Precipitate-Supported Thermal Proteome Profiling Coupled with Deep Learning for Comprehensive Screening of Drug Target Proteins
ACS CHEMICAL BIOLOGY(2022)
摘要
Although thermal proteome profiling (TPP) acts as a popular modification-free approach for drug target deconvolution, some key problems are still limiting screening sensitivity. In the prevailing TPP workflow, only the soluble fractions are analyzed after thermal treatment, while the precipitate fractions that also contain abundant information of drug-induced stability shifts are discarded; the sigmoid melting curve fitting strategy used for data processing suffers from discriminations for a part of human proteome with multiple transitions. In this study, a precipitatesupported TPP (PSTPP) assay was presented for unbiased and comprehensive analysis of protein-drug interactions at the proteome level. In PSTPP, only these temperatures where significant precipitation is observed were applied to induce protein denaturation and the complementary information contained in both supernatant fractions and precipitate fractions was used to improve the screening specificity and sensitivity. In addition, a novel image recognition algorithm based on deep learning was developed to recognize the target proteins, which circumvented the problems that exist in the sigmoid curve fitting strategy. PSTPP assay was validated by identifying the known targets of methotrexate, raltitrexed, and SNS-032 with good performance. Using a promiscuous kinase inhibitor, staurosporine, we delineated 99 kinase targets with a specificity up to 83% in K562 cell lysates, which represented a significant improvement over the existing thermal shift methods. Furthermore, the PSTPP strategy was successfully applied to analyze the binding targets of rapamycin, identifying the wellknown targets, FKBP1A, as well as revealing a few other potential targets.
更多查看译文
关键词
thermal proteome profiling,proteins,deep learning,precipitate-supported
AI 理解论文
溯源树
样例
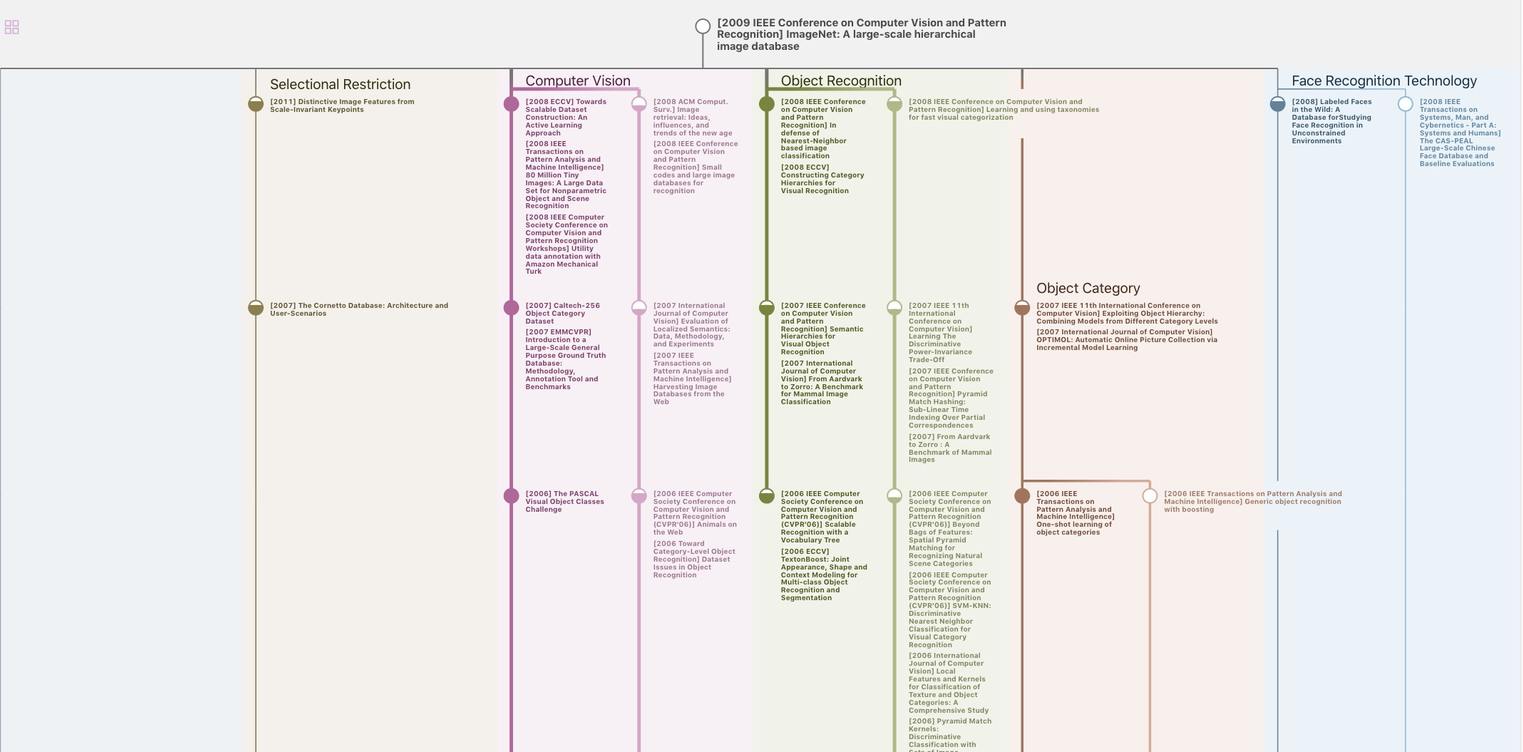
生成溯源树,研究论文发展脉络
Chat Paper
正在生成论文摘要