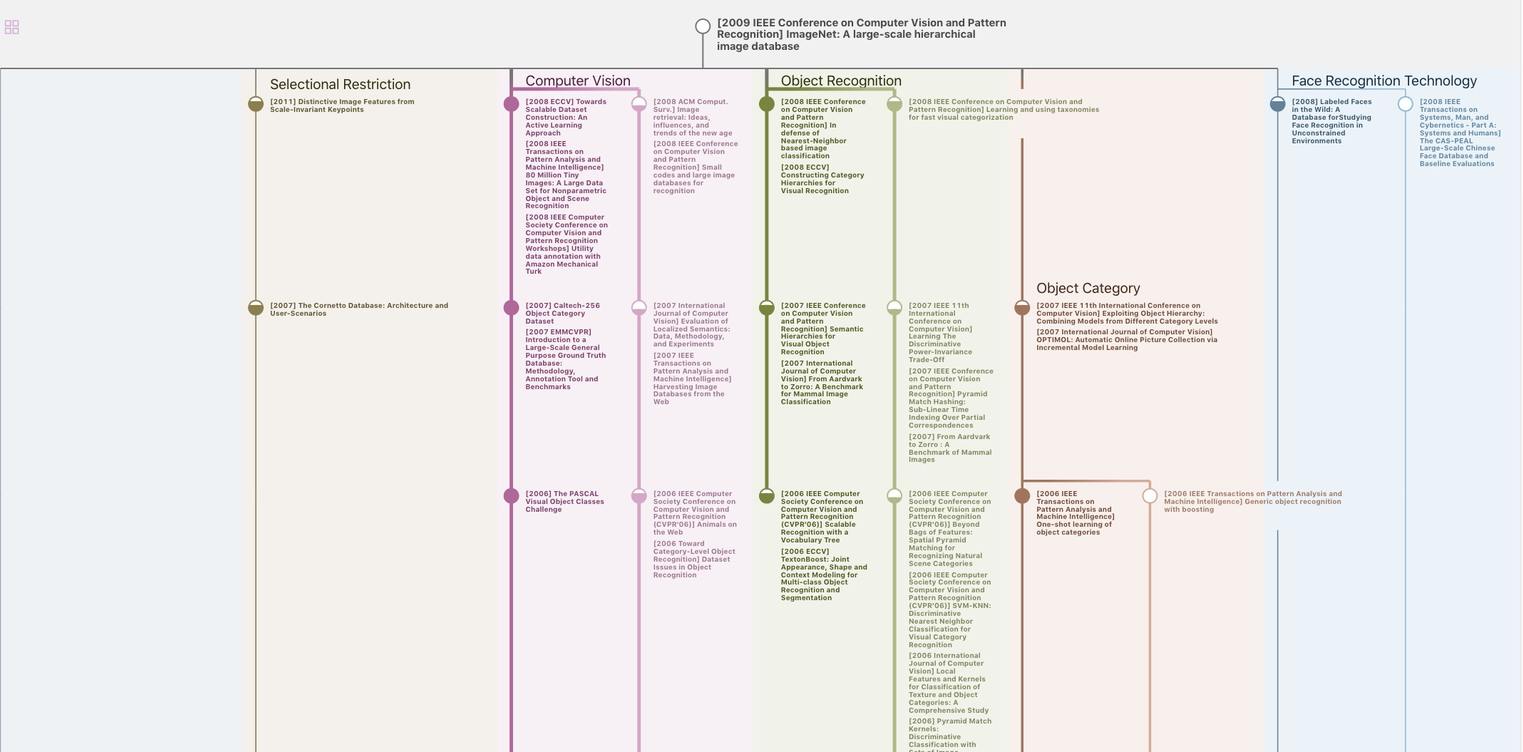
生成溯源树,研究论文发展脉络
Chat Paper
正在生成论文摘要