Optimality in noisy importance sampling
Signal Processing(2022)
摘要
Many applications in signal processing and machine learning require the study of probability density functions (pdfs) that can only be accessed through noisy evaluations. In this work, we analyze the noisy importance sampling (IS), i.e., IS working with noisy evaluations of the target density. We present the general framework and derive optimal proposal densities for noisy IS estimators. The optimal proposals incorporate the information of the variance of the noisy realizations, proposing points in regions where the noise power is higher. We also compare the use of the optimal proposals with previous optimality approaches considered in a noisy IS framework.
更多查看译文
关键词
Bayesian Inference,Noisy Monte Carlo,Pseudo-marginal Metropolis-Hastings,Noisy IS
AI 理解论文
溯源树
样例
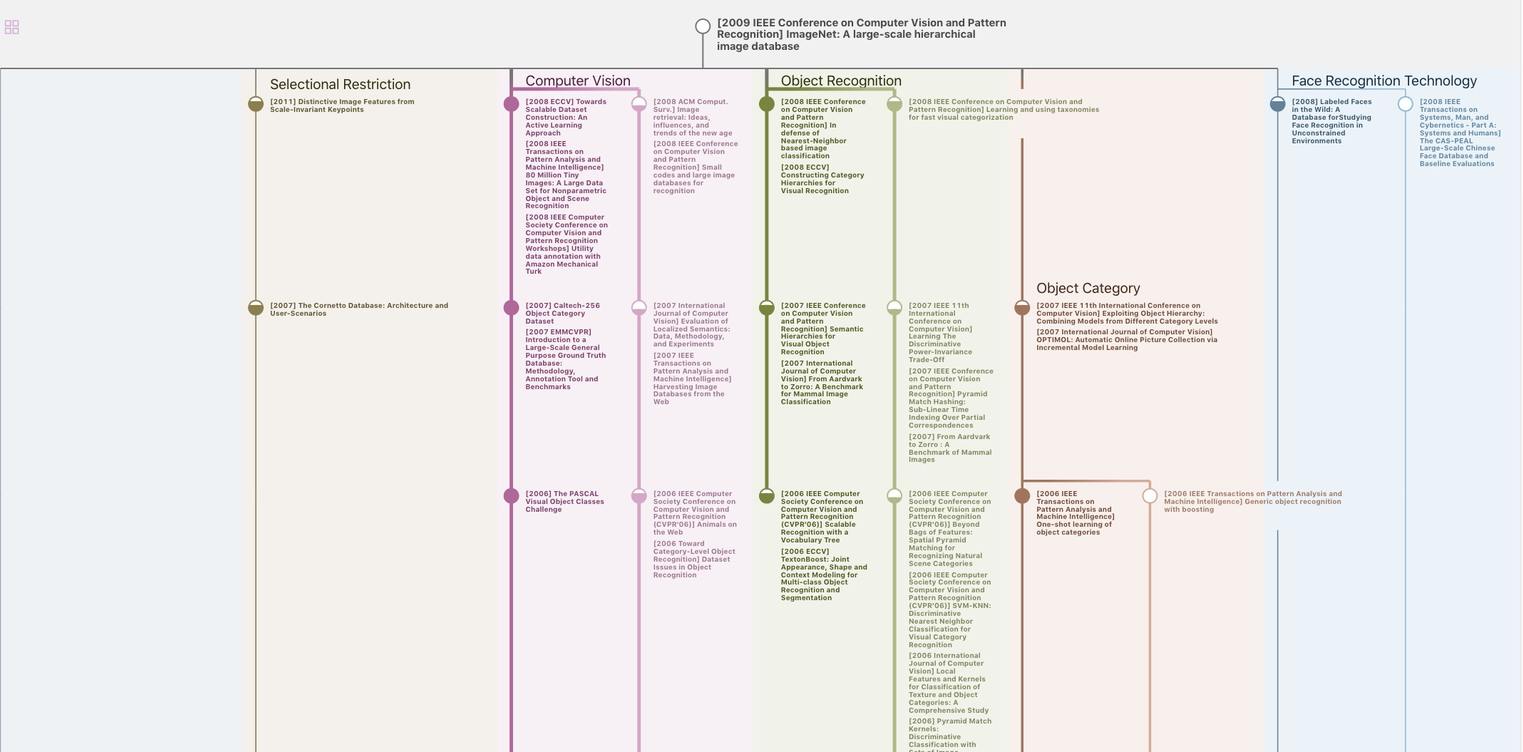
生成溯源树,研究论文发展脉络
Chat Paper
正在生成论文摘要