Vision in adverse weather: Augmentation using CycleGANs with various object detectors for robust perception in autonomous racing
arxiv(2023)
摘要
In an autonomous driving system, perception - identification of features and objects from the environment - is crucial. In autonomous racing, high speeds and small margins demand rapid and accurate detection systems. During the race, the weather can change abruptly, causing significant degradation in perception, resulting in ineffective manoeuvres. In order to improve detection in adverse weather, deep-learning-based models typically require extensive datasets captured in such conditions - the collection of which is a tedious, laborious, and costly process. However, recent developments in CycleGAN architectures allow the synthesis of highly realistic scenes in multiple weather conditions. To this end, we introduce an approach of using synthesised adverse condition datasets in autonomous racing (generated using CycleGAN) to improve the performance of four out of five state-of-the-art detectors by an average of 42.7 and 4.4 mAP percentage points in the presence of night-time conditions and droplets, respectively. Furthermore, we present a comparative analysis of five object detectors - identifying the optimal pairing of detector and training data for use during autonomous racing in challenging conditions.
更多查看译文
关键词
object detectors,cyclegans,robust perception,vision
AI 理解论文
溯源树
样例
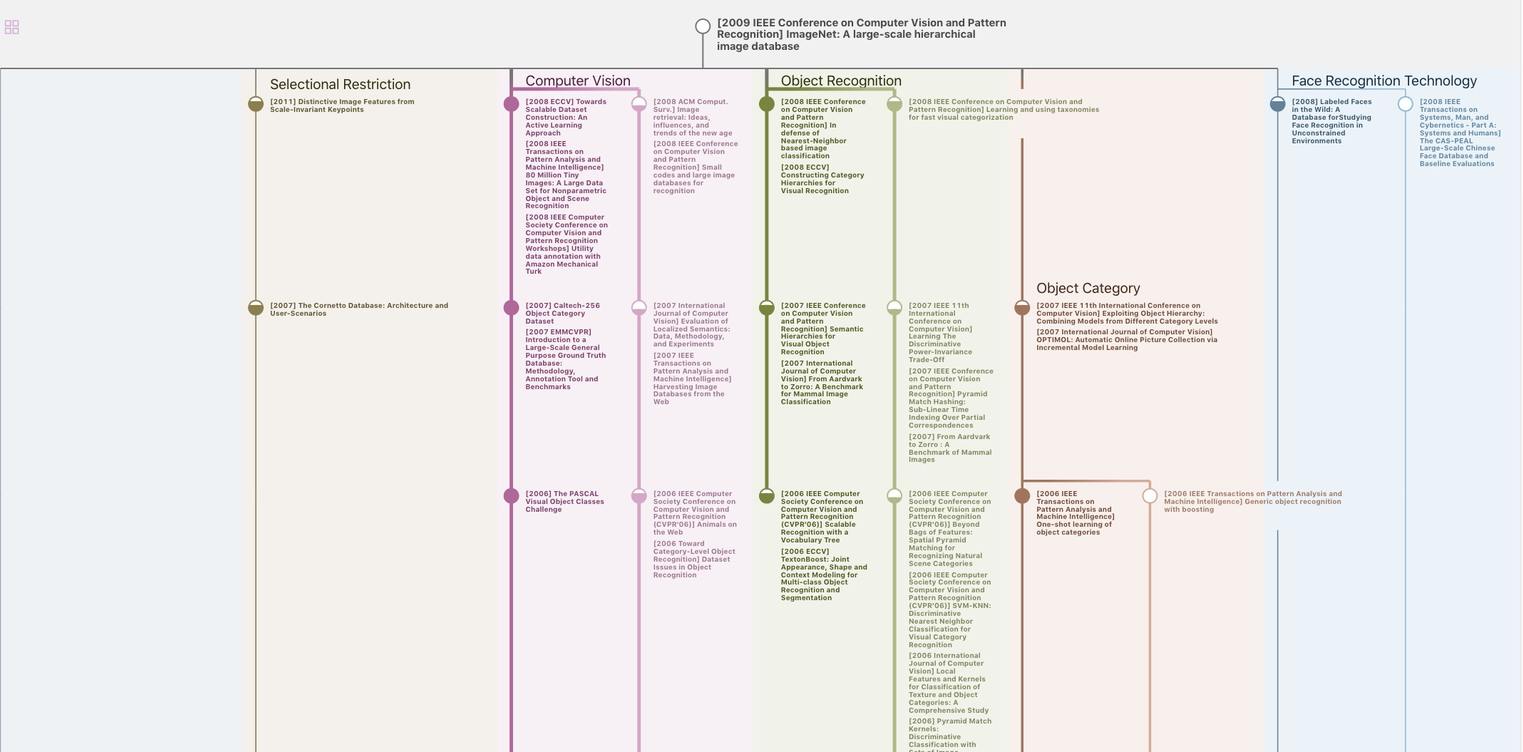
生成溯源树,研究论文发展脉络
Chat Paper
正在生成论文摘要