Optimization of the Diffusion Time in Graph Diffused-Wasserstein Distances: Application to Domain Adaptation
2021 IEEE 33RD INTERNATIONAL CONFERENCE ON TOOLS WITH ARTIFICIAL INTELLIGENCE (ICTAI 2021)(2021)
摘要
The use of the heat kernel on graphs has recently given rise to a family of so-called Diffusion-Wasserstein distances which resort to Optimal Transport theory for comparing attributed graphs. In this paper, we address the open problem of optimizing the diffusion time used in these distances. Inspired from the notion of triplet-based constraints, we design a loss function that aims at bringing two graphs closer together while keeping an impostor away. After a thorough analysis of the properties of this function, we show on synthetic data that the resulting Diffusion-Wasserstein distances outperforms the Gromov and Fused-Gromov Wasserstein distances on unsupervised graph domain adaptation tasks.
更多查看译文
关键词
Graphs, optimal transport, domain adaptation
AI 理解论文
溯源树
样例
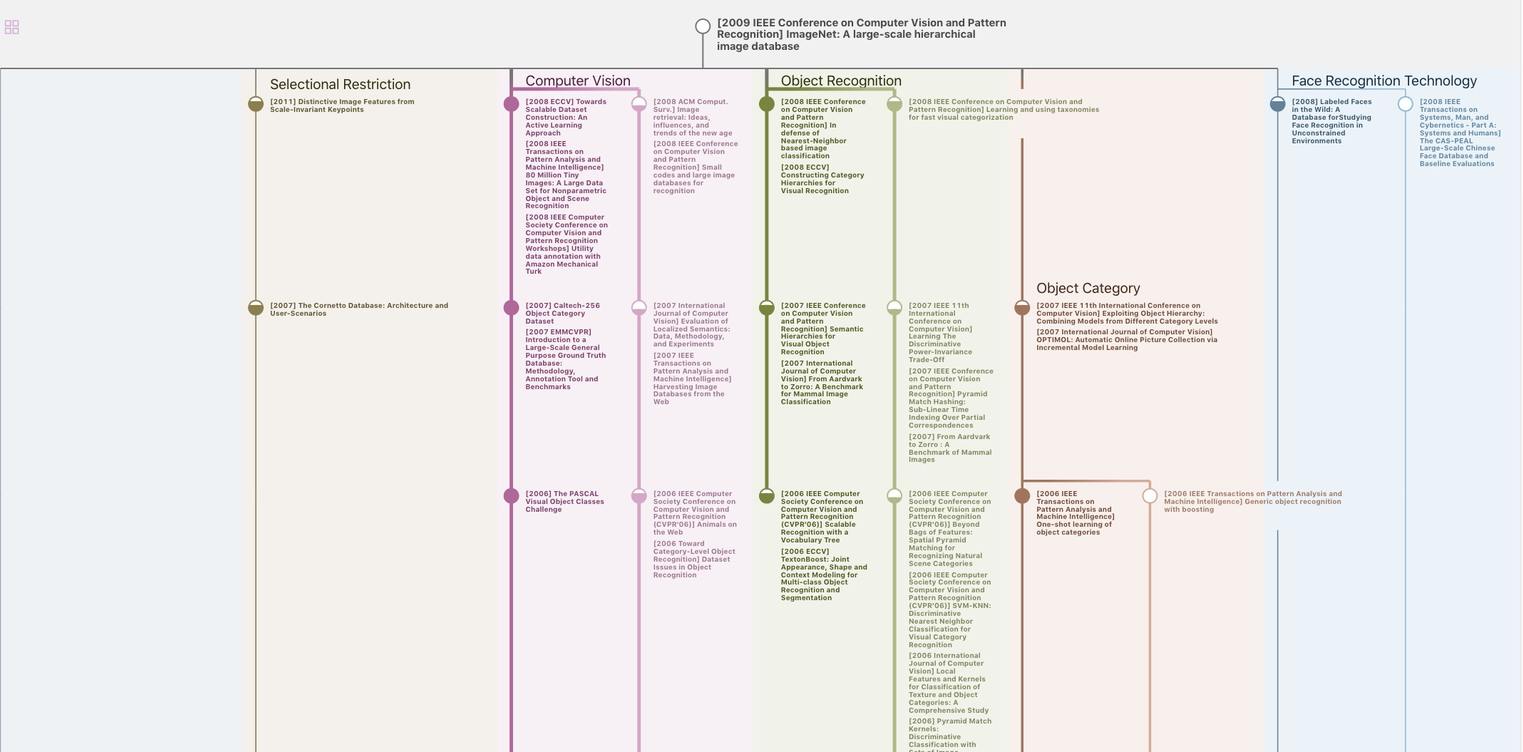
生成溯源树,研究论文发展脉络
Chat Paper
正在生成论文摘要