Computing top-k temporal closeness in temporal networks
Knowledge and Information Systems(2022)
摘要
The closeness centrality of a vertex in a classical static graph is the reciprocal of the sum of the distances to all other vertices. However, networks are often dynamic and change over time. Temporal distances take these dynamics into account. In this work, we consider the harmonic temporal closeness with respect to the shortest duration distance. We introduce an efficient algorithm for computing the exact top- k temporal closeness values and the corresponding vertices. The algorithm can be generalized to the task of computing all closeness values. Furthermore, we derive heuristic modifications that perform well on real-world data sets and drastically reduce the running times. For the case that edge traversal takes an equal amount of time for all edges, we lift two approximation algorithms to the temporal domain. The algorithms approximate the transitive closure of a temporal graph (which is an essential ingredient for the top- k algorithm) and the temporal closeness for all vertices, respectively, with high probability. We experimentally evaluate all our new approaches on real-world data sets and show that they lead to drastically reduced running times while keeping high quality in many cases. Moreover, we demonstrate that the top- k temporal and static closeness vertex sets differ quite largely in the considered temporal networks.
更多查看译文
关键词
Temporal graphs, Temporal closeness, Centrality, Top-k ranking
AI 理解论文
溯源树
样例
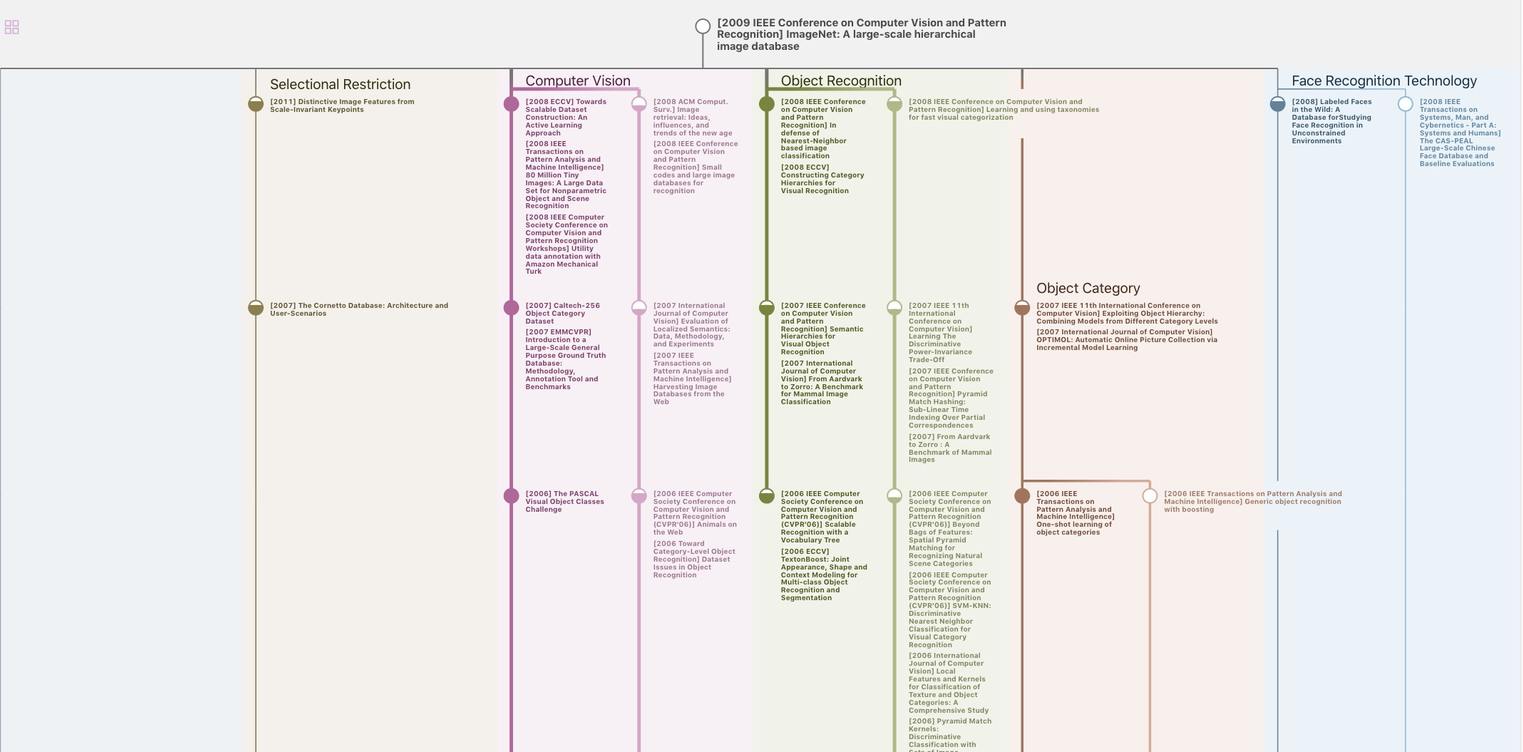
生成溯源树,研究论文发展脉络
Chat Paper
正在生成论文摘要