Overlapped Speech Detection and speaker counting using distant
COMPUTER SPEECH AND LANGUAGE(2022)
摘要
We study the problem of detecting and counting simultaneous, overlapping speakers in a multichannel, distant-microphone scenario. Focusing on a supervised learning approach, we treat Voice Activity Detection (VAD), Overlapped Speech Detection (OSD), joint VAD and OSD (VAD+OSD) and speaker counting in a unified way, as instances of a general Overlapped Speech Detection and Counting (OSDC) multi-class supervised learning problem. We consider a Temporal Convolutional Network (TCN) and a Transformer based architecture for this task, and compare them with previously proposed state-of-the art methods based on Recurrent Neural Networks (RNN) or hybrid Convolutional-Recurrent Neural Networks (CRNN). In addition, we propose ways of exploiting multichannel input by means of early or late fusion of single-channel features with spatial features extracted from one or more microphone pairs. We conduct an extensive experimental evaluation on the AMI and CHiME-6 datasets and on a purposely made multichannel synthetic dataset. We show that the Transformer-based architecture performs best among all architectures and that neural network based spatial localization features outperform signal-based spatial features and significantly improve performance compared to single-channel features only. Finally, we find that training with a speaker counting objective improves OSD compared to training with a VAD+OSD objective.
更多查看译文
关键词
Voice activity detection, Overlapped Speech Detection, Speaker counting, Distant microphones, Spatial features
AI 理解论文
溯源树
样例
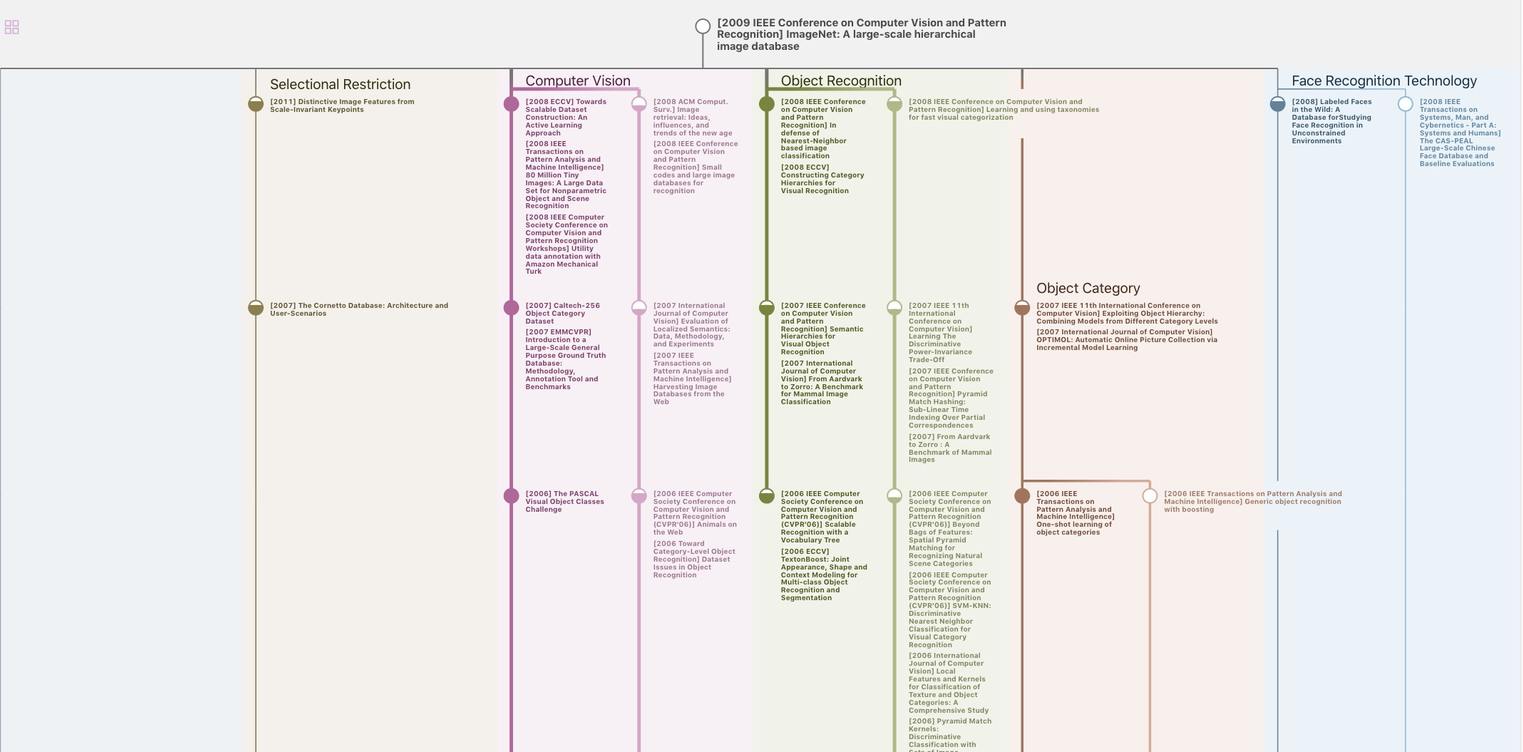
生成溯源树,研究论文发展脉络
Chat Paper
正在生成论文摘要