Inferring CT perfusion parameters and uncertainties using a Bayesian approach
QUANTITATIVE IMAGING IN MEDICINE AND SURGERY(2022)
摘要
Background: Computed tomography perfusion imaging is commonly used for the rapid assessment of patients presenting with symptoms of acute stroke. Maps of perfusion parameters, such as cerebral blood volume (CBV), cerebral blood flow (CBF), and mean transit time (MTI) derived from the perfusion scan data, provide crucial information for stroke diagnosis and treatment decisions. Most CT scanners use singular value decomposition (SVD)-based methods to calculate these parameters. However, some known problems are associated with conventional methods. Methods: In this work, we propose a Bayesian inference algorithm, which can derive both the perfusion parameters and their uncertainties. We apply the variational technique to the inference, which then becomes an expectation-maximization problem. The probability distribution (with Gaussian mean and variance) of each estimated parameter can be obtained, and the coefficient of variation is used to indicate the uncertainty. We perform evaluations using both simulations and patient studies. Results: In a simulation, we show that the proposed method has much less bias than conventional methods. Then, in separate simulations, we apply the proposed method to evaluate the impacts of various scan conditions, i.e., with different frame intervals, truncated measurement, or motion, on the parameter estimate. In one patient study, the method produced CBF and MTT maps indicating an ischemic lesion consistent with the radiologist's report. In a second patient study affected by patient movement, we showed the feasibility of applying the proposed method to motion corrected data. Conclusions: The proposed method can be used to evaluate confidence in parameter estimation and the scan protocol design. More clinical evaluation is required to fully test the proposed method.
更多查看译文
关键词
Stroke, computed tomography perfusion (CT perfusion), Bayesian inference
AI 理解论文
溯源树
样例
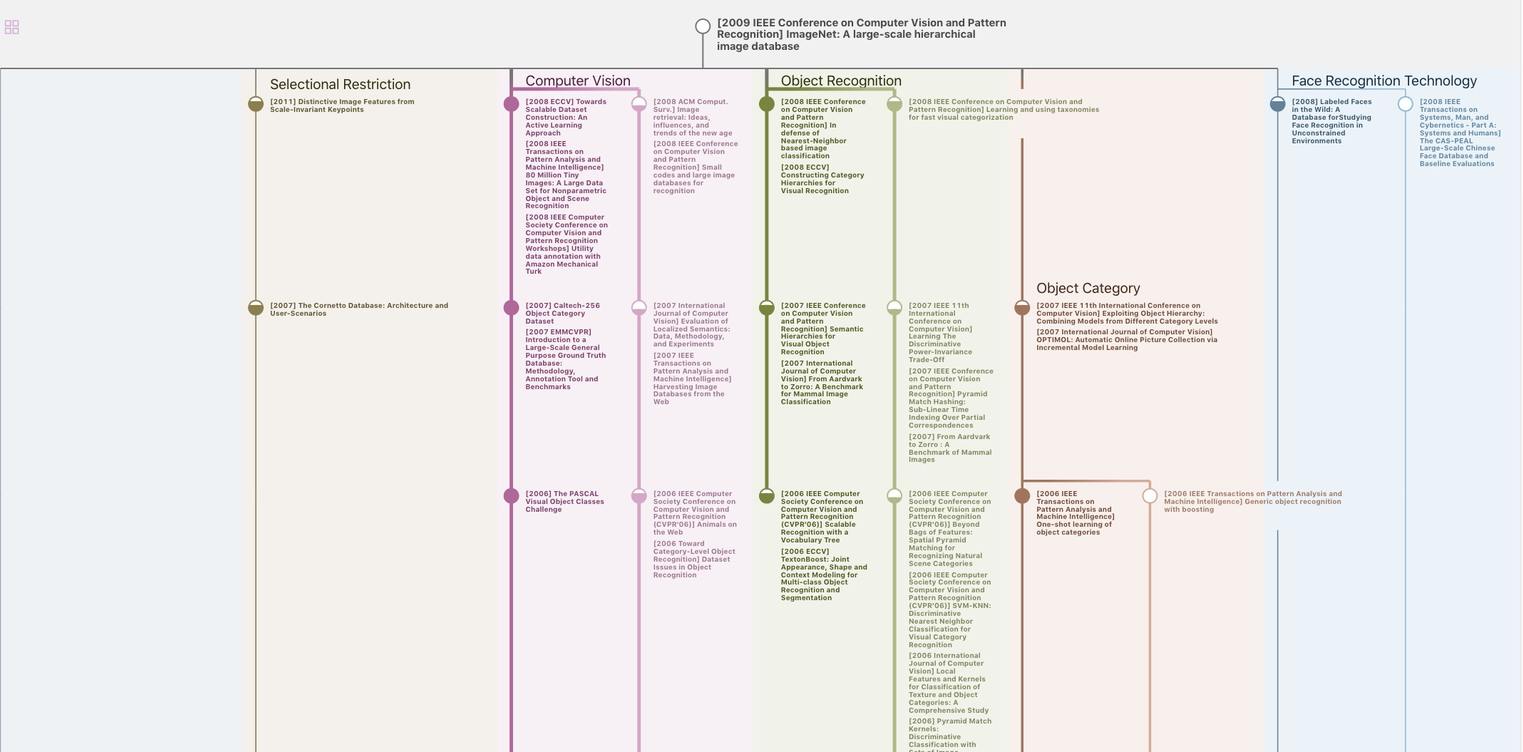
生成溯源树,研究论文发展脉络
Chat Paper
正在生成论文摘要