LoMar: A Local Defense Against Poisoning Attack on Federated Learning
IEEE Transactions on Dependable and Secure Computing(2023)
Abstract
learning (FL) provides a high efficient decentralized machine learning framework, where the training data remains distributed at remote clients in a network. Though FL enables a privacy-preserving mobile edge computing framework using IoT devices, recent studies have shown that this approach is susceptible to poisoning attacks from the side of remote clients. To address the poisoning attacks on FL, we provide a two-phase defense algorithm called Local Malicious Factor (LoMar). In phase I, LoMar scores model updates from each remote client by measuring the relative distribution over their neighbors using a kernel density estimation method. In phase II, an optimal threshold is approximated to distinguish malicious and clean updates from a statistical perspective. Comprehensive experiments on four real-world datasets have been conducted, and the experimental results show that our defense strategy can effectively protect the FL system. Specifically, the defense performance on Amazon dataset under a label-flipping attack indicates that, compared with FG+Krum, LoMar increases the target label testing accuracy from 96.0% to 98.8%, and the overall averaged testing accuracy from 90.1% to 97.0%.
MoreTranslated text
Key words
Distributed artificial intelligence,security and protection,defense,distribution functions,distributed architectures
AI Read Science
Must-Reading Tree
Example
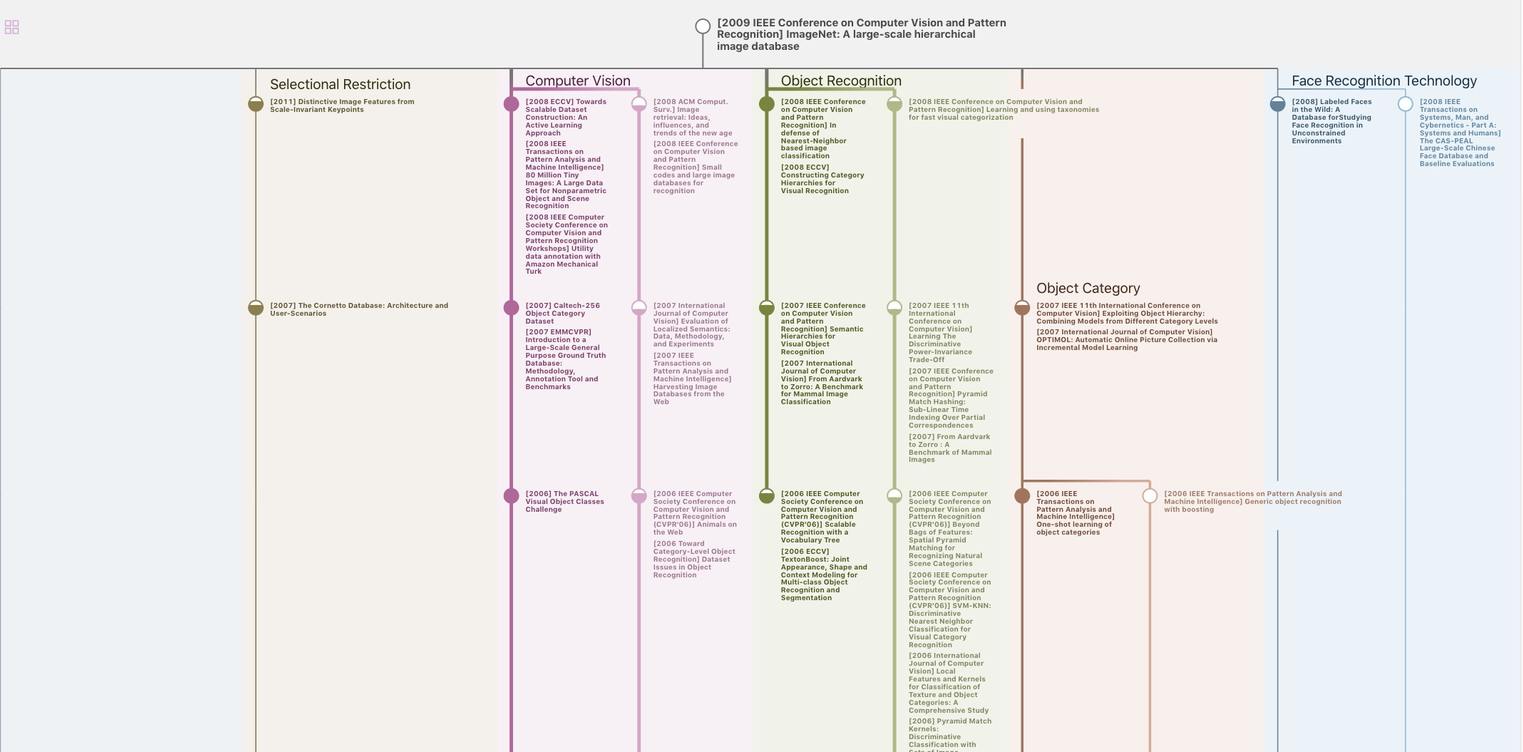
Generate MRT to find the research sequence of this paper
Chat Paper
Summary is being generated by the instructions you defined