Auction-Based Ex-Post-Payment Incentive Mechanism Design for Horizontal Federated Learning with Reputation and Contribution Measurement
arxiv(2022)
摘要
Federated learning trains models across devices with distributed data, while protecting the privacy and obtaining a model similar to that of centralized ML. A large number of workers with data and computing power are the foundation of federal learning. However, the inevitable costs prevent self-interested workers from serving for free. Moreover, due to data isolation, task publishers lack effective methods to select, evaluate and pay reliable workers with high-quality data. Therefore, we design an auction-based incentive mechanism for horizontal federated learning with reputation and contribution measurement. By designing a reasonable method of measuring contribution, we establish the reputation of workers, which is easy to decline and difficult to improve. Through reverse auctions, workers bid for tasks, and the task publisher selects workers combining reputation and bid price. With the budget constraint, winning workers are paid based on performance. We proved that our mechanism satisfies the individual rationality of the honest worker, budget feasibility, truthfulness, and computational efficiency.
更多查看译文
关键词
federated learning,incentive,contribution measurement,reputation,auction-based,ex-post-payment
AI 理解论文
溯源树
样例
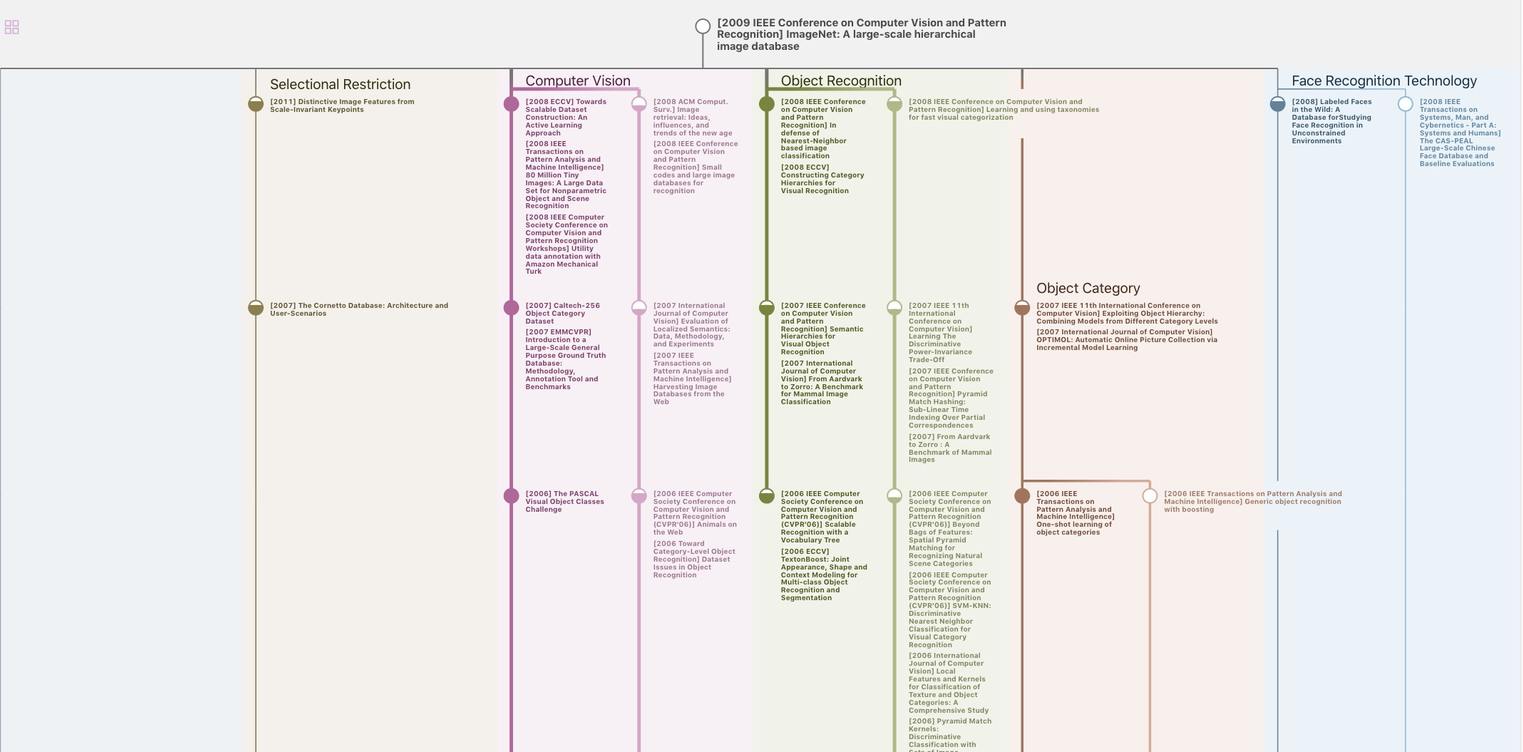
生成溯源树,研究论文发展脉络
Chat Paper
正在生成论文摘要