Sablas: Learning Safe Control for Black-Box Dynamical Systems
IEEE ROBOTICS AND AUTOMATION LETTERS(2022)
摘要
Control certificates based on barrier functions have been a powerful tool to generate probably safe control policies for dynamical systems. However, existing methods based on barrier certificates are normally for white-box systems with differentiable dynamics, which makes them inapplicable to many practical applications where the system is a black-box and cannot be accurately modeled. On the other side, model-free reinforcement learning (RL) methods for black-box systems suffer from lack of safety guarantees and low sampling efficiency. In this paper, we propose a novel method that can learn safe control policies and barrier certificates for black-box dynamical systems, without requiring for an accurate system model. Our method re-designs the loss function to back-propagate gradient to the control policy even when the black-box dynamical system is non-differentiable, and we show that the safety certificates hold on the black-box system. Empirical results in simulation show that our method can significantly improve the performance of the learned policies by achieving nearly 100% safety and goal reaching rates using much fewer training samples, compared to state-of-the-art black-box safe control methods. Our learned agents can also generalize to unseen scenarios while keeping the original performance. The source code can be found at https://github.com/ Zengyi-Qin/bcbf.
更多查看译文
关键词
Robust/adaptive control, robot safety
AI 理解论文
溯源树
样例
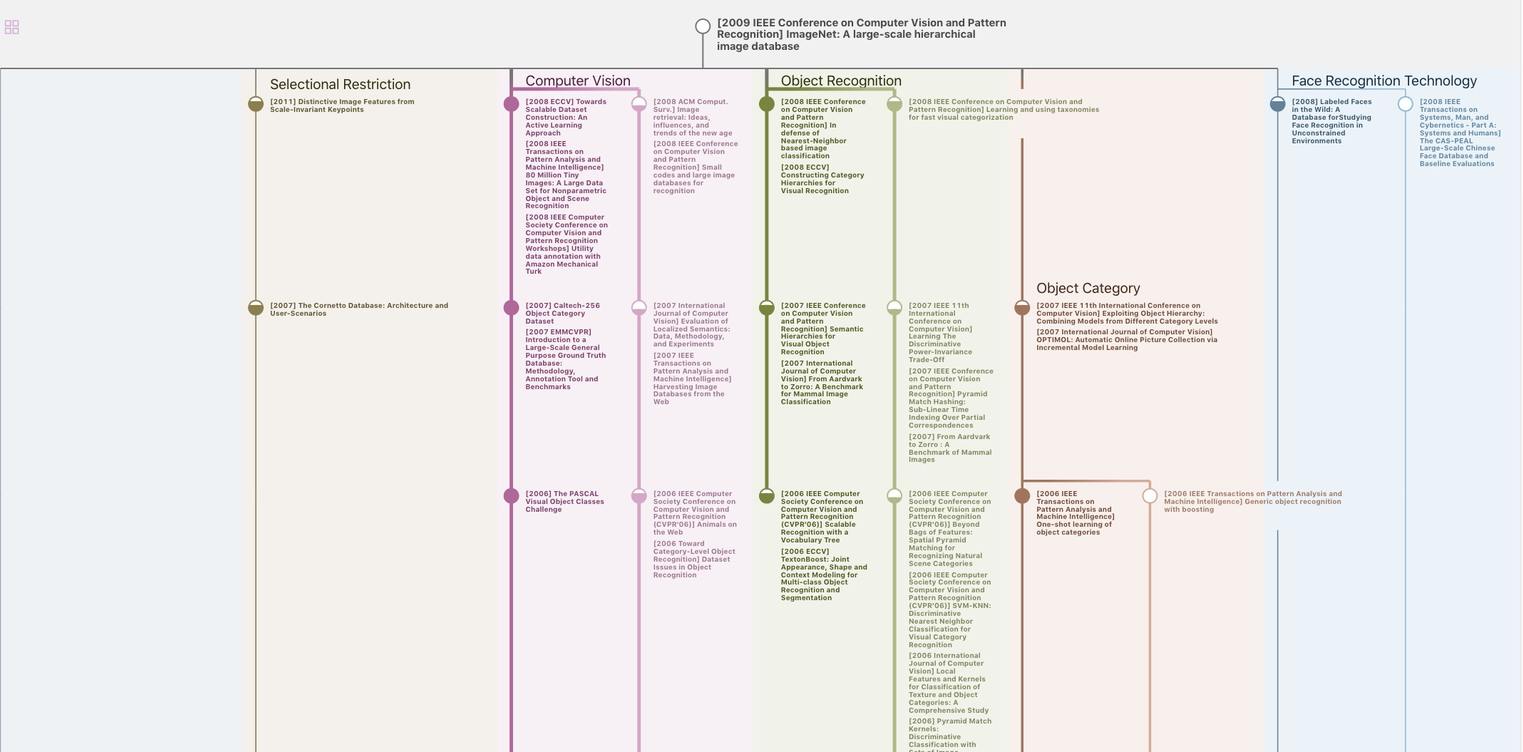
生成溯源树,研究论文发展脉络
Chat Paper
正在生成论文摘要