Extracting Semantic Information from Dynamic Graphs of Geometric Data
COMPLEX NETWORKS & THEIR APPLICATIONS X, VOL 2(2022)
摘要
In this paper, we demonstrate the utility of dynamic network sequences to provide insight into geometric data; moreover, we construct a natural syntactic and semantic understanding of these network sequences for useful downstream applications. As a proof-of-concept, we study the trajectory data of basketball players and construct "interaction networks" to express an essential game mechanic: the ability for the offensive team to pass the ball to each other. These networks give rise to a library of player configurations that can in turn be modeled by a jump Markov model. This model provides a highly compressed representation of a game, while capturing important latent structures. By leveraging this structure, we use a Transformer to predict trajectories with increased accuracy.
更多查看译文
关键词
Geometric data, Networks, Dynamic networks, Machine learning
AI 理解论文
溯源树
样例
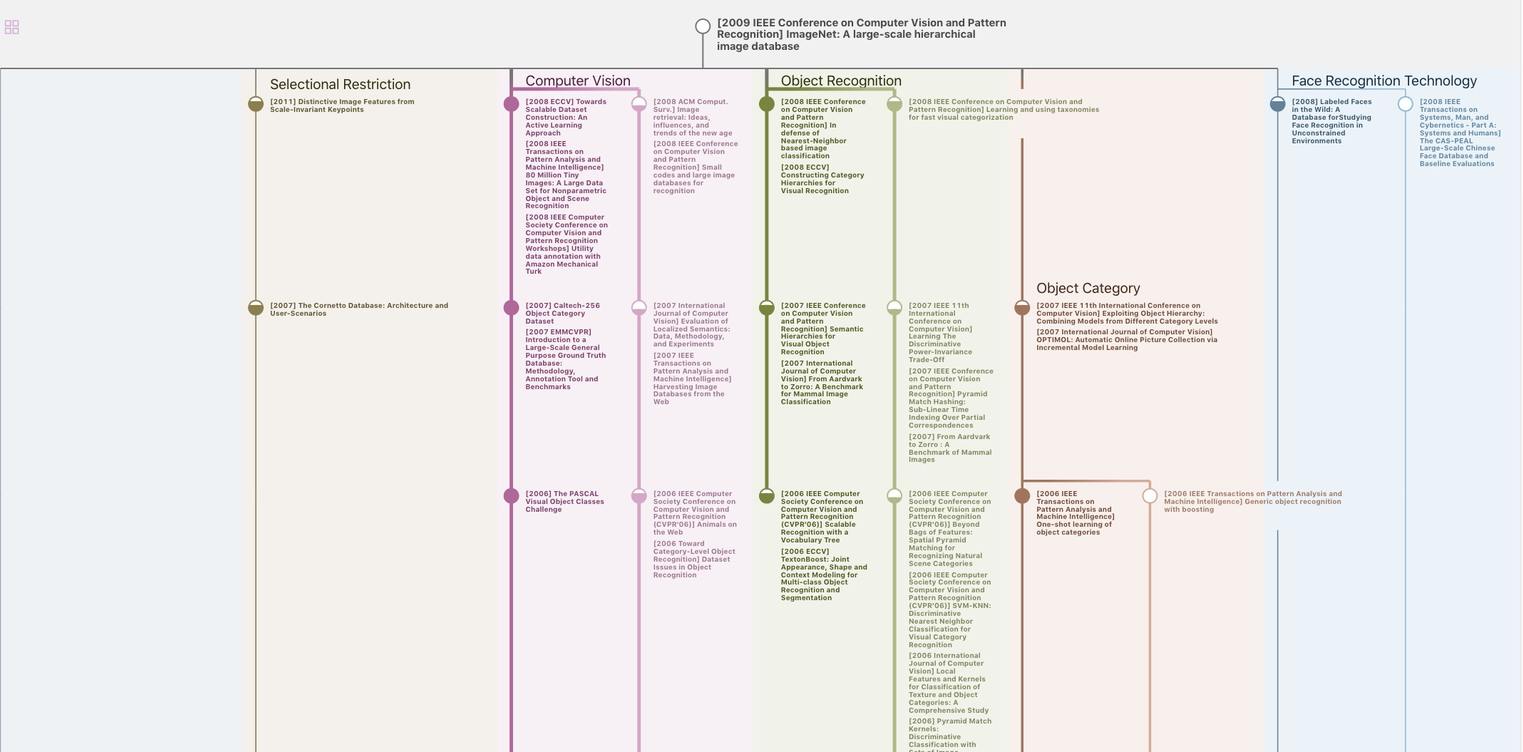
生成溯源树,研究论文发展脉络
Chat Paper
正在生成论文摘要