SEED: A Cross-Layer Semantic Enhanced SLU Model With Role Context Differentiated Fusion
2021 IEEE 33RD INTERNATIONAL CONFERENCE ON TOOLS WITH ARTIFICIAL INTELLIGENCE (ICTAI 2021)(2021)
摘要
The mainstream SLU models, such as SDEN, take the joint training way of slot filling and intent detection because of their correlation and add contextual information to improve the model performance by the contextual vector. Although these models have proved effective, it also brings challenges for slot filling. The slot filling decoder is fed with the deep-layer semantic encoding without alignment information, which will affect the performance of slot filling. The alignment information of the history utterances is attenuated in the context vector because of the repeated fusion process, which is not conducive to the performance improvement of slot filling. In order to solve the above problems, we proposed a novel cross layer semantic enhanced SLU model with role context differentiated fusion, which contains two important improvements: 1) the word embedding information of the current utterance is introduced into the slot filling decoder to strengthen the alignment information based on the mutual attention mechanism; 2) the utterances of different roles are fused in different ways to reserve the alignment information of history utterances in the contextual vector. A large number of experiments were carried out on the standard dataset from SDEN, named KVRET*, and the results verify the effectiveness of our new model. Our model can increase the F1 score of slot filling by more than 7.5% than the existing models.
更多查看译文
关键词
Spoken Language Understanding, Role Context, Differentiated Fusion
AI 理解论文
溯源树
样例
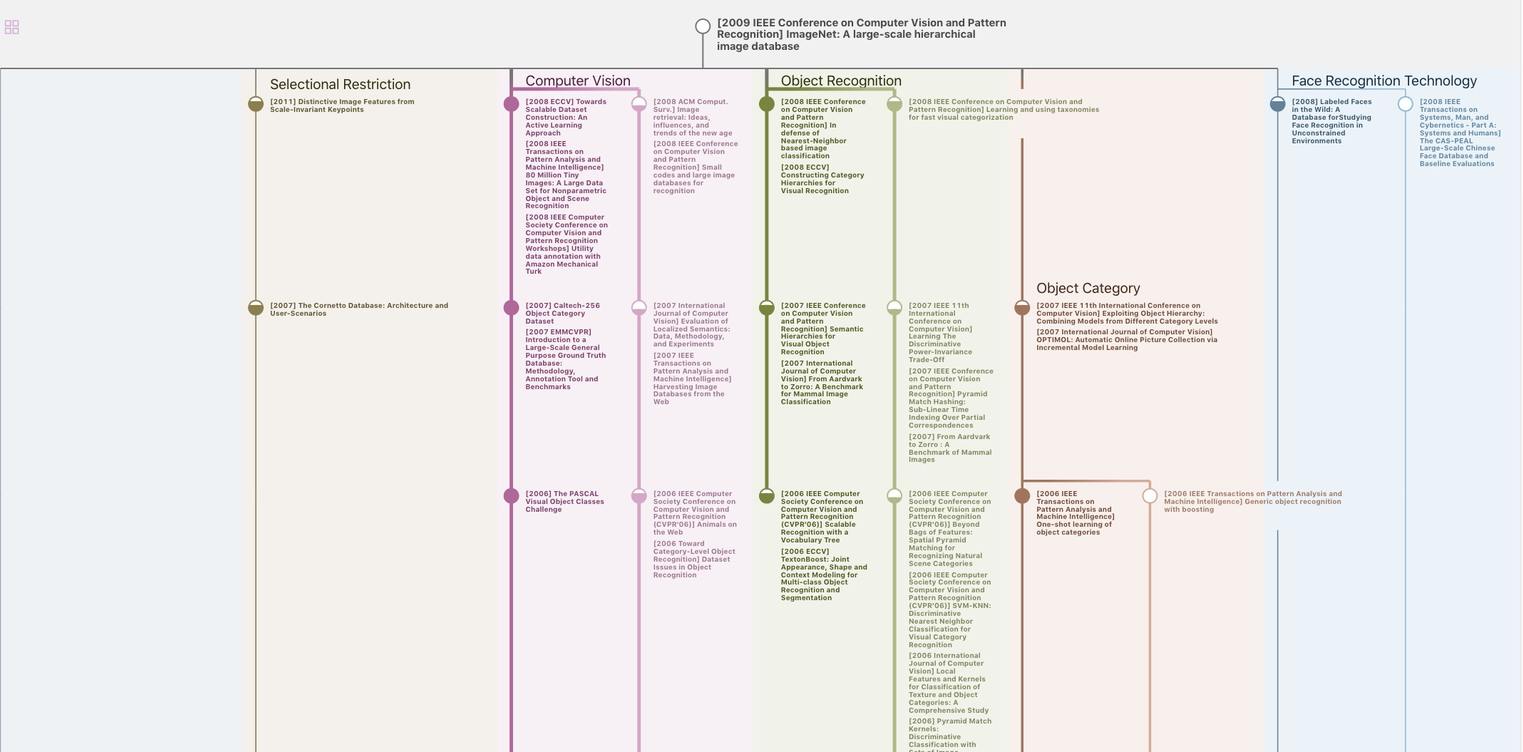
生成溯源树,研究论文发展脉络
Chat Paper
正在生成论文摘要