Random Compressed Coding with Neurons
biorxiv(2022)
摘要
Classical models of efficient coding in neurons assume simple mean responses--'tuning curves'--such as bell shaped or monotonic functions of a stimulus feature. Real neurons, however, can be more complex: grid cells, for example, exhibit periodic responses which impart the neural population code with high accuracy. But do highly accurate codes require fine tuning of the response properties? We address this question with the use of a benchmark model: a neural network with random synaptic weights which result in output cells with irregular tuning curves. Irregularity enhances the local resolution of the code but gives rise to catastrophic, global errors. For optimal smoothness of the tuning curves, when local and global errors balance out, the neural network compresses information from a high-dimensional representation to a low-dimensional one, and the resulting distributed code achieves exponential accuracy. An analysis of recordings from monkey motor cortex points to such 'compressed efficient coding'. Efficient codes do not require a finely tuned design--they emerge robustly from irregularity or randomness.
### Competing Interest Statement
The authors have declared no competing interest.
更多查看译文
关键词
neurons
AI 理解论文
溯源树
样例
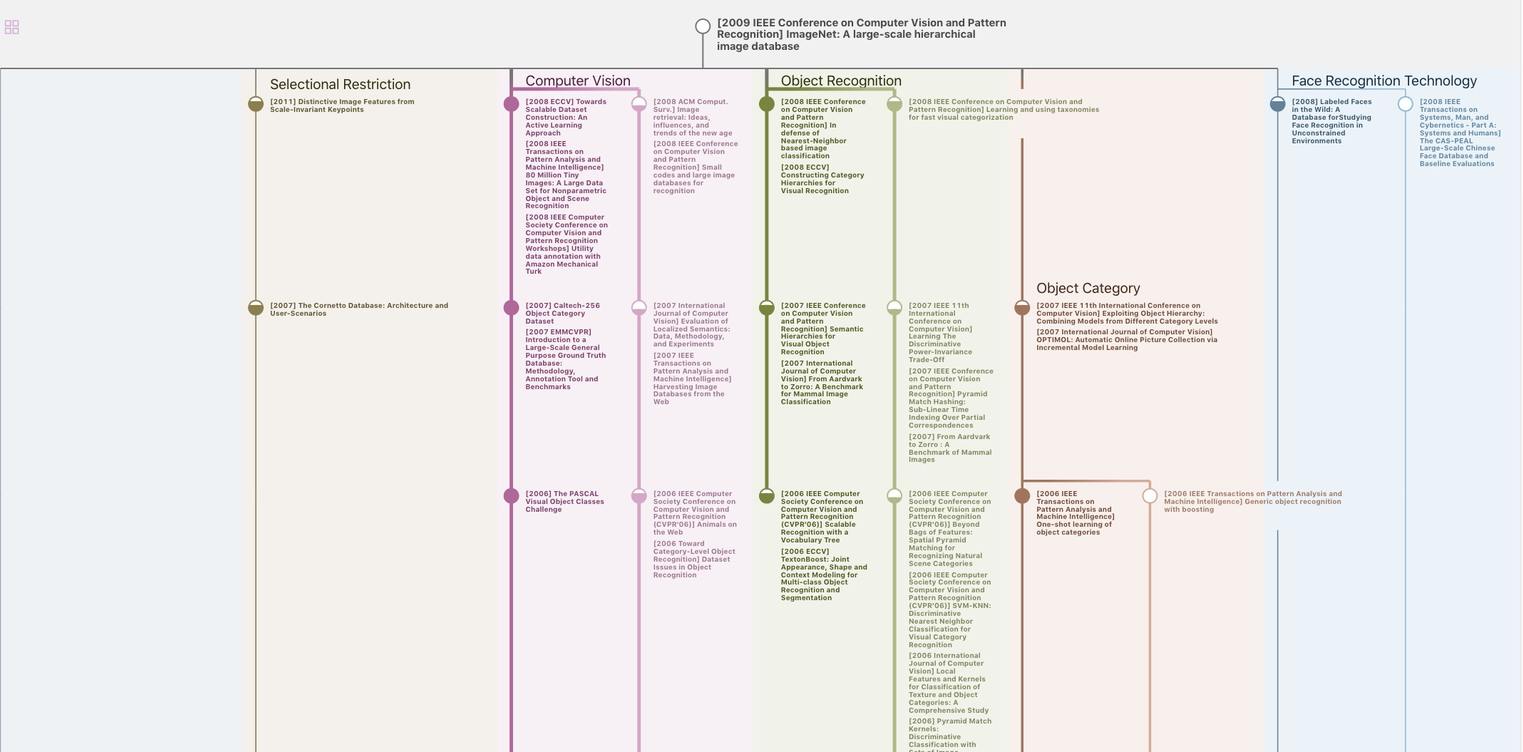
生成溯源树,研究论文发展脉络
Chat Paper
正在生成论文摘要