Crop Agnostic Monitoring Driven by Deep Learning
FRONTIERS IN PLANT SCIENCE(2021)
摘要
Farmers require diverse and complex information to make agronomical decisions about crop management including intervention tasks. Generally, this information is gathered by farmers traversing their fields or glasshouses which is often a time consuming and potentially expensive process. In recent years, robotic platforms have gained significant traction due to advances in artificial intelligence. However, these platforms are usually tied to one setting (such as arable farmland), or algorithms are designed for a single platform. This creates a significant gap between available technology and farmer requirements. We propose a novel field agnostic monitoring technique that is able to operate on two different robots, in arable farmland or a glasshouse (horticultural setting). Instance segmentation forms the backbone of this approach from which object location and class, object area, and yield information can be obtained. In arable farmland, our segmentation network is able to estimate crop and weed at a species level and in a glasshouse we are able to estimate the sweet pepper and their ripeness. For yield information, we introduce a novel matching criterion that removes the pixel-wise constraints of previous versions. This approach is able to accurately estimate the number of fruit (sweet pepper) in a glasshouse with a normalized absolute error of 4.7% and an R-2 of 0.901 with the visual ground truth. When applied to cluttered arable farmland scenes it improves on the prior approach by 50%. Finally, a qualitative analysis shows the validity of this agnostic monitoring algorithm by supplying decision enabling information to the farmer such as the impact of a low level weeding intervention scheme.
更多查看译文
关键词
plant classification, artificial intelligence, deep learning, convolutional neural network, image segmentation, field plant observation
AI 理解论文
溯源树
样例
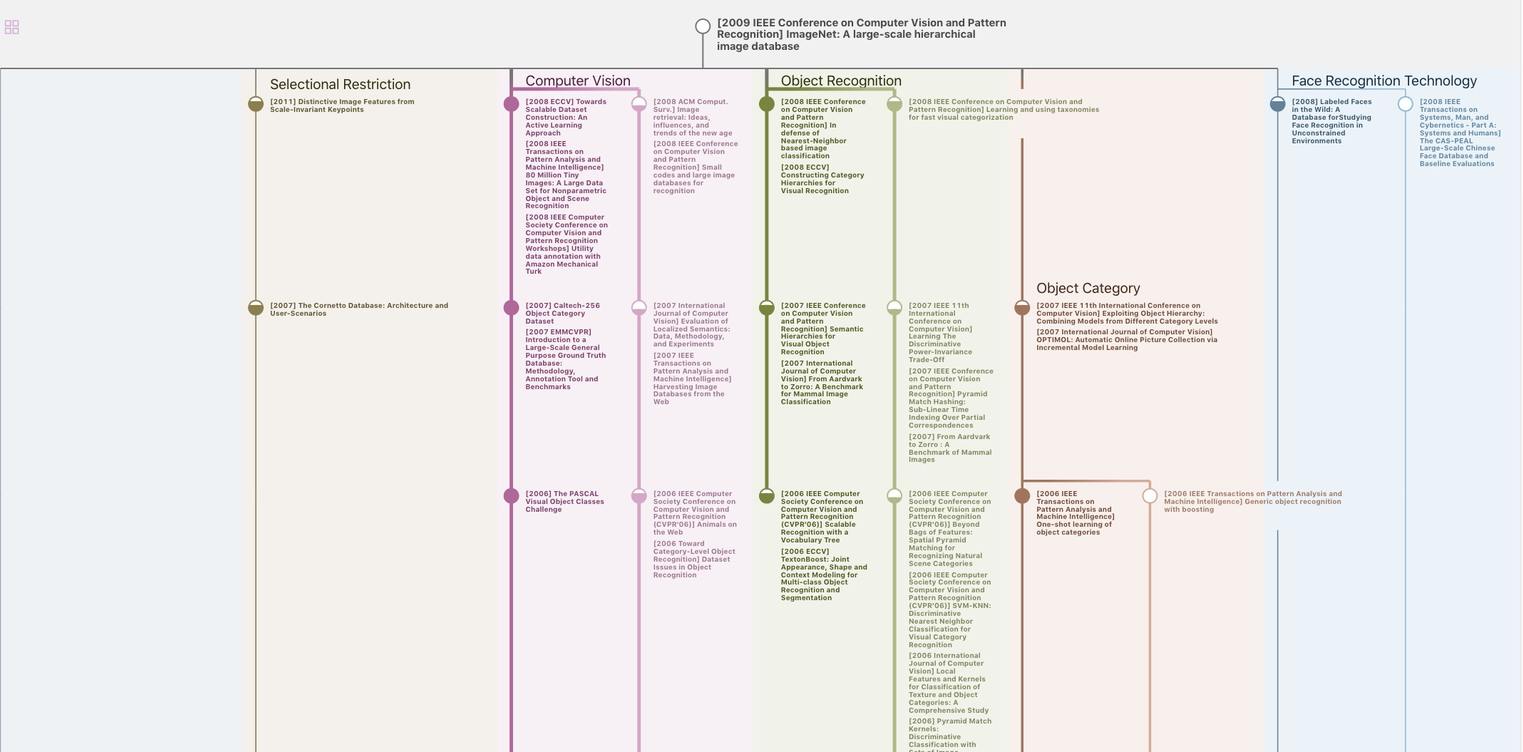
生成溯源树,研究论文发展脉络
Chat Paper
正在生成论文摘要